Understanding the hidden factors influencing child marriage: Lessons from an impact evaluation
Designing intake and consent processes in health care contexts
Overview
When conducting randomized evaluations of interventions with human subjects, researchers have to consider how to design intake and consent processes for study participants. Researchers must ensure that participants are well informed about a study in order for them to give informed consent. This part of the enrollment process will ultimately determine the study sample composition. Health care interventions present additional challenges compared to other human subject research areas, given the complexities of the patient-health professional relationship, concerns about disruptions to engagement in care, and the vulnerable position patients are potentially in, particularly if enrolled while receiving or seeking care.
This resource details challenges in health care contexts when designing the intake and consent process and highlights how challenges were addressed in two studies conducted by J-PAL North America: Health Care Hotspotting in the United States and the Impact of a Nurse Home Visiting Program on Maternal and Early Childhood Outcomes in the United States. These case studies show the importance of communicating with implementing partners, deciding how to approach program participants, supporting enrollment, providing training, and creating context-specific solutions. For a general overview of the intake and consent process beyond the health care context please see the resource on defining intake and consent processes.
Health Care Hotspotting
Description of the study
The Camden Coalition of Healthcare Providers and J-PAL affiliated researchers collaborated on a randomized evaluation of the Camden Core Model, a care management program serving “super-utilizers,” individuals with very high use of the health care system and with complex medical and social needs, who account for a disproportionately large share of health care costs. This program provides intensive, time-limited clinical and social assistance to patients in the months after hospital discharge with the goal of improving health and reducing hospital use among some of the least healthy and most vulnerable adults. Assistance includes coordinating follow-up care, managing medication, connecting patients to social services, and coaching on disease-specific self care. The study was conducted to evaluate the ability of the Camden Core Model program to reduce future hospitalizations amongst super-utilizers compared to usual care.
Patients were enrolled in the study while hospitalized. Prior to enrollment, patients were first identified as potentially eligible by the Camden Coalition’s triage team, which reviewed electronic medical records of admitted patients daily to see if they met inclusion criteria. Camden Coalition recruiters then approached potentially-eligible patients at the bedside, confirmed eligibility, administered the informed consent process and a baseline survey, and revealed random assignment. Potential participants were given a paper copy of the consent form, which was available in English and Spanish, and were given time to ask questions after the information had been provided to them. Enrollment ran from June 2, 2014 through September 13, 2017.
Enrollment at a glance:
- Enrollment period: 3.3 years (June 2, 2014 to September 13, 2017)
- Sample size: 800 hospitalized patients with medically and socially complex conditions, all with at least one additional hospitalization in the preceding 6 months
- Subjects contacted: 1,442 eligible patients were identified, many of whom declined to participate or could not be reached prior to discharge
- Who enrolled: Camden Coalition recruitment staff (distinct from direct providers of health care and social services)
- Where enrolled: Hospital, while subject was admitted
- When enrolled: Enrolled prior to random assignment; randomization revealed immediately after
- Level of risk: Minimal risk
- Enrollment compensation: $20 gift card
Which activities required consent?
Participants provided informed consent for study participation, for researchers to access data, and to receive the intervention if assigned to the intervention. The consent process included informing participants about the randomized study design, the details of the Camden Core Model program and the care they would receive, and how their data would be used. Participants were only able to receive services from the Camden Coalition if they agreed to be part of the study, but they were informed about their rights to seek alternative care regardless of randomization.
The consent process focused on consent to permit the use and disclosure of health care data, known as protected health information (PHI). As explained in the resource on data access under HIPAA, accessing PHI for research often requires individual authorization from patients, and individual authorization can smooth negotiations with data providers even when not required. The process for individual authorization under HIPAA can be combined with informed consent for research. In this case, the study relied on administrative data from several sources: hospital discharge data provided by Camden-area hospitals and the state of New Jersey, Medicaid claims data, social service data provided by the state, and mortality data provided by the federal government. The risk of accidental data disclosure comprised the study’s primary risk.
The consent process provided details so patients could make informed decisions about data disclosure. Patients were informed that the researchers would collect identifiers (name, address, social security number, and medical ID numbers) and use them to link participants to administrative data. Patients were informed about the types of outcomes considered, the types of data sources, the legal environment and HIPAA protections afforded to participants, and how data would be stored and protected. Plans for using administrative data were not all finalized at the time of enrollment so language was kept broad to allow for linkages to additional datasets. The consent form also made clear that the data existed whether patients participated in the study or not; consent was sought so that researchers could access the existing data to measure the impact of the Camden Core Model.
