Targeting Central Lead Farmers to Boost Agricultural Technology Adoption in Malawi
- Farmers
- Technology adoption
- Fertilizer and agricultural inputs
- Information
- Social networks
Many farmers in sub-Saharan Africa may be reluctant to adopt productivity-enhancing technologies because they lack persuasive information on the proper use of new inputs or agricultural techniques from credible sources. Researchers conducted a randomized evaluation to test whether the position of a trained lead farmer within a community’s social network affected other farmers’ decisions to adopt a new agricultural technology in Malawi. Results suggest that having access to multiple lead farmers/central individuals has the potential to increase adoption and speed the diffusion process of the technology. In other words, the researchers showed that targeting multiple central lead farmers was necessary to generate technology adoption in Malawi.
Policy issue
Agricultural productivity growth in sub-Saharan Africa has lagged behind the rest of the world, largely due to farmers’ low adoption of productivity-enhancing technologies. One reason farmers can be reluctant to adopt new technologies is a lack of credible information about the proper use and benefits of them. As a result, governments or NGOs seeking to promote technology adoption, generally do so through agricultural extension systems that rely on professional agents to provide farmers with information about farming practices and agricultural technologies. Because these systems often have limited resources or capacity, they rely on social networks to further diffuse information through farmer-to-farmer learning.
While existing research has documented faster diffusion when technologies are disseminated by people who are central in their social network (meaning that they are well-connected), it is still unclear whether it is the connectedness of a given farmer, or the number of agents and farmers in a social network simultaneously disseminating the information that leads to faster diffusion. Thus, understanding the number of informed connections farmers need before they adopt technologies themselves can help policymakers leverage social relationships in targeting information within agricultural extension systems. Can targeting information to farmers at different positions in their social network impact the diffusion and adoption of agricultural technologies?
Context of the evaluation
Malawi is a predominantly rural country where agriculture is a main source of employment and growth. Approximately 80 percent of Malawi’s population live in rural areas where maize dominates agricultural production, with 97 percent of farmers growing maize. Thus, technology adoption and productivity improvements to the crop are closely tied to welfare.
Maize farmers in Malawi traditionally plant seeds in either flat land or use a ridging technique that has been shown to deplete soil fertility and decrease productivity over time. Alternatively, pit planting is a technique that minimizes soil disturbance and involves planting seeds in a shallow pit to retain moisture in arid climates. While pit planting has shown to greatly improve maize yields in other contexts, it was a new technology in Malawi: only 0.2 percent of farmers in the study reported planting with pits in the year prior to the evaluation.
New agricultural technologies are often disseminated through an extension system operated by the Malawian Ministry of Agriculture and Food Security. The system relies on extension agents who are each responsible for thirty villages or more, resulting in little direct contact with farmers. For instance, in the 2007 national census, only 18 percent of farmers reported participating in any type of extension activity. Extension agents cope with staff shortages by training lead farmers (well-connected farmers who promote the technologies in their communities) to disseminate technologies through social learning. Thus, maximizing the reach of social learning for technology diffusion may be a cost-effective way to improve the efficiency of Malawi’s extension system.
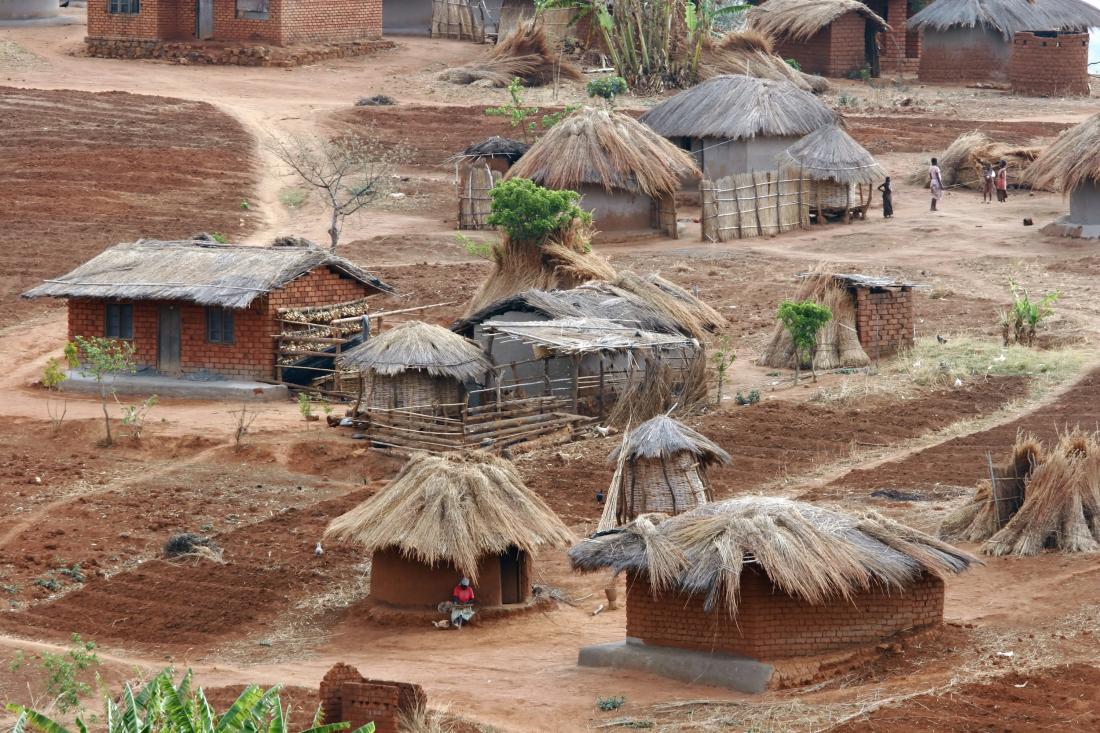
Details of the intervention
Following up on a similar intervention, Promoting Sustainable Farming Practices in Malawi, researchers conducted a randomized evaluation to study the impact of social network-based information dissemination on technology adoption. In collaboration with the Malawian Ministry of Agriculture and Food Security, researchers modified the existing extension program to promote two technologies: pit planting and better crop residue management (CRM), which emphasizes retaining residue to improve soil quality.
