Real-time monitoring and response plans: Creating procedures
Summary
When an unintended change arises in a study of a social program or policy, there is often a limited window during which to address it. Systematic monitoring plans can equip researchers and program staff to detect changes and make course corrections in real time if elements of the study deviate from the original plans.
Key takeaways
- Real-time monitoring alerts you to what you should be concerned about. If you’re not aware of any concerns at all, this may be a sign that you are missing information!
- Key indicators to monitor in randomized evaluations often include: fidelity to randomization procedures; take-up rates; and indicators of data quality.
- Automated systems for monitoring and generating red flags are useful, but only if a person is designated to actively review them.
- There is no substitute for regular, frequent interactions with an implementing partner. Regular visits or calls can alert you to concerns as they arise.
While our companion resource on implementation monitoring covers the overall monitoring and documentation of a study (for example, interviews with staff and procedures for periodic, detailed back-checks), this resource is a more procedural guide for action plans around key indicators of changes and concerns.
Introduction
A good monitoring procedure helps researchers learn about major deviations from plans by observing indicators of how the intervention, data collection, and other essential processes (e.g., data transfer or informed consent) are operating. Researchers should identify a limited set of need-to-know indicators and decide how to track them. These monitoring activities should continue throughout the design, pilot, and implementation of a randomized evaluation. This resource guides readers to:
- Choose which indicators are most important to include in a real-time monitoring procedure,
- Organize incoming data with automatically generated checks and flags, and
- Set up channels of communication and plans for reviewing data and reporting concerns.
Choose indicators and data
Indicators
Choosing a limited set of indicators1 helps to cut through the noise of available information and alert the research team to changes and concerns.
Key indicators to monitor and check in real time may relate to data quality (e.g., the match rate when matching datasets), randomization fidelity, or rate of recruitment. Examples of key indicators include: “duration of each tutoring session” as an indicator of the implementation fidelity for a tutoring program; or “number of deviations from survey script” in a study of a health care intervention––as measured in a weekly random audit of audio files of phone surveys––as an indicator of the appropriateness of the script or of how well staff understand protocols.
Selectivity in choosing key indicators enables researchers to identify important patterns and red flags quickly. Use your research questions, in conjunction with your budget and resource constraints, to identify the most important elements of the study to monitor actively. These considerations can help in the selection of indicators for a quick-response monitoring plan:
- Use research questions and practical constraints to decide which indicators to include in a real-time monitoring action plan and how frequently to check them. Consider the relative severity of possible concerns, budget, and staff capacity when choosing which indicators to monitor actively and how frequently to check them.
- Identify the points in a study when it is most important to follow protocols (or in other words, where there are the most concerns about things going wrong) and discuss these ahead of time with stakeholders. Researchers and implementers should discuss (1) what problems have arisen in previous versions of the intervention and (2) whether the study is introducing changes to the intervention. Pay extra attention to these areas in future communication.
- Assess the status quo before the study begins. Measure benchmarks of implementation for future comparison.
Data sources and data collection
Assess what data is available to report on key indicators and determine how to gather the information you need. Data sources for monitoring plans may include, for example: summary statistics of administrative data that are sent to researchers at regular intervals; survey responses sent to researchers automatically from electronic devices; or qualitative data shared during team calls.
Consider whether it is feasible to make data collection monitoring and review procedures the same for treatment and control (in order to preserve the ability to attribute differences to the treatment itself) or whether these need to be different due to practical constraints (Glennerster and Takavarasha 2013, 306). The monitoring methods themselves might affect the impacts of an intervention or policy. For example, if a monitoring plan includes asking people about the treatments they are or are not receiving, this might encourage them to seek treatment (Glennerster and Takavarasha 2013, 306). Research teams can assess these possibilities with the help of site visits, data, and conversations with partners.
Depending on the existing data systems of implementing partners, it may be mutually helpful for the research team and partners to work together to update systems and data collection procedures to support consistent implementation and monitoring.
