Data cleaning and management
Summary
Although quality control checks in the field should catch most errors in data collection, there are a number of steps you will have to undertake to prepare your collected data for analysis. This resource outlines the key steps involved in data processing and cleaning. We first describe best practices in file and code organization, which will help others (and yourself) easily find the most recent versions of files and understand what was done with the data. The second half of the resource details steps to make the data ready for use. This resource focuses on cleaning data with Stata code, but similar principles apply to R and other data analysis tools.
File organization
Setting up a clear folder structure early on in a study will enable consistent management of data, documents, and other files. Researchers typically will negotiate a data flow with study partners—and in tandem can begin creating a plan for storing and managing data and other files. Files are commonly organized by type: do-files, raw data, cleaned data, and survey instruments. In planning file structure, you should at a minimum do the following:
- Preserve original, de-identified data. See J-PAL's data de-identification resource to learn more.
- Keep data, coding files, and results separate, and include sufficient documentation to make the order and degree of data manipulation clear. Examples of data manipulation include dropping, adding, or editing data.
- It is also useful to keep the steps in data manipulation or analysis separate. For example, keep a separate do-file for data cleaning code (which produces a cleaned dataset from the raw data), a do-file for exploratory analysis code (which is used for trial and error, to play around with data, run regressions, produce summary statistics, and a do-file for final analysis code (which produces estimation results reported into LaTeX tables, properly formatted and annotated graphs, etc.).
- Keep a master do-file, which is a single do-file that calls all relevant do-files (see more below). It should be possible to run the master do-file, cleaning code, analysis code, and code that produces figures separately. You may want to rerun a specific analysis or output for a specific table, and having to run everything together is time-consuming.
- Practice version control, discussed further below, which includes archiving previous versions. This preserves past work, allowing you to return to it.
- Consider the data flow of the project (e.g., which individuals should have access to what data, how data will be stored/backed up, and how datasets will interact) when creating a folder structure.
An example of good file structure is as follows:
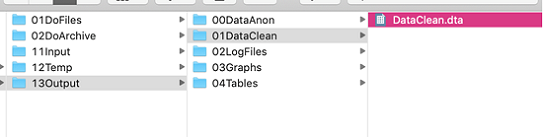
- The DoFiles folder contains do-files organized by task:
- The DataPrep folder contains do-files to import the data to Stata and de-identify it, as well as do-files to clean it to the version used in analysis.
- The DataAnalysis folder contains do-files that 1) run summary statistics, 2) analyze the data without producing formatted output (which is slow), and 3) produce nice LaTeX tables. Keeping these separate helps you rerun the analysis code quickly, as code to produce formatted tables can take a while to run.
- The DoArchive keeps archived and dated do-file folders. Archiving the entire DoFiles folder preserves the underlying structure of the do-files that worked together:
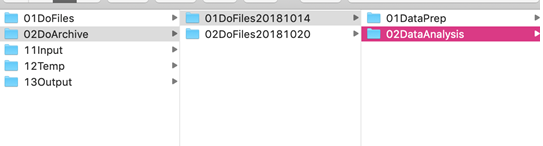
- The Input folder contains data that is being imported and should typically not be modified in any way. Within the folder, there are two subfolders:
- DataRaw should contain the encrypted file containing identified data (or data with information that could be used to identify individuals) or no data. For more information on data encryption, see our Veracrypt tutorial, the Data security procedures for researchers resource. J-PAL staff and affiliates: see also J-PAL's Data Security RST lecture.
- DataCorrections should contain any corrections that are made based on identified data, such as a GPS code that is replaced. These corrections should be merged in from an encrypted file as well, since they contain identifiers. Do not write them into do-file. Your workflow should be:
- Import data from DataRaw
- Import any corrections based on subject ID from DataCorrections
- Remove the identifying variables and generate a randomly assigned unique ID number following the procedure described in Data publication.
- The Temp folder contains any temporary files and should be cleaned out before analysis starts. Note: to avoid unnecessary clutter or “junk” files (many files that are used only once and then never again), it is useful to create temporary files that are erased after the program has run. In Stata, it is easiest to create a file in the Temp folder and, at the end of the do-file, use the command erase(tempfile) to delete the file. You can also use a local to create a tempfile. This has the benefit of automatically being erased after the code has been run (so you do not need to use the erase command) but can be unwieldy if you are running only snippets of code at a time, as it is a local and thus only works for the selected code sequence. The syntax to create a local tempfile is:
tempfile tempfilename
save `tempfilename'
/* this can then be used as any other dataset,
e.g.,can be merged, appended, etc. */
In some cases, you may inherit a disorganized or unclear folder structure. If you do not choose to reorganize it, which is time-consuming, you can create a folder map, which is an Excel or Word (or similar) document that lists key folders and their contents. Consider creating a template folder structure to use across projects, or across rounds of surveys within a project. IPA also has created template folder structures. See also the template folder structure linked as a related download in the sidebar.
