Why uncertainty in global health interventions matters—and what we can do about it
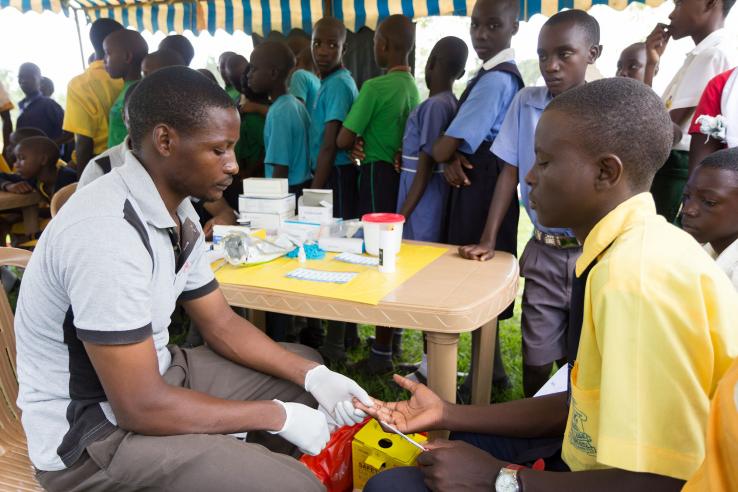
Global health interventions, like many public policies, are rife with uncertainty. Will a program, such as a malaria prevention strategy that looks strong on paper, work as intended? Will a new technology, such as a specific drug or device that appears effective in clinical trial settings, work in practice and provide good value-for-money?
In the case of programs made up of a complex interaction of multiple interventions, implementers often create a theory of change and then meticulously track whether it is being followed every step of the way, from each input translating into the prespecified activity, and the activities yielding the right outputs and the expected outcomes.
When observational data is available that permits quantitative analysis (evaluation), it may also be possible to estimate causal impact in a given setting by applying experimental methods (such as a randomized controlled trial) or quasi-experimental techniques (such as difference-in-difference analysis).
Such program evaluations generally consider outputs (e.g. the number of bed nets distributed) and relatively short-term outcomes (e.g. malaria infections following bed net distribution). Many evaluations also collect data years after the program to identify longer-term impacts. Cost-effectiveness calculations are sometimes conducted after ascertaining the cost and impact of the program, but such analyses aren’t necessarily considered when determining whether to implement a certain program or technology—especially when politics and other concerns get in the way.
Discrete clinical interventions and technologies (which are defined as including clinical interventions, drugs, diagnostics and even public health programs) are usually the subject of health technology assessment (HTA) to inform coverage decisions in many contexts. The underpinning evidence base for HTA typically involves a synthesis of randomized trial data, designed to reduce bias in estimating causal inference and relative effectiveness. Trial data is then combined with information from other sources and study designs to develop models of the technology’s long-term health and cost impact in a given context.
A key feature of both programs and technologies is uncertainty.
Success, as defined by expected impact, is not guaranteed—even when such interventions are implemented well. Understanding the sources of uncertainty is necessary for setting realistic expectations and for informing the collection of the right data at the right time to maximize impact.
This blog provides an overview of where this uncertainty can come from and outlines a few methods for addressing it.
Sources of uncertainty in programs and technologies
Uncertainty associated with programming
Some causes of uncertainty in programming are fairly straightforward. Perhaps a program goes over budget due to unforeseen circumstances, forcing on-the-spot adaptation. Perhaps a successful small-scale program fails at scale-up because the infrastructure was inadequate for serving the increase in demand.
Other causes of failure can be much more complex.
How humans react to a program can be challenging to predict but have vital consequences for a program’s effectiveness. For example, the Rwandan Ministry of Health launched a community-based environmental health promotion program focused on strengthening sanitation and hygiene practices through community hygiene clubs. It involved highly-trained facilitators that led households through weekly sessions using high-quality instruction to convey the importance of improved hygiene.
Researchers worked with the Ministry of Health to conduct a randomized evaluation (Sinharoy et al. 2017) to determine the program’s impact and found that while there was an increase in households self-reporting treating their drinking water, the program had no impact on rates of exclusive breastfeeding, diarrhea, or nutritional outcomes.
This lack of effect would likely not have been detected without an impact evaluation, further highlighting the need for greater use of evaluations and other tools to identify when programs don’t achieve their expected outcomes.
In other cases, however, the impact of behavior on program effectiveness may not be immediately apparent—a program could work in the short term, only to see its effects disappear over time.
In India, an NGO implemented a program to reduce nurse absenteeism. A randomized evaluation (Banerjee et al. 2008) found that using password-protected time- and date-stamping machines in conjunction with pay withholding for absenteeism initially increased nurse attendance by 15 percentage points. But 14 months later, this increase disappeared.
Nurses broke the machines, while administrators began to excuse all absences. Ultimately, fewer patients were being seen per day after the program than before it was rolled out.
These types of unintended consequences that stem from human behavior can be challenging to predict, but essential to track and, to the extent possible, predict before implementation.