In total, the program included approximately 5,600 households across 200 villages in three districts: Machinga, Mwanza, and Nkhotakota. In each village, extension agents trained two chosen lead farmers to promote the technologies among their communities. To identify potential lead farmers, researchers conducted a census in each village to map out social networks. Researchers then randomly assigned each village to one of four variations of the program, in which lead farmers were chosen through different selection methods while all other aspects of the program remained the same:
- Complex contagion network model: Lead farmers were chosen based on a social network model that assumed that a farmer must know at least two other farmers, who are knowledgeable about a technology, in order to be convinced to adopt it. This is known as “complex contagion.” Accordingly, the two lead farmers chosen in each of these villages were both central in the social network, where crucially they were likely to share common connections.
- Simple contagion network model: Lead farmers were chosen based on a social network model that assumed that farmers only need to know one other farmer who has adopted a technology, to be convinced to adopt it themselves. This is known as “simple contagion,” the way in which a virus spreads. In these villages one of the lead farmers was network-central while the second was typically less connected in order to also reach households who were more socially distant, or on the periphery of the social network.
- Proximity network model: Lead farmers were chosen based on the complex contagion social network model, but individuals were considered socially linked based on geographic proximity rather than the social census. These lead farmers were typically not central, but close to each other in the social network. This was introduced as a cost-effective alternative to the simple and complex network models to account for scalability, given the high costs associated with collecting social census data.
- Comparison: Lead farmers were chosen at the discretion of the responsible extension agents in accordance with the existing extension system. These farmers were shown to have an intermediate level of centrality in the social network.
In addition to receiving training, lead farmers received a small in-kind gift (valued at US$8) if they adopted pit planting in the first year of the program. No incentive was offered in the subsequent years, or for adopting CRM, or for convincing other farmers in the village to adopt either technology.
Lead farmers were selected and trained in 2011 in Mwanza and Machinga; and in 2012 in Nkhotakota. Over the next three agricultural seasons from 2011–2013, researchers collected information from households on farming techniques, technology adoption, yields, and other demographic data. Social network census data was also collected in order to understand with whom households in the study consulted when making agricultural decisions.
Results and policy lessons
Overall, the program convinced lead farmers to adopt pit planting. While technology adoption by non-lead farmers was fairly low across all groups, villages in which multiple central farmers were targeted with information exhibited increases in adoption relative to comparison villages, largely driven by households who had links to both trained lead farmers.
Lead farmer technology adoption: Over the three years, the program increased the knowledge and adoption rate of pit planting among lead farmers across all groups, suggesting that the training and incentives may have induced take up. Compared to comparable farmers, trained lead farmers were 25.8 percentage points more (i.e., greater than four times) likely to adopt pit planting in the first year. As a result, 30 percent of lead farmers compared to 5 percent of shadow lead farmers adopted the technology during the first year of the program.
Non-lead farmer technology adoption: While there was largely no difference in pit planting adoption in the first season across villages, adoption was significantly higher in network-targeted villages in seasons two and three. In season two, adoption rates among non-lead farmers increased by 3.6 percentage points (a 95 percent increase) in both simple and complex network villages, relative to comparison villages where the adoption rate was 3.8 percent. In the third season, among villages where knowledge of pit planting was comparatively low at the start of the research, the adoption rate in the complex network group was higher compared to both the simple network and comparison groups. This suggests that targeting well-connected lead farmers was most effective in the villages where the information was most valuable. At the end of the program, in 45 percent of the comparison villages, not even one household adopted pit planting. In contrast, there was a 56 percent greater likelihood that at least one non-lead farmer in complex network villages adopted the technology, relative to the comparison villages.
The increase in knowledge and adoption in network-targeted villages was largely driven by farmers who had links to both trained lead farmers, suggesting that the complex social network model was more effective than the simple model. Households who had connections to two lead farmers were 8.4 percentage points (a 33 percent increase) more likely to have heard about pit planting in season one and 6.2 percentage points (a 108 percent increase) more likely to report knowing how to pit plant relative to households with no connections. Furthermore, these households were 3.9 percentage points (a 90 percent increase) more likely to adopt the technique in the second year than those with no connections.
These results demonstrate that strategically targeting information to well-connected lead farmers can be effective in accelerating the diffusion of new agricultural technologies. Further research on other simple and inexpensive procedures to identify central farmers could make network-based targeting more policy relevant and scalable.
Beaman, Lori, Ariel BenYishay, Jeremy Magruder, and Ahmed Mushfiq Mobarak. “Can Network Theory based Targeting Increase Technology Adoption?” Working Paper, November 2014. (DO NOT UPLOAD TO WEBSITE)