Code organization
Code should be organized to help the reader understand what was done, which is useful both in continuing analysis and in writing up methods for a paper. Code files can get long, and well-documented code saves the user time by limiting repetitive work, errors, missed steps, and unnecessary re-testing. There are several practices you can incorporate to make your code easy to understand.
Header and footer
Start with a well-organized, informative header. The header of your do-file should contain the following:
- The project, who wrote the do-file, and when
- The purpose of the do-file
- The date the do-file was created
- Version of software
You should then set the environment:
- clear all (the command clear will only drop variables and clears labels. It won’t clear macros, stored estimates, programs, scalars etc. clear all clears everything, as the command implies)
- Set the Stata version for upward compatibility. That is, setting the version to Stata 14 will allow Stata 15 users to do exactly what you did. Setting the version to Stata 15 means that Stata 14 users will not be able to run your code. In Stata, this is done with version (version number)
- Set the seed to make randomization replicable. This must be combined with versioning (the bullet point above) to make randomization stable. In Stata, this is done with set seed (seed). See Stata's help documentation for information on how to choose the seed
- The command set more off will tell the code to run continuously, rather than Stata pausing and asking if you’d like to see more output. set more off, permanently will save this preference for future programs (set more on will turn this option back on).
- Force a log to close if one is currently opened
- A log is a full record of your Stata session, including output (excluding graphs) and commands embedded into the output. At the start of your do-file (not the header), start the log with the command log using
- Assign memory if more is needed (via set mem (memory))
* Author, date
* File loads raw data and carries out subroutine.do
* Written in Stata 14 on a Mac
clear all
/* clear memory
("clear" alone only clears variables and labels) */
version 14.2
/* later versions will do exactly what you did */
cap log close
/* closes any open log files; "capture"=no output,
even if the command fails (i.e., no open log)*/
set more off
/* running long code is not interrupted with
--- more ---. You set more off permanently
using set more off, perm */
set mem 100m
* assign additional memory if needed
set seed 20190802
/* doing anything random? set a seed so it's replicable! Make sure
you specify the Stata version or else your seed is not stable */
* Set working directory:
cd "/Users/Anon/Google Drive/Research/FileStructureExample"
/* Write one of those for each co-author and comment
out those you don't need. See the box below for alternative
methods You can use relative path names for everything in
the working directory. */
- Importantly, set a working directory or file path that differs between co-authors. From then on, use relative file paths. An absolute file path uses the entire reference (e.g., C:/Users/username/Dropbox/folder1/folder2), while a relative file path involves telling Stata that you are in some working directory (e.g., C:/Users/username/Dropbox/folder1) so that subsequent file paths can be the same across users. Using a relative reference/file path means that each co-author can comment out or change the file path of a different co-author in the header, without having to change every single file path throughout the do-file. It is useful for similar reasons if the file path changes, such as if you archive the folder.
- Note: While Windows, Linux, and Mac will recognize file paths using "/", Mac and Linux computers may not recognize "\". Therefore it is a best practice to use "/" in file paths regardless of your operating software.
In Stata, you can set up a relative reference by either defining a global or changing the working directory:
* Option 1: Using globals:
global user = "Name1"
*global user = "Name2"
// Comment out the names of other users
/* Note: globals are set permanently (until cleared). Locals
are only set for the duration of the current code sequence*/
if "$user" == "Name1" {
global path “C:/Users/user1/Dropbox/mainfolder/13Output”}
if "$user" == "Name2" {
global path “C:/Users/user2/Dropbox/mainfolder/13Output”}
* You can then use relative file paths, such as:
use "$path/13Output/01DataClean/Dataclean.dta", clear
* Option 2: Changing the working directory:
capture cd "C:/Users\user1/Dropbox/mainprojectfolder"
capture cd "C:/Users\user2/Dropbox/mainprojectfolder"
/* Note: if the command fails, using capture will ignore
the failure so that Stata continues on to the next line */
global data "data"
global output "output"
* You can then use relative file paths, such as:
use "13Output/01DataClean/DataClean.dta", clear
Note: Option 1 (using globals to set file paths) from the above example may be more robust to differences in coding styles between authors.
At the end of your do-file, the command capture log close will close and save your log file. Again, using the command capture means that Stata will continue through, even if there is an error in the command (e.g., there is no open log) and will suppress output from that command. exit will stop the do-file from running, and exit, clear will let you exit, even if the current dataset has not been saved.
Body and commenting
To keep do-files from getting too unwieldy, organize code into sections, each with a comment header (see below). You should also clearly label each discrete task, such as renaming variables or running different regressions. Comments should focus on how and why you have made certain decisions.