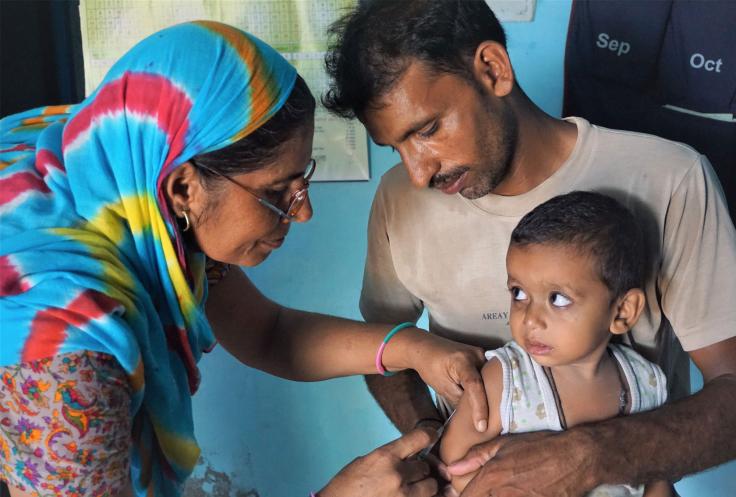
Photo: A nurse immunizes a child in India. Shobhini Mukerji | J-PAL
Uncertainty associated with introducing individual technologies
The roll out of GeneXpert, a diagnostic for tuberculosis endorsed by WHO and major development partners such as UNITAID and GFATM, provides a useful example for how uncertainty can affect downstream impacts.
While mathematical models had predicted that the diagnostic technology would save health care systems money, analyses post-launch and national roll-out in countries such as Brazil and South Africa revealed a different picture.
Empirical treatment practices, adherence to protocols by providers and patients, and availability of treatments post-diagnosis all reduced GeneXpert’s impact and/or inflated its cost, reducing its value for money and making the technology less of a breakthrough than had originally been expected. Furthermore, the contract between payers and the sole manufacturer was set up such that users had to pay for installation, power, cartridges, and servicing, which proved difficult to sustain in some cases.
Similarly, a lack of a systematic process for assessing the evidence around next-generation malaria nets, quantifying any uncertainty, and then agreeing on how to deal with it has delayed roll out. It may also, much like the GeneXpert case, compromise its ultimate value for money.
This type of miscalculation can have drastic impacts on budgets, especially as low- and lower middle-income countries such as Kenya are increasingly taking over health system financing. These governments must make unavoidable trade-offs with limited funds when determining which programs and technologies to implement. Paying for something that turns out to be more expensive and less effective than expected makes the trade-offs all the more acute.
Addressing uncertainty
Finding common ground
Whether we are considering relatively complex programs or discrete technologies, the sources of uncertainty are very similar.
These include uncertainty in key inputs (such as resource use and costs), uncertainty around a program or technology’s causal effects, and uncertainty associated with how the intervention will be implemented in practice, which may be linked with unintended or suboptimal outcomes.
How we address the uncertainty depends on the intervention and source of uncertainty, but it almost always involves the use of data and evidence.
Adopting methods appropriate to the job
Stakeholders involved in the generation and use of evidence have often disagreed on the most appropriate methods to be deployed when evaluating interventions in global health. These disagreements often turn on issues related to causal inference and effect size, and on the appropriateness (or otherwise) of randomized controlled trials to a given context.
Various groups of health stakeholders can have different perspectives on the generation and use of knowledge, including researchers who prioritize randomization, program evaluators focusing on other methods, health economists, econometricians, health services researchers, clinical trialists, and the list goes on.
These factions (with several of which we identify) are important, but our focus here is to identify the commonalities between them.
First, it is our contention that there isn’t a single source of evidence that can eliminate all uncertainty associated with the adoption of programs and technologies, and which adequately informs context-relevant policy questions. Rather, it is necessary to understand the different shortcomings of different study designs (randomized or otherwise) and their relative appropriateness to address different aspects of the policy question being considered.
For instance, well-designed randomized trials and evaluations are a robust method for evaluating whether a program is achieving its expected impact, and evidence from them can answer many questions on how to design a program or policy to be maximally effective.
However, we also encounter programs and contexts where randomization is not appropriate or possible. Perhaps the sample size is too small. Perhaps the cost of data collection is too high. Or maybe the program has already been implemented with no plans for expansion. Relying on one study design in all situations without consideration of its appropriateness, given the context and the policy question at hand, can lead to misunderstanding and futile debate.
In many instances, it may also be appropriate to consider a body of evidence that comprises multiple study designs (such as complementing quantitative evidence with qualitative) to fully understand all aspects of the policy question at hand.
Combining data in this way is a feature of so-called decision analytic modelling, commonly applied in HTA.
While a randomized evaluation can speak to a technology’s impact, it doesn’t always explain why we see those impacts. This represents an important difference between evaluation of programs and technologies: It tends to be easier to design a randomized evaluation that identifies the mechanisms behind why a program is successful (for example, if you want to know if information provision or incentives are most effective to achieve a certain outcome, you can test one against the other). With technologies, it is more difficult to test different components of a technology against each other—often it either works or it doesn’t.