In addition to informed consent for health data disclosure, researchers also obtained informed consent for participating in the study and receiving the intervention. Participants were informed about the parameters of the study (including the goals of the research, that participation is voluntary and does not affect treatment at the hospital, and the probability of selection for receiving the intervention) as well as details of the program (including the composition of the care team and the goals and activities of the program, such as conducting home visits and scheduling and accompanying patients to medical appointments). Because the intervention was not created by the researchers, was unchanged for the purposes of the study, and came with no additional risks beyond the risk of data disclosure, the bulk of the consent process focused on data. Participants selected into the intervention group filled out an additional consent form in order to receive the Camden Core Model program.
A waiver of informed consent was not pursued by the research team, despite the focus on secondary data, which may have qualified the study for a waiver. Waivers are not allowed when it is practicable to seek consent. There was a previously existing in-person intake process for Camden Core Model program participants, so seeking consent was practicable, and therefore sought by the research team.
Seeking informed consent also generated additional benefits. It gave all potential participants the opportunity to understand the research study and make an informed decision about participation. Consenting prior to randomization also improved statistical power, because those who declined to participate were excluded from the study entirely rather than diluting an intervention effect by lowering take up. As noted, informed consent may have also helped researchers gain access to data even if a HIPAA waiver or a DUA (in the case of limited data) may have been technically acceptable.
Who was asked for consent?
All participants, in both intervention and comparison groups, were required to give consent to participate in the study. Initial eligibility checks performed by examining electronic medical records guaranteed that all potential participants approached by recruiters met most inclusion criteria. The broader triage population whose records were examined did not give informed consent because this process was already part of program implementation and was not unique to the study.
If potential participants were cognitively impaired, did not meet eligibility requirements, or could not communicate with the recruiter when being approached to inform them about the program and the study, the individuals were deemed not eligible to participate and the consent process did not take place.
The study included vulnerable participants who were chronically ill, currently hospitalized, and likely economically disadvantaged. Beyond the target population for the intervention however, specific vulnerable groups governed by human subjects regulations (such as pregnant women, children, or prisoners) were either explicitly excluded (in the case of children) or recruited only incidentally.
How was program enrollment modified for the study?
The Camden Core Model was already in place before the study started. Before the study took place, Camden Coalition staff identified eligible patients using real time data from their Health Information Exchange (HIE) — a database which covers four Camden hospital systems — and then approached patients while in the hospital to explain the program and to invite them to enroll. Because of program capacity constraints, only a small fraction of the eligible population could be offered the program, and participant prioritization was ad hoc prior to the beginning of the study.
The study was designed with similar inclusion and exclusion criteria and a similar triage and bedside enrollment process as the one the program had in place. For randomization, researchers created a randomization list with study IDs and random assignments prior to enrollment. Triage staff, who identified eligible patients using the HIE, assigned a study ID to potential participants without knowing intervention and comparison assignments. Informed consent and the baseline survey took place at the bedside, and recruiters revealed random assignments at the end of the enrollment process. The Camden Coalition hired additional full time recruiters to meet the scale of the study and strictly separated functions of their data team, triage staff, and recruiters in order to preserve the integrity of random assignment.
When did random assignment occur and why?
Recruiters revealed random assignments immediately after the informed consent process and the baseline survey were completed. Randomizing after consent was extremely important for two distinct reasons: bias and power. Randomizing after consent ensured that participation rates were balanced between intervention and comparison groups; this helped to prevent bias because individuals could not self-select into the study at different rates based on intervention assignment. Had the study team randomized prior to seeking consent, some individuals offered the program may have declined participation in the study, lowering take-up and reducing statistical power. Randomizing after consent also greatly increased power since researchers were able to exclude those who declined to consent from the study entirely, resulting in increased take up. This approach, however, places burdens on enrollment staff who must inform participants when they are assigned to the comparison group that they cannot access the intervention. As a result, it is important for researchers to discuss these issues early with the implementing partner and brainstorm whether there are ways to conduct randomization after recruitment in a manner that the partner is comfortable with.