There are two common approaches to commenting while coding:
- More-is-better: In this approach, you should err on the side of making more comments than necessary, bearing in mind that decisions that may seem obvious to you now may not seem obvious to others (or to yourself in the future). That said, you should be careful to ensure the code and corresponding comment are consistent, i.e., if you update a certain line or lines of code, make sure the comment is updated accordingly.
- Self-documenting: In this approach, the goal is to reduce the need for comments. Code is “self-documenting” if variables, functions, macros, and files are named descriptively, clearly, and consistently and the code is structured to guide readers. To supplement descriptive naming practices and structures, version control software or comments within code can document why decisions were made—for example, why a certain type of outlier is dropped or why the code uses one command versus another (Pollock et al., 7). Some teams may decide to format comments in a standard way for consistency and clarity; some teams may choose an entirely self-documenting system for documentation that uses no comments at all. While it takes time and effort to learn any system for writing self-documenting code, the goal of such a system is to avoid errors arising from outdated comments and to reduce the maintenance costs of time and effort required to keep comments up to date (Gentzkow and Shapiro, 2014, 28).
In both approaches, it is useful to create comment markers at important events or certain types of decisions, such as creating or exporting a table, graph, or chart; merging and appending datasets; reshaping and collapsing; dropping duplicates; and changing a unique ID. If you use the same marker for the same type of event (e.g., generating a new variable, top-coding a variable, replacing values, etc.), you can later easily search the do-file for that term. Examples of comment markers are /!\ and decision. Define the markers used in the first section of the do-file, after the header.
In Stata, there are 4 important ways of breaking up code and adding comments:
- * is used for full-line comments. Use this to create section dividers
- // is used for in-line comments. Use this for markers or short comments
- /*...*/ is used for block comments. Use this for longer comments, but keep the line length reasonable (to 80-100 characters)
- /// is used for line breaks
*****************************************************
*** Beautiful comments ***
*****************************************************
*If the line starts with * the whole line is a comment
// Short comments can be stored in an in-line comment:
sum var1, detail // in-line comment example
* You can break long commands over several lines with ///
/* Example: a graph with 3 lines for
control, HW, and FC groups: */
graph twoway ///
line nlnlScont _t if _t<=$ndays+5, sort lw(thick)||///
line nlnlShw _t if _t<=$ndays, sort lp(shortdash)||///
line nlnlSfc _t if _t<=$ndays, sort lp(longdash) ||///
leg(label(1 "Control") label(2 "Hw") label(3 "FC"))
/* Longer comments can go in a comment box,
enclosed by /* and */. /*
This is an example of a longer comment. */
/* Comment markers used in this do-file:
/!\ signifies an issue that needs further attention
// decision signifies a decision, such as every
time the data was manipulated during data cleaning */
** Comment markers in action:
isid uniqueid // /!\ id variable doesn't
// uniquely identify observations
duplicates report
duplicates drop // decision: dropped duplicates
Pseudocode
Pseudocode is an outline of the algorithm you plan to use written out in a mixture of plain English and code. It allows you to think about high-level design and the pros and cons of various approaches to what you want to do before looking up specific programming details. In a long program, is easier to change a couple of lines of pseudocode than real code (which can require significant debugging), so it is worth putting in some time on pseudocode upfront. For more information, see Pseudocode 101 from MITx's Introduction to Computer Science and Programming Using Python course.
Pseudocode is also useful for documentation; rather than adding comments to code after it is written, which can lead to conflicts if the code is updated and is no longer consistent with the comment, the thought process is already documented in the pseudocode. Note that when using loops (as in below example) you can use the command set trace on to determine which lines of code work.
*** Code in published .do file:
* Has all the information but is hard to fully understand.
local datetime_fields "submissiondate starttime endtime"
// format date time variables for Stata
if "`datetime_fields'" ~= "" {
foreach dtvar in `datetime_fields' {
tempvar tempdtvar
rename `dtvar' `tempdtvar'
gen double `dtvar'=.
cap replace`dtvar'=clock(`tempdtvar',"MDYhms",2025)
drop `tempdtvar'
}
}
You could write the following:
*** The same algorithm in pseudo code:
// Start with an auto-generated list of variables
// containing time and date information
// if list not empty:
{
// for each date-time variable in the list:
{
// save the original date-time variable "dtvar"
// under the temporary name "tempdtvar"
// create an empty variable "dtvar"
// decode the temporary variable
// and format it as a time-date field
// drop the temporary variable
}
}
Once you know what you want the algorithm to do, you can fill in the pseudocode with real code:
*** Filling the pseudo code step by step with real code:
// Start with an auto-generated list of variables
// containing time and date information
local datetime_fields "submissiondate ///
starttime endtime"
// if list not empty:
if "`datetime_fields'" ~= "" {
// for each date-time variable in the list:
foreach dtvar in `datetime_fields' {
// save the original date-time variable "dtvar"
// under the temporary name "tempdtvar"
tempvar tempdtvar
rename `dtvar' `tempdtvar'
// create an empty variable "dtvar"
gen double `dtvar'=.