With this in mind, it is important to recognize the limitations of each evaluation method alone and combine information and lessons from multiple methods.
Second, and on a related point, the availability of evidence should not determine the relevant policy questions to be answered, but it may mean that the best course of action is further research. This is especially true in instances with high levels of uncertainty that may be at least partially addressed by further research prior to technology roll-out or program implementation.1
Third, there is disagreement (or different perspectives) among stakeholders on the technical and value judgements needed for determining which findings and data should be incorporated into decision-making.
One way this can be described and addressed is by defining a Reference Case, a tool developed by iDSI Health. This can provide decision makers with relevant and reliable ways to determine the likely implications of implementing a treatment or health service in specific contexts.
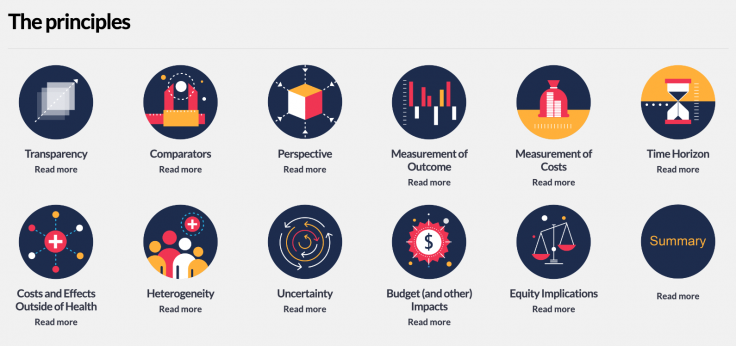
Graphic: iDSI Reference Case Principles
Finally, careful analyses are needed both before program/technology roll-out and as part of it. These analyses should be based on decision frameworks (such as context-specific Reference Cases as noted above, but adapted to different interventional types).
They should consider all evidence aspects relevant for policy-makers, including the issue of uncertainty and its possible impact on expected outcomes. Roll-out could then be seen as an opportunity to collect more evidence, especially in areas that are most uncertain.
Motivating evidence use
Addressing uncertainty requires stakeholders to acknowledge that uncertainty exists, recognize that it matters every single time an investment (or disinvestment) decision is made, and use evidence to address it.
For example, large, complex development programs such as the Global Fund to Fight AIDS, Tuberculosis and Malaria, the Global Financing Facility, and Gavi involve roll-out of both interventions and technologies. They use investment cases to inform replenishment processes and often rely on modelled, impact assessment claims that also carry uncertainty.
This uncertainty ought to be quantified alongside a credible narrative for ways of addressing it after the investment is made. A critical element in such an assessment of uncertainty, and indeed in being able to address it, is the inclusion of impact information and cost-effectiveness data. This type of economic information, however, is often excluded even from the simplest value assessment of individual healthcare commodities.2
In the longer term, explicit acknowledgment of uncertainty by budget holders in global development—stakeholders at foundations, bilaterals, multilaterals, national governments, and others—would help increase the use of evidence, including economic evidence, to better understand and address the consequences of decision error when investment decisions are made.
It would also create the right incentives for technology manufacturers or program advocates to invest in evidence generation prior, during and after an intervention or program is rolled out.
The process of addressing uncertainty about the value of a technological innovation can, pre roll-out, inform pricing negotiations or, in the case of a programmatic intervention, scheme design. During roll-out it can form part of a formative evaluation. After roll-out, it can inform a review of a country’s benefits package to delist an intervention not shown to work in the real world or review of an impact assessment.
Such course correction approaches remain rare in development but are very common in upper middle- and high-income country markets, at least when it comes to commodities. In lower-income countries, support by intelligent information technology can help develop a learning healthcare system that, coupled with formative research, reduces uncertainty around effects of interventions and programs and eventually becomes the norm.
It’s ok to be unsure—just be explicit about it, and be prepared to change your mind
Uncertainty is inevitable when seeking to implement interventions to enhance global health. It needs to be acknowledged explicitly as part of a process to generate evidence suitable for decision making. Analyses before implementation are needed to flag the consequences of getting a decision wrong and to facilitate better implementation and subsequent course correction.
Accepting and quantifying uncertainty before major decisions is instrumentally and intrinsically important, whether the subject of evaluation are technologies, more complex program interventions, or even whole agencies like the Global Fund.
Finally, evidence generation is a continuous process, and the appropriate course of action cannot be settled within a single iteration.
Targeted data collection forms an integral part of rolling out an intervention and the evidence thus derived can then be fed into a process of review that helps ensure decisions are kept up to date, represent good value for money and can be defended to all those affected by them.
__________
1. See here for a discussion of dealing with uncertainty in the context of making a reimbursement decision for a new health technology.
2. For a discussion of the importance of costs, including opportunity costs in WHO norm setting in relation to evidence informed decision making, see here and here. In the case of the Global Fund, see here and here.