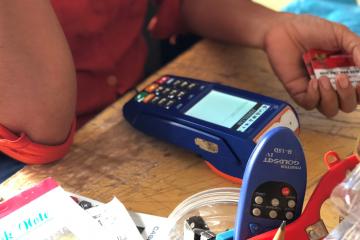
Using alternative data and artificial intelligence to expand financial inclusion: Evidence-based insights
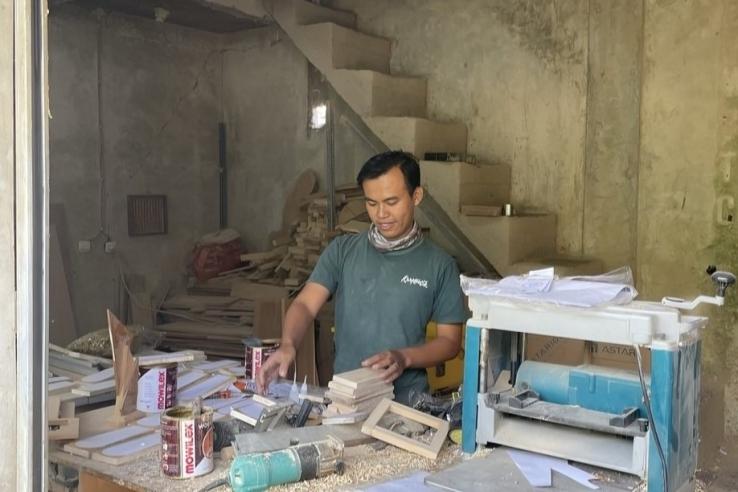
Photo: Nurul Fadilah | J-PAL
In Indonesia, many micro, small and medium enterprises (MSMEs) face challenges in accessing credit to grow their business, with only 12.7 percent of MSMEs borrowing from banks and other sources in 2021. These constraints are partially due to MSMEs' lack of collateral or historical credit information, leading lenders to view them as high risk or charge high interest rates.
In response, firms using innovative credit-scoring (ICS) have emerged to help banks and peer-to-peer lenders predict potential borrowers’ likelihood to repay a loan. In contrast to traditional credit scoring models that use credit history, ICS uses artificial intelligence (AI), specifically machine learning algorithms, to evaluate data unrelated to a borrower’s credit history—what we refer to here as “alternative data”—to make predictions. These data could be digital transaction data, mobile phone and social media usage, or bills and tax payment history.
Machine learning algorithms analyze large sets of the alternative data to identify patterns that can be useful in making credit risk assessments, which traditional models may overlook. For example, research shows that the presence of a financial app on an individual’s phone can be an indicator of financial sophistication and therefore, a useful variable for predicting credit worthiness. The accuracy of these algorithms increases over time as new historical data are added to the model.
Adoption of ICS is a promising approach to increase credit access, especially for those who are unbanked or lack collateral. The Government of Indonesia has announced that they are exploring implementing a credit scoring system that uses alternative data to help more MSMEs gain access to lending. However, several questions remain: How can ICS effectively assist governments and lenders in increasing credit access? How accurate is the information generated by ICS, considering the diverse demographic of Indonesia? Are there risks associated with using ICS?
These were some of the key questions explored during the recent “Learning Collaborative: Innovation for MSME Development” event hosted by J-PAL Southeast Asia and the Directorate of MSME and Cooperative Development of Indonesia’s Ministry of National Development Planning (Bappenas) on 11 December 2023. During the session, representatives from government, the private sector, academia, and non-governmental organizations discussed the opportunities and challenges of using alternative data and AI in credit scoring by drawing from existing research and their experience.
Photo: J-PAL
Opportunities and challenges of using AI and alternative data
Alternative data, such as mobile and social footprints, may enhance traditional credit scoring models and improve financial inclusion. A study in India by Agarwal et al. (2019) found that machine learning models using mobile and social footprint variables (e.g. types of apps installed, presence of social apps) can predict loan defaults more effectively than models that only use credit scores as they capture unique insights into credit risk that are not easily captured by earnings, education, or credit scores. The study also suggested that using alternative data can improve credit access for individuals without a credit score who would have been rejected by the traditional credit screening model.
While alternative data may be used to predict loan default, participants at the Learning Collaborative event raised the challenge of data integration and transfer that may affect the performance of ICS, which highly depends on the quality and quantity of data. Currently, acquiring high-quality alternative data remains a challenge in Indonesia, due to hesitancy from data owners to share information, a lack of understanding of the methods to share data safely (i.e. protecting personal information), and the absence of a standardized data collection protocol among firms.
At the moment, the government of Indonesia is creating an integrated MSME database that could facilitate data standardization and sharing and has developed the Personal Data Protection Law and AI code of ethics to govern safe AI use. Moving forward, participants also highlighted the importance of strengthening the regulatory landscape to enhance data management practices. Aligned with these concerns, the Financial Services Authority of Indonesia (OJK) recently issued OJK Regulation No.3/2024 to support financial technology innovations, whilst ensuring consumer and data protection.
Machine learning algorithms may be able to work alongside human agents and improve credit assessments. A study by Bryan et al. (2023) found that loan officers may not accurately assess potential clients, leading to suboptimal lending choices. In the study, loan officers incorrectly perceived clients predicted by machine learning as top performers to be more likely to default than those predicted as low performers. These studies suggest that loan officers may benefit from better identification methods, such as machine learning to make better credit decisions.
However, while machine learning algorithms may be used to measure creditworthiness, there are biased risks associated with it. A study in Pakistan by Kisat (2021) revealed that including gender in machine learning’s dataset led to the algorithm selecting fewer women and thus, suggested that not revealing gender information may boost more equitable credit allocation. Similarly, a report by the Center of Indonesian Policy (CIPS) also cautions against biased risks.
In Indonesia, biases may arise due to women’s underrepresentation in training datasets, as men are more likely to own smartphones and have household bills associated with their names. Therefore, it emphasizes that ICS firms must ensure that gender does not affect credit scores.
Research questions to move forward
Despite the increasing interest in ICS methods, there is limited research on AI’s application in MSME credit evaluations, especially in Indonesia. Further research can help guide ICS firms in developing algorithms that can boost financial access for MSMEs and minimize biases. It can also assist governments in creating policies and regulations that can facilitate credit allocations safely, efficiently and equitably.
Areas for further research may include:
Mapping out alternative data for credit risk assessment
- Which types of alternative data are most effective for assessing the creditworthiness of entrepreneurs with varying socioeconomic backgrounds?
Evaluating the implementation, performance, and cost-effectiveness of ICS
- How can financial service providers effectively integrate the assessment of ICS with those made by loan officers?
- How does the predictive accuracy of machine learning compare to that of loan officers?
- Is using machine learning more cost-effective than employing loan officers? How can ICS and human judgments be effectively combined?
- What strategies can increase MSME owners’ awareness about ICS to help them dispute unfavorable credit decisions and take corrective actions?
Understanding the discriminatory risks in credit scoring
- How do machine learning algorithms and loan officers respond differently to diverse demographic profiles?
- How can the discriminatory risks of machine learning be mitigated?
J-PAL SEA’s Innovation for MSME Development Initiative (IMDI) funds policy-relevant research and promotes knowledge-sharing among practitioners and policymakers who champion the use of rigorous evidence in the MSME sector. The IMDI team is open to discussing existing evidence, policy lessons and potential research questions and brainstorming ideas to advance MSME growth. To get involved, reach out to Rizka Diandra Firdaus (Policy and Communications Manager).