// decode the temporary variable
// and format it as a time-date field
cap replace`dtvar'=clock(`tempdtvar',"MDYhms",2025)
// drop the temporary variable
drop `tempdtvar'
}
}
Master do-file
A master do-file is one that calls all of the different steps of the analysis in order and runs them in one go. It’s useful to include when you want to run everything, such as if others want to check the replicability of your code or want to check results before submitting the paper. It can be time-consuming to run through everything, however, so it is useful to write code so that the individual files also run by themselves. Within the master do-file, call each program using the command do (do-file name).
In order for the master do-file to work, the file references in both the master do-file and the individual files must be consistent. You should also list in the master do-file any user-written commands (i.e., Stata commands written by Stata users that are not part of Stata's default offerings) that are used in the code, along with the code that installs them (these should also go in the readme). A sample master do-file can be found below--note that the screenshot excludes some header contents, though these should be included in the master do-file as with any other do-file.
* Set working directory:
cd "/Users/Anon/Google Drive/Research/FileStructureExample"
/* If you may need to use files that are not in the working
directory, you may want to specify the path name upfront: */
global datapath "/Users/Anon/Google Drive/Research/FileStructureExample/13Output/0DataAnon"
global dopath "/Users/Anon/Google Drive/Research/FileStructureExample/2DoArchive/1DoFiles20180114"
// clear out the temporary directory
cd "./11Temp"
local tempfilelist : dir . files "*.dta"
foreach f of local tempfilelist {
erase "`f'"
}
cd "../"
log using "./13Output/2Logfiles/ProjectNameLog.txt",replace
// Import data and clean it
do "${dopath}/1DataPrep/Clean.do"
// Run regressions
do "${dopath}/2DataAnalysis/2Analysis.do"
// Make LaTeX tables and graphs for the paper
do "${dopath}/2DataAnalysis/3LaTeXandGraphs.do"
/* Note! $datapath is used in the individual do-files! Make sure
your use of global macros vs. relative file paths is consistent
everywhere */
Documentation and version control
Documentation and version control are critical components of any project. Documentation tracks your thought process, letting others (including your future self) understand why certain decisions were made. This both helps others understand your results and improves the replicability of your work--and, thus, the integrity of your research. During data cleaning and analysis, it is easiest to document mechanical coding decisions directly in the code using pseudocode or clear comments. Bigger decisions such as the imputation of variables or dropping outliers should also be documented in a separate file and later included in the paper. Task management systems (such as Asana, Wrike, Flow, Trello, and Slack) can document tasks, decisions, and other work that occurs outside of coding. This information can later be written up in a readme file, the related paper, or in other documentation.
Version control lets you preserve earlier versions of files, meaning that no change is irreversible (an example of an irreversible change is when you save a change and then close the file, you can’t easily undo the change). This is especially important when multiple revisions are made to a file--which will be the case during data cleaning. Your two most common choices for version control are doing so manually or using Git and Github. Dropbox offers limited version control capabilities but only stores previous file versions for a finite amount of time.
For manual version control, as written above, archiving and dating the entire do-file folder together preserves the structure that worked together. Keep the current version of each file in your working directory and date each version using the format YYYY_MM_DD or YYYYMMDD - this lets you sort files by date. Do not append file names with initials, as this quickly gets confusing. That is, use file names such as cleaningcode_20190125, rather than cleaningcode1_SK.
An alternative is to use Git and GitHub, or SVN. Git has a bit of a learning curve but has distinct advantages over manual version control. For example, multiple users can work on the same file at once and ultimately sync the changes, and the changes themselves are documented, so it’s easy to see which change was made from one version to the next. This makes Git particularly useful for projects involving many people collaborating on the same files. Useful resources for getting started with Git include the Udacity Git/GitHub course, and GitHub help. J-PAL staff and affiliates: see also J-PAL's Research Staff Training presentation on the subject.
Regardless of your choice of manual version control versus using Git/Github/SVN, for data cleaning, the two most important points to always remember are:
- Document any and all changes made to the raw data. This can easily be done in the cleaning do-file using comments and the code used to make the changes. Changes made in Excel or a similar type of program will not be documented, which is why it is important to do everything in Stata, R, or similar.
- Never overwrite the raw data file. To help prevent this, save cleaned data files in a different folder, as described above.
Data cleaning
Introduction
Data cleaning is an important precursor to doing any analysis. Even with careful surveying, there may be typos or other errors made in data collection, or there may be outliers that, if not properly accounted for, could skew your results. Before doing any kind of analysis, it is important to first clean the data, regardless of whether it is original field data or administrative data. Cleaning data can be time-consuming, but putting in the effort upfront can save a lot of time and energy down the line. Note: the first step in data processing should always be to encrypt data that contains information that could be used to identify individuals.
