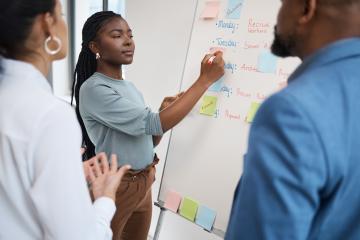
Researching racial equity: Administrative data bias
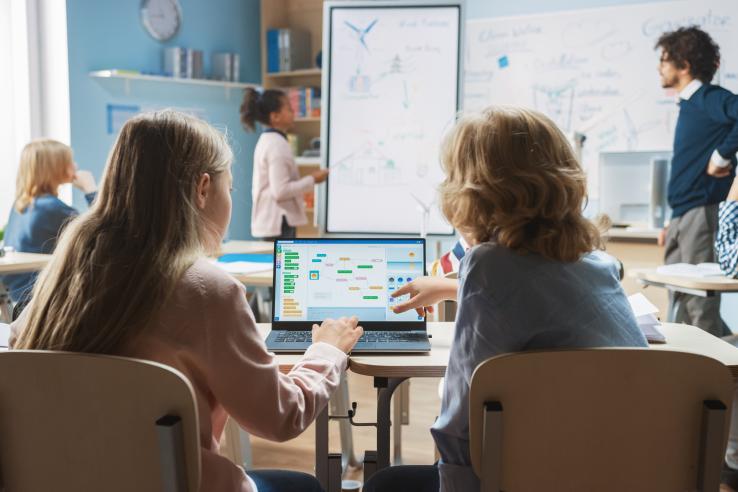
In J-PAL North America’s researching racial equity blog series, we discuss how research plays a critical role in identifying structural inequities in systems and policies that disproportionately affect communities of color. In part six of this blog series, we identify sources of bias in administrative data and describe these within the educational context.
Linking randomized evaluations to administrative data (such as medical records, education records, and tax records already collected by organizations for operational purposes) has greatly expanded the ability of researchers to examine program impacts on a wide range of outcomes, over longer periods of time, and at lower cost, with less burden on study participants. This has led to an increased fraction of academic studies published in top economic journals using administrative data over the past several decades. Administrative data is often considered less biased than survey data because it minimizes the risk of social desirability, enumerator, and recall biases. However, administrative data is not free from bias, and randomization — which ensures that systematic differences between intervention and comparison groups do not drive differences in outcomes — does not remove all bias inherent in existing datasets.
Administrative datasets are incredibly useful to researchers conducting randomized evaluations, but using administrative data for impact evaluation partially outsources choices over what and how to measure. These choices are subjective (reflecting an individual’s personal biases and perspectives) and reflect a researcher’s positionality (where one is situated in society based on their identities). In this post, we discuss how subjectivity and structural inequity can affect administrative data, provide examples from the educational context, and identify potential solutions applicable in education and other sectors.
Choosing what counts: How subjectivity shapes administrative records
What researchers choose to measure and how they choose to measure it shapes what we perceive as important and influences research priorities. These choices can reflect subjectivity that is often unexamined and unquestioned. For administrative data, these choices have important implications on representativeness by centering or decentering measurement methods that affect groups of people differently.
One example is the creation of gross national product (GNP) by Simon Kuznets in the 1930s. Tasked with developing a method to measure a nation’s income, Kuznets chose to exclude unpaid household labor, overlooking how domestic labor underpins population growth, employment, and business growth. This exclusion may have resulted from Kuznet’s personal bias, constraints in collecting household data, or a combination of these factors. Because of this choice, household labor is not explicitly reflected in macroeconomic datasets and, until childcare shortages became extreme due to Covid-19, has not been a focus of policy conversations about national growth. By omitting the economic contributions of groups predominantly involved in unpaid labor, notably women, this measurement choice might inadvertently undervalue their role. Economic policies and research, as a result, may not fully recognize or support the needs of these groups.
Examples from education
In the field of education, the influence of subjective choices is evident in standardized assessments. The development of educational standardized assessments was shaped by the views of individuals such as Carl Brigham, whose work was driven by an underlying racist belief: the notion that non-white students were intellectually inferior. A body of education literature has since lamented the biased assumptions that standardized tests are based on, and how results from tests inform critical policies, such as closing the racial and ethnic achievement gap. Despite concerns, standardized testing is a mainstay of the American education system. Understanding what assessments actually measure, what data is excluded, and how this affects groups of students differently, can help ensure that research works to mitigate bias instead of entrenching it.
Researchers can explore alternative non-test score measurements to complement or substitute for testing data. A recent study highlights an example of non-test score measurements, where teacher impact on student behaviors, measured by absenteeism, grade repetition rates, and suspension rates, are more influential on student long-term outcomes than teacher impacts on test scores. In this case, non-test data tell a different story than test data alone. Additional measures, such as family engagement rates, attendance, student discourse, and classroom engagement, can provide further insight into schools’ role in driving learning.
