Using Mobile Phone and Satellite Data to Target Togo's Emergency Cash Transfer Program
Project Summary
Location: Togo
Sample: People living in Togo’s 100 poorest cantons
Timeline: 2020
J-PAL Initiatives providing funding: Innovation in Government Initiative (IGI)
Target group: Adults; families and households; rural population
Outcome of interest: Social service delivery
Covid-19 dimensions (please highlight the dimensions studied): Access to government relief measures; cash transfers
Mode of data collection: Mobile phone metadata (call detail records); satellite imagery; surveys
Nature of activities: Developing and evaluating an approach to targeting social assistance based on machine learning algorithms and data from satellites and mobile phone networks
Research paper: Aiken, Emily, Suzanne Bellue, Dean Karlan, Chris Udry, and Joshua E. Blumenstock. "Machine learning and phone data can improve targeting of humanitarian aid." Nature 603, no. 7903 (2022): 864-870. https://doi.org/10.1038/s41586-022-04484-9.
Project Details
In Togo, a small country in West Africa, over 50 percent of the population lives below the international poverty line. The Covid-19 pandemic threatened to reverse years of poverty reductions, as lockdown measures designed to stop the spread of the virus limited economic activity and threatened food security.
In response to the crisis, the Government of Togo launched Novissi, an innovative social assistance program designed to provide emergency cash assistance to Togo’s neediest families. Over the course of a few weeks, the government built and deployed a contactless, digital system that provided over a half million people with cash. Beneficiaries registered using their mobile phones (no smartphone was required); after entering basic information into a menu, they were sent mobile money transfers of approximately US$20 per month, lasting for three months.
A key question for Novissi was how to prioritize those people with the greatest need. The difficulty was that the Government of Togo did not have a comprehensive social registry that would allow them to directly identify its poorest people. The last census was conducted in 2011, and even that database did not have any information that could allow the government to determine who should be prioritized for assistance. In the middle of a pandemic, it was impractical to collect the data required to create a new registry. When the government launched Novissi in April 2020, it prioritized informal workers living in the regions most impacted by the lockdown restrictions. To determine eligibility, they relied on a voter database that had been recently updated, and in which people had self-declared their home location and their occupation.
At the conclusion of Novissi’s first phase, researchers worked with the Government of Togo and GiveDirectly to expand the program to rural areas of the country, where extreme poverty was most severe. The Government of Togo had two policy goals in expanding Novissi to rural areas: (1) prioritize benefits to people living in Togo’s poorest cantons (the smallest administrative unit), and (2) prioritize the poorest people in the poorest cantons.
Intervention Details
The researchers’ approach to expanding Novissi used machine learning to analyze non-traditional data from satellites and mobile phone networks and featured two distinct steps.
1. Prioritizing the poorest villages and neighborhoods: The Government of Togo was interested in prioritizing support to the 100 poorest cantons of the country, but they did not possess a poverty map that would allow for such granular targeting. Thus, the research team produced micro-estimates of the relative wealth of 2.4km square “cells” within a geographic grid by applying machine-learning algorithms to high-resolution satellite imagery. These estimates provided an indication of the relative wealth of all households in each small grid cell; researchers took the population-weighted average of these grid cells to estimate the average wealth of each canton. See Figure 1 below for an illustration.
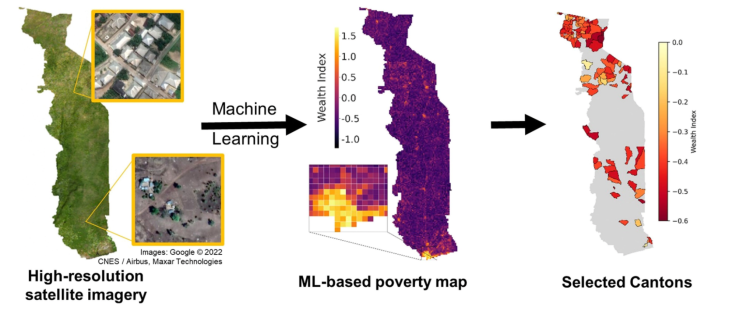
Figure: Emily Aiken, Suzanne Bellue, Joshua Blumenstock, Dean Karlan, and Chris Udry in Nature
2. Prioritizing the poorest people: In expanding Novissi to rural areas, the government wanted to ensure that benefits flowed to the poorest people living in the 100 poorest cantons. To do this, the research team estimated the average daily consumption of each mobile phone subscriber by applying machine-learning algorithms to mobile phone metadata. Specifically, they conducted surveys with mobile phone subscribers, used the surveys to measure the wealth and/or consumption of each subscriber, and then matched the survey-based estimates to detailed metadata on each subscriber’s history of phone use. This was used to train machine-learning algorithms to predict wealth and consumption from mobile phone use. See Figure 2 below for an illustration.
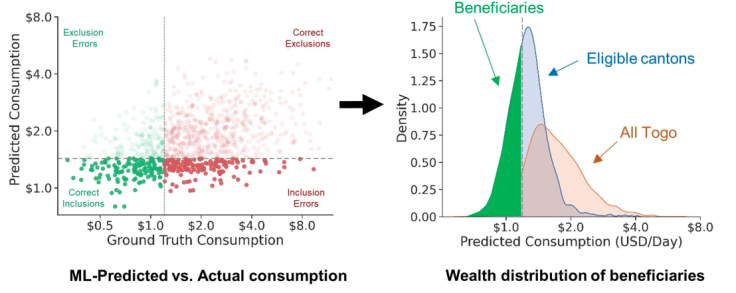
Figure: Emily Aiken, Suzanne Bellue, Joshua Blumenstock, Dean Karlan, and Chris Udry in Nature
Research Results
To evaluate the performance of the new targeting approach that combined machine learning and mobile phone data, the researchers compared targeting errors using this approach to targeting errors under different targeting regimes.
Compared to a “geographic blanketing” approach that would provide benefits to all people residing in specific villages, the machine-learning approach reduced the number of people who would have been inaccurately excluded from cash transfers by 4–21 percent. Researchers also found that the machine learning algorithm did not unfairly exclude women or any ethnic, religious, or age group.
Researchers estimated that traditional targeting methods (for example, proxy-means testing) would have performed even better than the machine learning approach had there been a detailed social registry from which to construct the appropriate measures. However, the Government of Togo did not have a traditional social registry that could have been used to assess program eligibility when it launched Novissi, and it did not have the time or resources to build such a registry in the midst of the pandemic.
Taken together, the results suggest that the machine-learning approach is a rapid and cost-effective way to target aid in the absence of up-to-date and detailed data sources, but that it should not replace more traditional targeting methods when the data and timeline permit it. The findings highlight the potential for new data sources to complement traditional methods for targeting humanitarian assistance, particularly in crisis settings in which traditional data is missing or out-of-date.