Create monitoring tables and reports
Monitoring tables typically display a small set of indicators such as treatment intensity (if applicable) and the balance of indicators across treatment and control groups. See the appendix for an example of a monitoring table. Deviations that exceed thresholds of anticipated variation can prompt researchers to investigate further. Tables and reports should complement open communication with partners and within the research team.
Set up automatic flags and thresholds
Set up flags within monitoring tables and design procedures to ensure that implementers and research staff are empowered to report possible concerns even if they are not certain about what might be happening. Automatic red flags can prompt the designated reviewer to report concerns to the appropriate party. For example, if variable A drops below a certain number, then alert the primary investigator; if variable B increases rapidly, then talk with an implementing partner. There should also be defined ways to raise concerns based on information that falls below pre-set reporting thresholds but still causes doubt about whether all is as running as intended.
Balance checks test whether randomization is working as planned by testing for differences in observable characteristics between the treatment and control groups. High-frequency checks can be a helpful way to streamline a process of automatic flags and checks. Innovations for Poverty Action (IPA) has developed Stata templates for high-frequency checks designed for population-based survey research and randomized impact evaluations. These templates run high-frequency monitoring checks of incoming research data.
Considerations to guide the creation of monitoring tables
Randomization: For studies with rolling or on-the-spot randomization, monitoring tables should automatically check the balance of key variables or of the enrollment rates into treatment versus control groups.2 If there is imbalance that exceeds anticipated margins, the research team should investigate potential causes of imbalance, which may include intentional or unintentional deviation from randomization processes.
For example, consider an intervention of intensive job placement services, randomized by day, with the randomization schedule determined weeks in advance and distributed among staff at a job services center. If many more job seekers tend to come to the center for services on treatment days, researchers might work with staff to determine why this is happening. Staff might investigate whether the randomization schedule has accidentally been posted in an area of the office where it is visible to job seekers, or whether job seekers are recommending services to friends who come in on the same day (which may in fact be a desired outcome), or whether other factors are involved.
Take-up and participation: Track indicators of how many of the individuals or groups assigned to treatment choose to take up (participate in) the intervention and how intensively people are participating. For example, if an intervention requires participants to attend trainings or other sessions, track attendance rates over time. If take-up or participation are falling below anticipated levels, this may have implications for statistical power and may indicate deviations from intended protocols for the study or for the intervention itself.
Technology: Monitoring tables can help to detect when code, hardware, or other technologies create unanticipated problems. If a study relies heavily on certain technologies—for example, tablets for administering surveys—researchers should determine ways to check functionality. For a study that relies on survey tablets, monitoring tables might include hard-coded indicators, such as percentage of surveys successfully completed once started, along with regular channels for soliciting feedback from survey enumerators to ask whether they are encountering difficulties with the tablets.
Even with careful monitoring plans, problems may still pass undetected. For example, if the logic of the code behind a technology-based intervention were to change as part of software updates unrelated to the study, this might affect random assignment and treatment.3 Monitoring of the first stage, along with open and frequent channels for communication, can help make it more likely that researchers and implementers can prevent and detect such challenges.
Case study: Undetected code changes or errors may compromise treatment fidelity. In one study, health care providers were assigned to either receive a pop-up “nudge” alert through an electronic medical record software program (treatment), or to continue as usual (control). Partway through the study, an undetected change in the intervention software altered the inclusion criteria for when the nudge appeared, resulting in providers in the control group seeing the treatment nudge. This change occurred months into the study, and researchers did not find out about the change until data collection had been completed. The research team was able to use the data, but it answered a different research question than was intended.
A well-implemented monitoring and tracking procedure may have been able to bring researchers’ attention to this error when it occurred. When possible, a monitoring plan that tracks treatment intensity (for example, the appearance of nudges for the treatment group and the control group), should automatically flag causes for concern (in this example, by alerting researchers if anyone in the control group receives a nudge). This type of flag facilitates open channels of communication between researchers and staff at implementing organizations. The combination of these systems may help to detect or avert unintended effects of changes.
