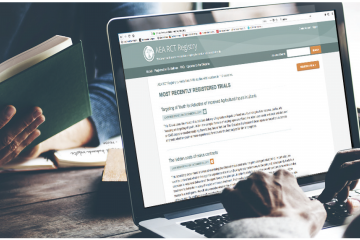
Newly published data on health care hotspotting study underscores the importance of research transparency
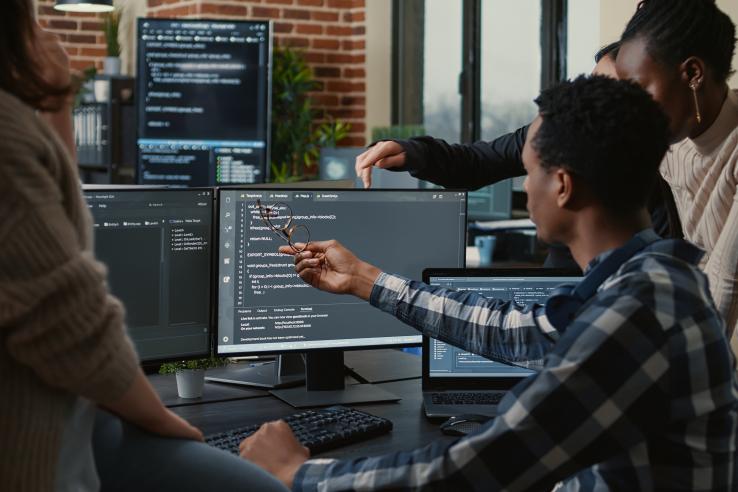
This month, our team published de-identified data needed to replicate the results in Finkelstein and coauthors’ 2020 Health Care Hotspotting randomized controlled trial. This study showed that a much-lauded care coordination program failed to reduce hospital readmissions for “super-utilizers'' of the health care system with complex medical and social needs. The paper was the result of a long-term partnership between J-PAL affiliated researchers and the Camden Coalition of Healthcare Providers dating back to 2014.
Although this paper came out in January 2020, we’ve just now been able to share the data. We made this effort, even after the project was done, because producing public use data is an important component of transparent, reproducible science.
Why publish data?
Publishing replication data allows other researchers to confirm and reproduce research results. This means researchers can check the work of others by looking at the data and running their code to make sure it is accurate. It also ensures the original researchers did not selectively publish only the results that fit their hypotheses. Transparency in research, including openly sharing data, plays a key role in making science credible.
Publishing data also enables researchers to perform new analyses and examine hypotheses that may not have been covered by the original investigators. For example, when Finkelstein and colleagues published data from the Oregon Health Insurance Experiment, other independent researchers were able to investigate who was driving the increase in emergency department use as a result of Medicaid enrollment, with novel findings that the original team had not considered. In addition, publishing data allows the research to be included in meta-analyses, so that we can learn from the combined results of multiple studies. For research questions such as ours, where we’ve seen mixed evidence on similar programs, this is particularly important.
Building and cleaning a dataset takes time and effort. By making our data available, that work can benefit others. Researchers embarking on new evaluations of similar programs can now use our data to inform power calculations. And students and teachers can use our data to learn about research methods, exploring the role of regression to the mean to understand how a randomized evaluation was critical to unpacking the null effect of the program.
Considerations for publishing your own data
We’ve learned a lot about the process of publishing replication data over the past couple of years. Here are five key considerations we encourage other researchers to keep in mind as they seek to publish their own data:
- Plan for publishing replication data from the start of the research project. Publishing this data took as long as it did because our research uses administrative data from several Camden, New Jersey hospitals, and our data use agreements did not stipulate whether we could publish data. After publishing our paper, we worked with the hospital IRBs and our partners at the Camden Coalition to clarify our intent to publish and win required approvals. Thankfully, everyone involved saw the value of sharing data, but a preferable strategy would be to plan for publishing replication data from the start.
- Make clear to participants what you will do with their data through the informed consent process. This allows participants to make informed choices about study participation, and this is often the group that is hardest to go back to later if additional authorization is needed.
- Get IRB approval for data publication. This ensures some institutional oversight and that data publication has been weighed as part of the benefits and risks of the study.
- Codify the ability to publish data in data use agreements for administrative data. We failed to build data publication into data use agreements either because data providers required standard language or we feared raising it early would jeopardize access to data. With the benefit of hindsight, I recommend you get an early start. Universities already require that agreements allow academic freedom to publish results. We should think of the right to publish data as similarly important, particularly in cases where there are not clear ways for other researchers to acquire the data directly.
- Protect participant confidentiality. Publishing data needs to be balanced with protecting the personal information of study participants. We ensured that data was de-identified, following guidelines from HIPAA (the key US health care data law) to remove eighteen identifiers. We also removed or coded additional variables, like the names of hospitals and participant age, which, although not required, further reduced the likelihood of re-identifying participants. Researchers should understand the legal restrictions on their data (especially when working in sensitive topic areas or with vulnerable populations), follow requirements in data use agreements, and conduct their own risk assessments.
In our case, we can’t share all of our data, as some of our data use agreements explicitly prohibit publishing data in disaggregated form. That was the price of receiving data on the take up of government benefits, for example. But we can share the main outcomes related to hospital use, which is a great place to start.
Replication data is not the only component of research transparency. It goes hand in hand with other best practices like trial registration. And statistical replication is not the same thing as replicating the concepts of the study in another location. Still, it’s an important starting point, and we’re happy to have finally accomplished it for this study.
We encourage everyone to download our code and data and take a look around. See if you can recreate our tables and figures. See what new insights you can find. And let us know what you discover.
If you’re publishing data on your project, J-PAL has handy guides on data de-identification and publication.