Throughout the data cleaning process, the two most important points to keep in mind are:
- Document decisions
- Never overwrite the original/raw data file
By documenting all data cleaning tasks and preserving the original data file, any mistakes can be undone, and the steps taken to clean the data can be recreated. This is especially important for research transparency and robustness checks. For example, researchers can test the stability of results based on different cleaning/trimming criteria (e.g., winsorizing or dropping observations above the 95th vs. 99th percentile of the distribution). Data cleaning should be done on de-identified data (as identified data will not be used in analysis anyway). For more information on encrypting identified data, see Data security procedures for researchers. J-PAL staff and affiliates: see also J-PAL's RST lecture on data security. For more information on de-identifying data, see the de-identification resource.
Steps
1. Set up your file
Follow the steps above: set up a header that clears the environment, sets the working directory, seed, and version, and includes information on project name, co-authors, purpose of the do-file, date of creation, etc.
2. Import and merge your data
- In your do-file, import and merge files as needed. Doing this in your do-file means that the import is documented: someone else (or your future self) can just run the do-file and know exactly which raw data file is imported.
- In Stata, use merge 1:1 when each observation in the master data file matches a single observation in the using file. If not, use merge m:1 or merge 1:m (not merge m:m - see why on the last slide of Kluender & Marx's IAP Stata workshop slides.
- Check: Did the files merge correctly? Check a couple of specific observations to be sure.
- Check: Which observations did not match? (In Stata: tab _merge)
3. Understand your data
- Use the browse window to look at your data
- Look at the distribution of every variable (tab; kdensity; sum var, detail). Are there any obvious issues? These could include:
- Typos (e.g., someone 500 years old; other data entry problems, e.g. with units)
- Outliers, both plausible and implausible values
- Logical errors such as:
- Someone working 1,000 hours in the past week
- Children older than their parents
- Time-invariant variables not being time-invariant (e.g., district in which household is located)
- Check whether means are the same over time: tab year, sum(timeinvariant_var)
- Check also for “other” entries in your categorical variables (see more below)
- Check for duplicates in unique IDs (in Stata: isid id_variable will tell you whether the variable id_variable uniquely identifies observations. Other useful commands include duplicates report, duplicates list, etc.).
- Note: This should ideally be done during data collection so that field staff can be consulted to determine whether the duplicates are due to some incorrectly assigned IDs or double submissions
- Missing values: Check the number of observations for every single variable. In Stata, the command tab variable, missing will tell you the share of missing observations for a given variable
- Is there any obvious censoring or truncation? For example, a large number of households having exactly 6 household members listed and none with 7. This can also happen with variables such as income, assets, and age, and with variables where the respondent is asked to list out everything in that category and answer questions about each individual listing (such as a roster or census of a household’s agricultural fields, where the respondent is then asked questions about each individual field)
- Check the variable types of each variable (which can be done via the describe command). Are numerical variables coded as string variables? See below for more on dealing with strings
- Make sure times and dates are coded consistently
- Check that your data is in the appropriate format (wide vs. long) for your analysis. In a wide format, a single unique identifier (such as household ID) will have a different value for each observation (i.e., uniquely identifying it), while in a long format a combination of identifiers (such as household ID and survey wave) will be needed to uniquely identify each observation. You can use reshape in Stata to convert your data from wide to long or long to wide
Make decisions
Obvious errors
- Cross-check and use as much information as you have to fix the errors. For example, for a pregnant male, you can check the individual’s relationship to the household head, as well as the composition of the rest of the household, to determine whether the pregnant male is actually a pregnant female, or a not-pregnant male.
- If you have a baseline/endline survey, or panel data, use linked observations from other survey waves to cross-check data (e.g., match individual IDs from other survey waves to determine the person’s gender).
- If you are unable to determine the correct value (e.g., whether the pregnant male is in fact a pregnant female or a not-pregnant male), replace the incorrect value with a missing value. Use Stata’s extended missing values to code the reason for the value being missing (e.g., .n=not applicable; see also Innovations for Poverty Action (IPA)'s suggested standards for extended missing values).
Outliers
Unless an observation is an obvious error (and it is obvious what the value was meant to be), do not try to guess what it should be.
- Note: Outliers can be dropped in analysis--this could mean dropping (or winsorizing, which means replacing all values over or under a given percentile such as the 99th or 1st percentiles with the value at that percentile) observations over the 99th percentile or below the 1st percentile, for example. When cleaning data, it is generally better to not drop outliers (though you may want to when analyzing the data). For example, research partners or others may wish to use the dataset for other analyses and may treat outliers differently. Discuss how to approach outliers with all others who will conduct data analysis.
- If you drop outliers from analysis (rather than winsorize), use Stata’s extended missing values, as described below, to indicate why the new value is missing.