Establish procedures for communication, reviewing data, and reporting concerns
The research team should make a plan for reviewing monitoring tables and responding to concerns. The following steps will help the research team make the best use of monitoring tables and other sources of information.
Designate a primary reviewer
Designate one person to review the few absolutely key indicators in monitoring tables at regular, realistic, and clearly defined intervals. By reviewing tables regularly, the designated person will be able to detect and report if there are major changes, discrepancies, or other red flags in how the intervention is being implemented. Detailed plans for checking and responding to monitoring data are essential: without sufficient, yet manageable, procedures, monitoring tables may become overlooked and therefore ineffective. A good plan will catch major causes for concern and will be reasonable for staff to balance with their other responsibilities.
Lower barriers to raising concerns about data and protocols
Although it is not possible to pre-specify how to respond to every possible situation, it can be helpful to set a few important thresholds (see above) and procedures to enable quick responses. This is especially important if junior staff, such as Research Associates (also known as RAs or Research Assistants), will be reviewing data and triaging concerns: staff should feel empowered to say, "hey, come look at this!" Pre-set procedures, combined with regular conversations with managers, can help RAs identify what must be flagged and reported immediately, versus what must be flagged but is not urgent, versus what should be documented but may not ultimately cause problems. Clear plans and open channels of communication help staff work together to make informed decisions.
Similarly, implementing partners should feel comfortable raising concerns about protocols, whether they are observing changes to protocols in practice, think that protocols should be changed, or both. If possible, discuss ahead of time how to communicate about and design potential changes to implementation protocols if an intervention begins to evolve over time.
Case study: In a case management intervention designed to help low-income students overcome barriers to college completion, researchers observed that differences in outcomes between treatment and control groups narrowed after two years (Evans et al. 2017). Outcomes included enrollment in college, GPA, and degree completion. After one year, outcomes for the control group were starting to look similar to the outcomes for the treatment group. In response to this type of change, researchers can ask program staff to discuss changes together. These conversations should not be framed as “the program not working,” but rather as a request to investigate the program together and explore what has changed.
In this case management example, the study team read case notes and identified less engagement between students and counselors over time as a possible cause of the narrowing difference in outcomes between treatment and control groups. They found that the average length of time that counselors had been spending per session with students had declined starting at the end of the first year. This was not in line with the program’s guidelines and dampened the effect of the intervention.
In order to adjust the intervention towards the program’s desired implementation, the program staff changed the guidance and requirements for counselors, asking counselors to ensure that each session met a specific minimum time requirement. A monitoring plan that flags narrowing outcomes across treatment and control groups, together with thoughtful communication between researchers and implementing partners, can prompt this type of exploration and adjustment.
Set up regular calls and visits with implementing partners
Implementing partners hold essential information about a study’s progress and any changes or challenges in the intervention or study. During the process of choosing indicators and data—and throughout the entire process of study design and implementation—research staff and implementation staff should set up regular calls for communicating updates and concerns.
Regular calls with regular staff to discuss study details, updates, and progress in general, even if problems seem unlikely, mean that there will be opportunities to learn about problems as they occur.4 These regular check-ins help to create routines and establish understanding and trust that will be helpful if problems do arise. They also make it more likely that minor challenges will not worsen. Open and regular communication makes it more likely that issues that may seem minor to some, but are actually major issues, come to the attention of the team. There does not need to be an agenda for each call: the most important thing is to have a regular point of contact.
Site visits, if possible, are another way to both facilitate open communication with partners and actively observe how protocols are being implemented in practice.
Consider designating a “field RA” or embedding a researcher on site
Designating a research associate to engage with implementing partners on the site of the study can be a valuable way to build relationships and open a channel for the research team to be aware of unexpected problems as they arise. A “field RA” may be designated to work on these tasks full time; or fielding activities may be woven into the responsibilities of an RA with more typical office-based, data-focused activities. Goals for a field RA can include both implementation monitoring and learning whether the planned protocols make sense to the partner or should be modified.
