Long-run outcomes: Measuring program effectiveness over time
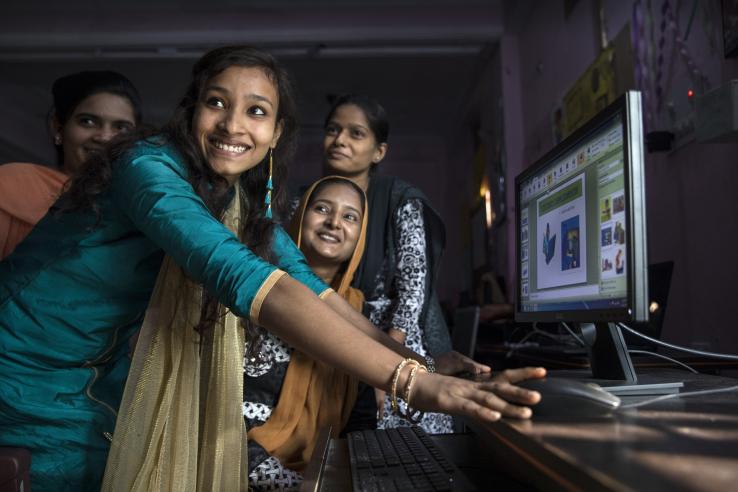
Over the past 20 years, J-PAL affiliated researchers have conducted policy relevant research through over one thousand randomized evaluations. Like those from randomized controlled trials (RCTs) more broadly, the vast majority of estimates in these interventions focus on short- and medium-term impacts ranging from a few months to a few years after program implementation.
Only in recent years has it become possible to assess program impact a decade or more post-intervention. Studies that have begun to do so show how the persistence of treatment effects over long time horizons can be difficult to predict. For example, programs with a focus on building human capital seem to have more persistent effects, while those centered around short-term cash transfers appear to have a strong initial effect that then wanes over time. Understanding changes in the magnitude of effects over extended time horizons can reframe how we think about program efficacy, which can in turn have important implications for experimental design, cost-effectiveness analysis, and policy decisions.
With the rise of big data, more advanced data gathering applications, and increased mobile phone usage worldwide, there is a growing opportunity to utilize new data sources for long-run tracking and respondent follow-up to capture long-term effects. This work is not without challenges, however. Studies may not have been designed to allow for long-run follow-up and analysis, and in many cases, participants’ contact information is either outdated or no longer available. Furthermore, challenges around participant tracking, attrition, contamination from other programs, and adequate funding make it difficult to collect the data necessary to observe long-term impacts.
In this blog post, we draw on the experience of J-PAL research staff and J-PAL funded projects to discuss some of the opportunities and challenges associated with measuring long term impacts in greater depth. It is the first in a series highlighting lessons learned from J-PAL’s 20 years of conducting randomized evaluations, with attention to exciting developments driving research towards the future.
What are long-run outcomes, and why are we interested in measuring them?
Though there is no set definition of what constitutes "long-run," we follow Bougen et al. 2019 and consider long-term outcomes to be those observed roughly ten or more years post-intervention. This timeframe allows researchers to observe treatment effects on participants’ long-term living standards and life trajectories. Measuring the persistence of effects over this period is particularly useful for several reasons.
One reason is that strong short-run effects can fade over time due to lack of persistence, among other factors. The persistence of treatment effects reflect the extent that behavior change in program participants continues after the end of an intervention. Low persistence rates could lead to an eventual convergence in key outcomes between treatment and control groups.
Alternatively, weak short-run effects may strengthen over time depending on the nature of the intervention. Programs such as those relating to early childhood development or other human capital and social investments may take time to produce impacts but have the potential to persist and grow over extended periods.
Further reason to measure long-term outcomes is that studies with short-run effects shown to weaken in the medium-run can have impacts that resurface in the long-run. This fluctuation may result from inadequate capture of relative skills that could interact with and improve long-run outcomes (e.g. connection to social networks or pre-existing aptitudes) in earlier rounds of data collection. Assuming there would be no future impact based on small or declining medium-term results could lead to incorrect conclusions about an intervention’s true effectiveness.
Lastly, the persistence of effects over time could have implications for the overall cost effectiveness of an intervention. Effects that persist or strengthen over time can increase an intervention’s rate of return and improve overall cost effectiveness; see Attanasio et al. (2017) for an example. This can inform policymakers and practitioners on where to best allocate limited resources.
Emerging insights from studying long-run outcomes
Findings from studies with long-run follow-ups are beginning to uncover important insights into outcome trajectories for specific types of interventions. These assessments at times reveal unexpected effect patterns for commonly implemented programs. A few of the studies assessed so far include evaluations of cash transfer programs, child health interventions, integrated training programs, and education-focused interventions. In this section, we briefly highlight findings from a few studies, including those conducted by J-PAL affiliated researchers; for a more exhaustive overview of studies examining long-term impacts, see Bouguen et al. (2019).
Long-run analysis of various cash transfer programs (conditional and unconditional cash transfers, lump-sum grants, and scholarships) show stark differences in outcomes between programs which designate funds used for human capital building—in particular for children—versus those that do not. For simple unconditional cash transfers and entrepreneurial grants, studies show that gains largely fade once the value of transfers have been depleted. However, scholarships and conditional cash transfers contingent on school attendance show potential long-term gains for children in treatment households, with some evidence that savings components may add to this effect. There is also evidence supporting positive long-run effects for cash transfer programs when coupled with complementary productive measures such as technical skills training and financial advising.