Missing values
- Ideally, “true” missing values, such as if the subject stopped the survey, are caught during data collection so enumerators can follow up. If missing values are caught at a point when it is not possible to collect more data, you should, at minimum, recode the missing values to indicate the reason for missingness (if known).
- If missing values were coded as -999, -998, and so on in the survey, changing the codes to Stata’s extended missing values (.a, .b, .d, .r, etc.) is useful because it means that the missing values won’t skew the distribution if someone forgets to check for them.
- Having a different missing value associated with each reason also preserves information. Standard codes include: .d=don’t know, .r=refusal, .n=N/A. You can also recode for “dropped during cleaning”. We recommend following IPA's standard missing values. Following these guidelines, the standard missing value in Stata (.) should be reserved for true missing values. You may also want to give coding errors or surveyor errors, such as if the respondent gave an answer that was mistyped by the surveyor (such as for GPS or billing codes, ID numbers, etc.)
- In Stata, recoding values from a SurveyCTO form can be done with the command mvdecode:
mvdecode variable, mv(-999=.d \-998=.r \-997=.d)
- Doing nothing else beyond recoding your missing data may be a reasonable choice if the missing values occur at random, but as this is often not the case, this could bias your results. Discuss how to approach missing data with all others who will conduct data analysis. A list of things to consider when deciding how to respond to missing data can be found in this post from Marc Bellemare's 'Metrics Monday Blog.
- If you choose to take further action to address missing values, there are several options including:
- Imputing missing values: If you do impute values, clearly document in the code the method of imputation. Some methods for imputing missing values include, but are not limited to:
- An alternative to imputation is aggregation. For example, if you are interested in land price data but are missing land prices for some villages in a district, use the district-level mean or median in place of village-level prices for missing observations.
- An instrumental variables approach, if you have a plausible instrument for the missing variable (this is unlikely).
Dealing with string variables
When programming your survey, limit use of strings as much as possible (for example, use codes and value labels for districts, rather than a string variable with the district written out). This will save enumerators time and reduce the number of errors made in data entry (e.g., by mistyping the word “male” as “mael”). The string functions section of the Stata manual has a number of useful commands, and IFPRI has a helpful guide that covers string manipulation. Some particularly useful Stata commands to help clean string variables include:
- Convert strings to numeric values using encode, which will automatically label the numeric variable (though the values will be assigned in alphabetical order, e.g., 1=Allston, 2=Brighton, 3=Cambridge, 4=Somerville, regardless of whether your data is in that order). With encode, you should generate a new variable (i.e., encode stringvar1, gen(numericvar1))
- The command destring will also convert strings to numeric variables if the string consists entirely of numbers, but it will not label the new values. With destring, you can replace the string variable with a numeric variable, i.e., destring var1, replace
- Replace all of the characters in the values of the string variable in either upper or lower case using replace(variable)=upper(variable) or replace(variable)=lower(variable)
- Trim spaces at the beginning or end of the values in the string variable (e.g., replace “ Male “ with “Male”) using trim(stringvar). The syntax is replace stringvar=strtrim(stringvar).
- Create dummy variables for different string values with the command tab var1, gen(newvar). This will create a new dummy for each value of var1. For example, if you have the variable cityname, with values Cambridge, Somerville, Allston, and you write tab cityname, gen(city), you will get 3 new numeric variables (city1, city2, city3). City1 will equal 1 for households in Allston and 0 otherwise, city2 will equal 1 for households in Cambridge and 0 otherwise, and so forth. Note that the dummies are created in alphabetical order.
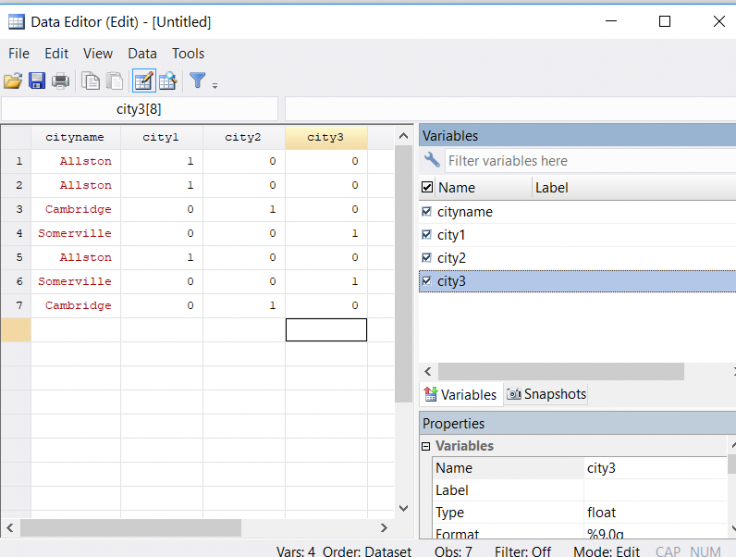
- Replace characters in the values of the string variable using the subinstr command (with parameters s, n1, n2, & n3), where s is a string or a variable name, n1 is what you would like replaced, n2 what you would like n1 replaced with, and n3 the number of instances in which you would like it replaced (use a period(.) in n3 to replace all instances of n1). Note that if you do not use a period in n3 , it will replace the first n3 instances of n1, so if for some reason you are not replacing all instances, be sure to sort your data first for replicability.