When relying on data from standardized tests, researchers can investigate the testing instrument itself for bias. The Center for Measurement Justice offers a validity framework to analyze bias intrinsic in an assessment. Considering the degree of bias can guide decisions on how to interpret research results and when to select additional data sources.
Structural Inequities: The hidden variable in administrative data
Organizations collecting administrative data exist within the context of systemic, institutionalized racism, where programming and the data collected from it reflect existing racial inequities in society. For example, administrative datasets on crime record only the individuals that police choose to investigate, not the individuals that police observe (or do not observe), a process subject to systemic bias through over-policing of neighborhoods and interpersonal bias from individual officers. This can lead to overrepresentation and underrepresentation of groups of people by race, ethnicity, and socioeconomic status in existing datasets. It is important to understand the context in which data is collected and research is conducted, so that outcomes that differ by racial and ethnic subgroups are analyzed and interpreted without relying on racist assumptions or stereotypes.
Examples from education
Education evaluations often use learning disability status as a criterion for defining study eligibility. Educators, parents, doctors, and others play instrumental roles in identifying and assessing students with learning disabilities, who are then eligible to receive additional classroom support. Identification and diagnosis processes are prone to conscious and unconscious bias and are embedded within unequal systems. Significant research has been devoted to understanding bias in special education placements, with some evidence suggesting that Black, Latino/a/e, and non-white students are over-referred for special education placements, while other evidence suggests these groups of students are under-referred, particularly for ADHD diagnoses, once other factors are taken into account.
Over and under representation in administrative data are important when considering program effects, particularly for subgroups defined by those data. Although randomized evaluations generate internally valid treatment effects, understanding how the sample and any subgroups are defined is important for interpretation, generalizability, and comparing subgroups. For example, the impact of a tutoring program may be over or understated because students eligible for participation may have a different distribution of learning disabilities than the data implies. When administrative categories are used for subgroup analyses, differential coverage in the data may explain observed differences in treatment effects. Care should be taken not to essentialize these differences as related to race, for example, rather than racism or factors that co-vary with race (see the section on Pursuing Rigor in our previous post on stratification economics).
Understanding implementation contexts can help assess whether eligibility criteria based on administrative data constructs are biased. The Institute of Education Sciences provides an implementation research toolkit so that researchers can understand implementation contexts and connect these to evidence generated from research.
Tools for navigating administrative data bias
To navigate bias, researchers should critically scrutinize data choices and embrace tools and frameworks that prioritize equity. Below are tips for navigating administrative data bias, along with tools and resources.
Be careful mapping indicators to constructs
Be explicit when theorizing connections between the indicators available in the data and the broader constructs you hope to capture. Problems can arise when researchers are too quick to connect indicators to constructs that reinforce rather than reject existing biases. Does a test score measure aptitude, learning, or resources? Disciplinary records may be a biased measure of behavior if discipline is not equitably distributed, but infractions may still be worth measuring if they are an outcome an intervention hopes to reduce.
Don't outsource outcome decisions to what is available in the data
While some researchers are genuinely interested in improving test scores, others may default to measuring learning in terms of test scores simply because they are available. This data equity framework gives examples and key considerations when choosing outcomes.
Understand where randomization mitigates (or doesn’t mitigate) bias
Bias that correlates with treatment status is most damaging to the ability to estimate causal effects. Systemic biases that are present in the data for both treatment and comparison groups will not affect the ability to estimate unbiased average treatment effects, usually computed as the differences in means between groups. However, more care needs to be taken to interpret the actual levels of an outcome for a particular group.
As noted above, the ability to measure outcomes for a particular group will be influenced by how that group appears in administrative data and may have implications for program targeting. A program to reduce disruptive behavior, for example, may not generate measurable effects in administrative data if that group of students is rarely the target of enforcement. Comparing a variety of characteristics of the underlying population, potentially eligible sample frame, and actual study sample can ensure that results are contextualized and generalizable. This resource also provides a framework for assessing racial and ethnic bias in administrative data.
Group identities themselves are both socially constructed and may be further limited by choices available in administrative data. Keep these facts in mind when interpreting results for subgroups in particular.
Consider supplementing administrative data with primary data collection
Asking participants to self-report identities like race and gender with thoughtfully created categories can address limitations often inherent in equivalent administrative data and may be relatively simple to include in an intake and consent process.
For guidance on these types of questions, see J-PAL’s inclusive language guide and We All Count’s data biography template.
Acknowledge limitations
Not every source of bias is correctable, but researchers should think critically about the potential for bias, discuss the limitations inherent in their research design, and take these into account when interpreting results and communicating with stakeholders and policymakers.
The researching racial equity blog series features the contributions of researchers and partners in examining and addressing racial inequities and offers resources and tools for further learning. Part one shares an example of evaluating racial discrimination in employment. Part two features work quantifying housing discrimination. Part three gives an overview of stratification economics in the context of evaluations. Part four discusses how to center lived experiences throughout the research process and in impact evaluations. Part five shares guidance for incorporating inclusive and asset-based framing throughout the research cycle. Lastly, in part seven, Damon Jones and J-PAL staff share progress on researching racial equity and future areas of work.