Other findings from studies on early childhood health interventions show positive effects on educational advancement and economic productivity in the long-run. An evaluation of an early childhood cognitive stimulation program shows significant gains in educational attainment and achievement twenty years post-intervention, complementing similar findings from early childhood nutritional supplementation programs.
Finally, long-run results from some studies are beginning to reveal differential effects between treated groups, most notably along gender lines. Although some studies report larger effects for women than men in terms of learning and educational attainment more than a decade post-intervention, others show the inverse, while still other studies show both men and women benefiting from the intervention, but in different ways.
Ensuring successful long-run studies
Challenges associated with tracking outcomes over a decade or more include participant tracking and associated costs, possible contamination with other programs, and more. As such,
designing a study from the beginning to measure long-run effects can help set it up for success. Design aspects to consider include those around informed consent, sampling, randomization strategy, program implementation, and data collection.
Informed consent, Institutional Review Board (IRB) approvals, and Data Use Agreements (DUAs): The consent script should include language on whether the participant consents to being re-contacted for follow-up surveys. Additionally, new IRB approval will be required in almost all cases, and all members of the long-term follow-up study team should be listed on the IRB protocol. Participants' identifiable information should not be shared with members of the follow-up study team (if different from the original study team) unless the participants have consented to being re-contacted and an IRB has approved the new protocol. If the original study relied on administrative data, a new data use agreement (DUA) will typically be required, and identified data should not be shared without written permission from the data provider in addition to the IRB approvals and consent/waiver of consent requisites described above.
Sampling: From the start, a study must have sufficient statistical power to measure long-run impacts. This may involve oversampling to account for possible attrition (see J-PAL’s resource on power calculations for more). Some studies investigating long-term impacts have invested heavily in participant tracking and achieve very low attrition rates (to the order of 83 percent or even 94 percent of the original sample reached over a decade later). Others have higher attrition rates, more in the range of 40 percent. Thus, realistic estimates of attrition and the ability to invest in long-term tracking are critical.
Randomization strategy: Phase-in designs present challenges in measuring long-term impacts, as the control group ultimately receives the intervention. Thus, the longer the lag between each phase, the easier it will be to capture long-term differences between groups. Bouguen et al. (2019) additionally show that it is possible under certain assumptions to estimate long-term differences using phase-in designs, provided outcomes are measured prior to the control group receiving the program and are measured sufficiently frequently thereafter.
Maintaining integrity of the study design: Just as in examining short- and medium-term effects, maintaining the integrity of the study design throughout the duration of the study is essential to measuring valid and reliable outcomes. Beyond sample size considerations, attrition that differs between the treatment and control groups (e.g., if recipients of a vocational training program are more likely to migrate out of the study location to seek work elsewhere) presents a challenge to measuring long-term impact. Spillovers can present another challenge in a similar fashion as with evaluations focusing on short- or medium-term effects: skills from a job training program may filter out to untreated workers, successful businesses started through an entrepreneurial grant may affect others in the area, or health interventions may affect other children in the vicinity. Another consideration is if competing programs or policies implemented in the study area are offered to a subset of the population. It then becomes important to understand who has access to those other programs and to account for possible effects in analysis.
Data sources: The rise of administrative data in randomized evaluations presents an opportunity to track outcomes over an extended period. When available—that is, when the data contains relevant indicators and can be matched to study participants—administrative data can be considerably cheaper than participant tracking and a series of follow-up surveys as well as provide better coverage of study participants. For example, Barrera-Osorio et al. (2019) use administrative data on school enrollment and completion to examine the long-term impacts of three Colombian conditional cash transfer programs, while Attanasio et al. (2017) use social and labor market administrative records to study a vocational training program, also in Colombia.
When administrative data is not available or needs to be augmented by survey data (e.g. to follow up with participants who have left the area covered by the administrative data, such as those who have left the country), it is important to collect comprehensive contact information from respondents during data collection. This should include phone numbers, WhatsApp numbers (which are less likely to change over time), and phone numbers of close relatives or neighbors—and verifying them at the time of data collection and periodically between follow-up surveys, ideally at least every few years (Bouguen et al. 2019).
Opportunities for building in long-run follow-up for RCTs
The rise of big data and other advanced information-gathering applications as well as increased mobile phone penetration in low- and middle- income countries (LMICs) can enable new methods of long-run participant tracking and outcome assessments. Big data sources such as satellite and mobile phone records can facilitate the use of predictive learning models to estimate household wealth, electrification, and certain other long-run outcomes of interest. With increased use of these non-traditional sources, data quality checks similar to those done on survey data are being developed to ensure the integrity of data being used for analysis.
Increased mobile phone penetration in developing regions can also facilitate long-term participant tracking and survey administration. Advancements in Computer Assisted Telephone Interviewing (CATI) methods make remote surveying a cost-effective option for collecting reliable data (see J-PAL’s resource on conducting remote surveys for more) and may be particularly helpful in tracking highly mobile study participants, such as migrant workers or those belonging to displaced populations. With certain samples, as participants age and settle into more predictable work life routines, it also becomes easier to track them for follow-up.
Longitudinal datasets such as the Kenya Life Panel Survey, others currently being administered, or even census activities planned by the government can provide the opportunity for researchers to plan experiments around existing study samples and data collection rounds. Another opportunity to take advantage of planned longitudinal data collection efforts comes from government census activities. These types of pre-planned efforts may also help relieve some of the burdens of long-term follow-up for researchers and reduce sample attrition.
As these various methods become increasingly accessible, there is great potential to learn more about long-term impacts and cost-effectiveness of programs implemented a decade or more ago. In turn, these learnings can help further inform evidence-based policymaking and future program design.