- You can also use subinstr to either generate new string variables or replace a string variable
- Stata's sub-string function (with parameters s, n1, & n2) is used to extract characters in string s starting at position n1 and continuing for n2 characters. For example, if you had a variable called doctor_name, and rather than write the doctor’s last name enumerators filled in “Dr. Name1”, “Dr. Name2”, etc. you could substitute a "." for the characters starting in position 1 and continuing for 4 characters to produce a new string with just "Name1", "Name2", etc..
“Others” in categorical variables
Even with extensive questionnaire piloting, you will have instances where the options included in a categorical variable are not exhaustive, and respondents choose “other” (where a new string variable for the “other” responses for that particular variable is then created). For example, a household roster that includes the relationship to the household head may not include great grandparents, who then appear in the survey, or respondents may report maize sales in non-standard units that you did not include (e.g., 5 liter buckets). In these cases, you should:
- Try to standardize the “other” options as much as possible using string manipulation tools
- If the same “other” answer appears in many instances (for example, many households included a great grandparent in their roster), consider adding a new value to the main categorical variable (e.g., relationship to household head) for this “other” option. If you do so, be sure to document this by updating the variable’s value labels, the questionnaire and codebook, and your data cleaning comments.
- To update the value labels, use the command label define…, add. For example, label define relationship 7 “great grandparent”, add will add great grandparent to the set of relationship labels you defined in an earlier step
- This will be useful if you want to use the variable in analysis, as you cannot use strings in a regression. For example, you may want to look at intergenerational intrahousehold allocation and either want to pool great grandparents with grandparents or have enough great grandparents to look at them separately.
Final important point
Document any judgment calls you make extensively, and keep them reversible - possibly create a new variable. For example, HH_income_annual vs. HH_income_annual_corrected.
Labels
- Name variables in self-explanatory ways and label them. Example: don’t use var150b if you can use HH_Income_annual
- You can save yourself time by choosing SurveyCTO variable names and labels to facilitate this:
- Under the survey tab, variable names go in the name column (see image 1 below) and the survey programming resource for more resources on programming in SurveyCTO.
- Use the rename variable command in Stata as needed
- Use prefixes for related variables (e.g., hh_varname for all household-level variables, dist_varname for district-level ones, etc.). This naming scheme is useful for quickly writing loops or summarizing variables (summarize hh_* will summarize all household-level variables, for instance).
- You can save yourself time by choosing SurveyCTO variable names and labels to facilitate this:
- All variables in the clean dataset should have variable labels to describe them. For example, label variable HH_Income_annual “Household’s annual income (KSH)”
- In SurveyCTO, this is done in the survey and choices tabs (images 1 and 2 below, respectively)
- In the survey tab, the type should be select_one, followed by the label name
- In the choices tab, the value name goes in the list_name column. Numeric values for the response options go under the value column, and the corresponding labels go under the label column.
- In SurveyCTO, this is done in the survey and choices tabs (images 1 and 2 below, respectively)
- Categorical variables: Label all possible categories/values of the variable
- For binary variables, (re)code so that 1 “affirms” the variable (e.g., 1=Yes, 0=No). Sometimes, “no” is coded as 2, which can present problems in analysis (e.g., reg outcome treatment - the coefficient on treatment is not easily interpreted if 1=treatment group and 2=control group). Avoid these problems by recoding “no” as 0 during data cleaning.