A full-time field RA might be required for some projects, while other projects’ field monitoring might be accomplished via more infrequent visits from an RA who has both data and fielding responsibilities. Sending a research associate to a study site for a day-long visit, for example, can allow this member of the research team to learn, in real time, how protocols are being implemented. If a schedule of regular check-ins is set, it’s important to conduct these visits as planned even if no concerns have arisen yet; visits can help build good relationships and cement contingency plans for if concerns do arise.
Appendix: sample monitoring tables
Appendix table A: important yes/no checks
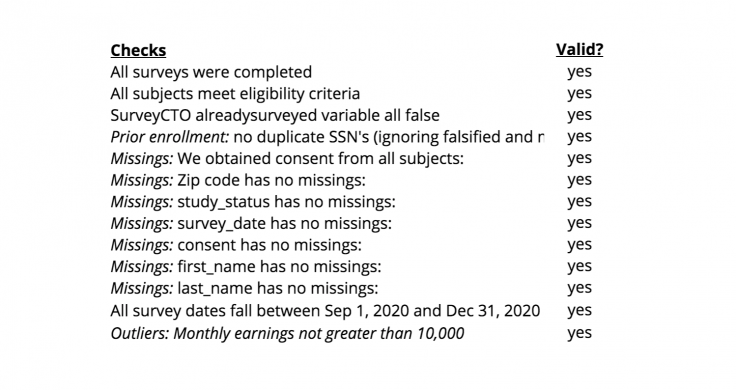
Appendix table B: custom balance checks
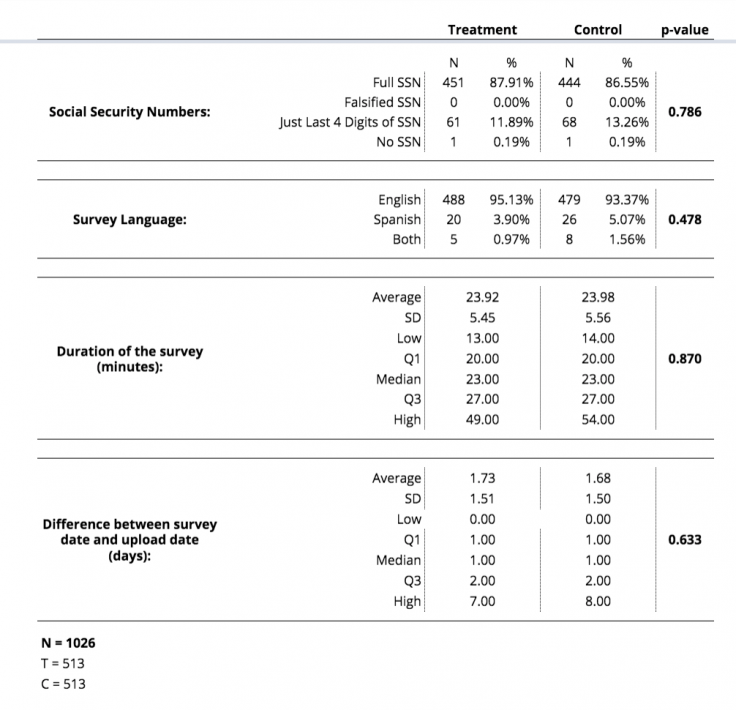
Appendix table C: survey duration
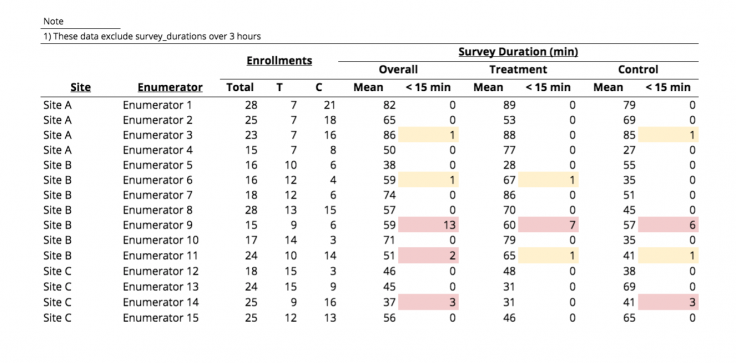
For example, if a survey is designed to take 45 minutes, then it may be helpful to track the number of surveys that are much shorter than expected—in this case, 15 minutes is a threshold for concern. A research team might decide that although short surveys do occasionally happen and a single short survey is not necessarily cause for concern, they want to track whether enumerators tend to frequently administer surveys shorter than 15 minutes. In this example table, one short survey is a “yellow flag.” However, if one enumerator has two or more surveys lasting less than 15 minutes within a certain monitoring period, this generates a “red flag” and may prompt a member of the research team to reach out to the enumerator to ask whether they are encountering difficulties.