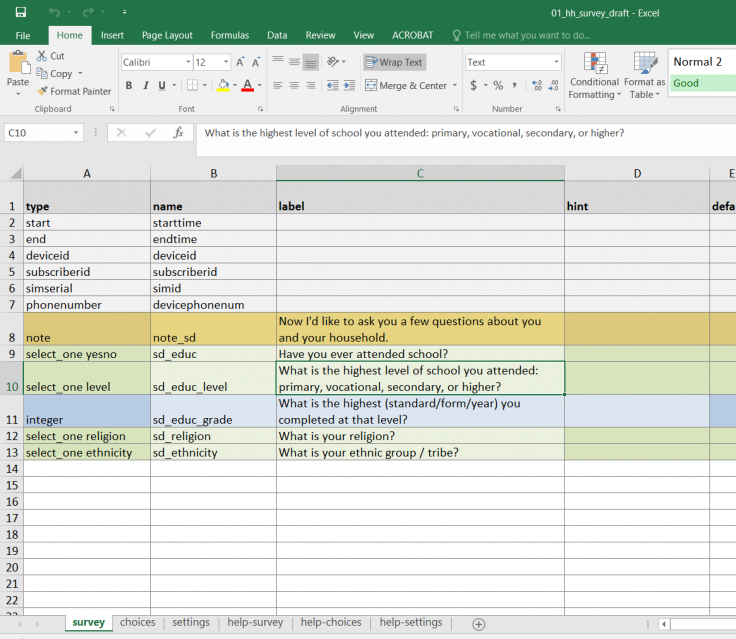
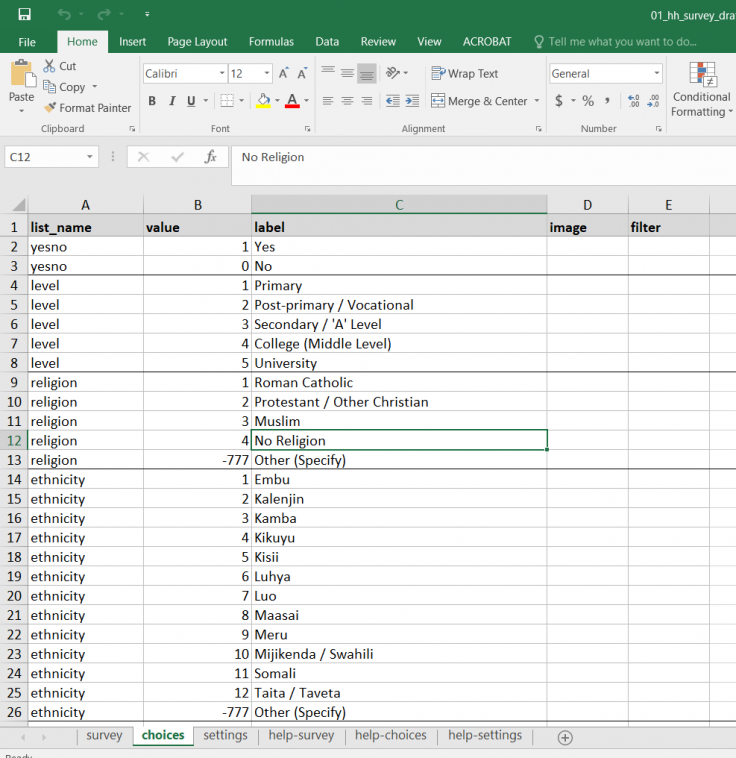
Last updated March 2021.
These resources are a collaborative effort. If you notice a bug or have a suggestion for additional content, please fill out this form.
We thank Lars Vilhuber, Maya Duru, Jack Cavanagh, Aileen Devlin, Louise Geraghty, Sam Ayers, and Rose Burnam for helpful comments and Chloe Lesieur for copyediting assistance. Any errors are our own.
Additional Resources
Programming with Stata, by Raymond Kluender and Benjamin Marx (2016).
Stata Manual: Coding times and dates
Stata Manual: Duplicates
Stata Manual: Erase a disk file
Stata Manual: Logging your session
Stata Manual: mvencode
Stata Manual: Reshaping data
Stata Manual: Set seed
Stata Manual: String functions
Stata Manual: Trace
UCLA's Stata Learning Modules: Reshaping data wide to long
UCLA's Stata Learning Modules: Reshaping data long to wide
UCLA's Stata Learning Modules: Multiple imputation
Dropbox: File Version History Overview
GitHub Help: Set Up Git
J-PAL's Introduction to GitHub RST Lecture (J-PAL Internal Resource)
Open Office: Subversion Basics SVN
Udacity's Version Control with Git course
MIT Data Management Services‘s Managing your data – Project Start & End Checklists
University of Minnesota Libraries’ Creating a Data Management Plan
IPA's Cleaning guide
IPA's Reproducible research: Best practices for data and code management
IPA's Standard missing values (see the link on page 6)
J-PAL's Coding Best Practices RST Lecture (J-PAL internal resource)
Julian Reif's Stata coding guide
Marc Bellemare's 'Metrics Monday blog: Data cleaning
Marc Bellemare's 'Metrics Monday blog: What to do with missing data
MIT School of Engineering: Can a computer generate a truly random number?
MITx 6.00.1 (Introduction to Computer Science and Programming Using Python)'s Pseudocode 101 resource.
The World Bank DIME Wiki: Data cleaning
The World Bank DIME Wiki: Data cleaning checklist
References
Gentzkow, Matthew and Jesse M. Shapiro. 2014. Code and Data for the Social Sciences: A Practitioner’s Guide. University of Chicago mimeo, http://faculty.chicagobooth.edu/matthew.gentzkow/research/CodeAndData.pdf, last updated January 2014.