Thanks to Mike Gibson, Sam Ayers, Kim Gannon, Sarah Kopper, and Sam Wang for their advice and suggestions. Thanks to Stephanie Lin for compiling case stories of lessons learned from past research engagements, on which the case studies above are based. Caroline Garau copyedited this document. Please send any comments, questions or feedback to [email protected]. This work was made possible by support from Arnold Ventures and the Alfred P. Sloan Foundation. Any errors are our own.
An “indicator” is an observable signal (Glennerster and Takavarasha 2013, 181).
For suggestions on documenting randomization, see this resource on managing files, data, and documentation for randomized evaluations.
Evaluating technology-based interventions includes more examples and recommendations for studying interventions that may be affected by software updates and code changes.
J-PAL North America’s guide on “Implementing Randomized Evaluations in Government: Lessons from the J-PAL State and Local Innovation Initiative” shares discussions and examples to guide communication with partners as part of a monitoring plan (Chabrier et al. 2017, 20-22). For example, it may be helpful to discuss with partners how monitoring during a pilot period can clarify or answer important questions before the start of a study. In a study of the Bridges to Success program in Rochester, NY (a mentor program that aims to help residents in high-poverty neighborhoods of Rochester overcome barriers to self sufficiency), researchers and implementers conducted a pilot period to monitor rates of enrollment in the program. Since enrollment rates were lower than anticipated, the program expanded its eligibility criteria before the full launch of the study in order to fill the number of program spots available (Chabrier et al, 2017, 22-23).
Additional Resources
Evaluating technology-based interventions, J-PAL North America. This resource provides guidance for evaluations that use technology as a key part of the intervention being tested and discusses challenges that J-PAL North America staff have encountered with these types of studies. Examples of these challenges can help readers anticipate ways in which monitoring plans could help detect and avoid technology-related challenges
Formalize research partnership and establish roles and expectations, J-PAL North America. This resource outlines steps to establish and build a strong working relationship with an implementing partner at the beginning of a randomized evaluation. The subsections “Define communications strategy” and “Elements of a communications strategy” may inform choices about building regular communication with partners into a monitoring plan.
High-frequency checks, Innovations for Poverty Action (IPA). These Stata templates are designed for population-based survey research and randomized impact evaluations. This code runs high-frequency monitoring checks of incoming research data. These templates may be customized according to a study’s needs.
Randomization: Balance tests and re-randomization, J-PAL. This resource provides code samples and commands to carry out balance tests and other procedures.
References
Chabrier, Julia, Todd Hall, and Ben Struhl. 2017. “Implementing Randomized Evaluations In Government: Lessons from the J-PAL State and Local Innovation Initiative.” J-PAL North America.
Evans, William N., Melissa S. Kearney, Brendan C. Perry, and James X. Sullivan. 2017. “Increasing Community College Completion Rates among Low-Income Students: Evidence from a Randomized Controlled Trial Evaluation of a Case Management Intervention.” NBER Working Paper. https://www.nber.org/papers/w24150
Glennerster, Rachel and Kudzai Takavarasha. 2013. Running Randomized Evaluations: A Practical Guide. Princeton: Princeton University Press.
J-PAL. 2019. Implementation Monitoring Lecture from 2019 Research Staff Training.