Who conducted enrollment?
The study team used enrollment specialists employed by Camden Coalition who, while separate from other program staff, were sensitive to participant concerns about research participation. Some specialists were newly hired while others were redeployed from other positions within the Camden Coalition. As much as possible, the Camden Coalition hired specialists from the community where the study took place to allow for greater cultural and demographic alignment with participants. To ensure staffing across the full study period, however, they were flexible in this criterion.
Enrollment specialists approached potential participants to describe the program and seek consent prior to random assignment. Recruiters were bilingual, to communicate with patients in either English or Spanish. They were trained to approach eligible patients in a timely manner and in a standardized way following study protocols. They were also trained to introduce themselves and ask the patient questions to assess how they were feeling prior to talking about the intervention, to assess whether patients had the cognitive capacity to listen to and understand the information being provided, and to give consent. Camden Coalition staff led the development of this training, building off how enrollment was conducted prior to the RCT.
Some patients were wary of participating in research in general, and both patients and recruitment specialists often felt discouraged when patients were assigned to the comparison group. By limiting enrollment to a small number of recruitment specialists, researchers and the Camden Coalition were able to provide specialized support and training to the recruitment specialists. The Camden Coalition provided staff funding for therapy to support their mental health. The recruitment specialists also supported each other and developed best practices, including language to introduce the study without promising service, methods of preventing undue influence, and ways to support disappointed patients. To help maintain momentum and morale throughout the study period, the team celebrated enrollment milestones with a larger group of Camden Coalition staff, which helped to illustrate that enrollment was a part of a broader organizational goal.
How were risks and benefits described?
The IRB deemed this study minimal risk. The only risk highlighted during enrollment was the loss or misuse of protected health information. The study team noted that this risk existed for both intervention and comparison groups and that they were taking steps to mitigate it. Benefits were similarly modest, and as noted above, recruiters made sure to avoid over-promising potential benefits to participants. The benefits included potential useful information derived from the results of the study, and receipt of the program (which was described as potentially improving interactions with health care and social service systems). Although not considered a research best practice, the $20 compensation for completing the survey was also listed as a benefit.
How were participants compensated?
Participants who completed the baseline survey (administered after consent and prior to randomization) were given a $20 gift card for their time. Recruiters informed participants that the survey would take approximately 30 minutes.
Randomized Evaluation of the Nurse-Family Partnership
Description of the study
J-PAL affiliated researchers partnered with the South Carolina Department of Health and Human Services (DHHS), other research collaborators, and other partners to conduct a randomized evaluation of the Nurse-Family Partnership (NFP) program. This program pairs low-income first-time mothers with a personal nurse who provides at-home visits from early pregnancy through the child’s second birthday. The goal of the program is to support families during the transition to parenthood and through childrens’ early childhood to improve their health and wellbeing. The program includes up to 40 home visits (15 prenatal visits, 8 postpartum visits up to 60 days after delivery, and 17 visits during the child’s first two years), with services available in Spanish and English. An expansion of the program in South Carolina to nearly double its reach presented an opportunity to evaluate the program and measure its impact on adverse birth outcomes like low birth weight, child development, maternal life changes through family planning, and other outcomes, further described in the study protocol.
Different channels were used to identify potential participants, including direct referral through local health care providers, schools, and Special Supplemental Nutrition Program for Women, Infants, and Children (WIC) agencies; direct referrals from the Medicaid eligibility database to NFP; referrals by friends or family members; knowledge of the program through digital and printed advertisement; or identification of participants by the outreach team hired for the study period for this purpose. After identification, NFP nurses who were also direct service providers visited the potential participants at their homes or private location of their choice, assessed their eligibility, and if eligible, conducted the informed consent process. After obtaining informed consent, the nurses administered the baseline survey, after which the participant was compensated with a $25 gift card for their time, and received a referral list to other programs and services in the area. Immediately after, participants were randomized into the intervention or comparison group using the survey software SurveyCTO. Two-thirds of participants were randomly allocated to the intervention group and one-third to the comparison group. Enrollment ran from April 1, 2016 to March 17, 2020.
Enrollment at a glance:
- Enrollment period: 4 years (April 1, 2016 to March 17, 2020)
- Sample size: 5,655 Medicaid-eligible, nulliparous pregnant individuals at less than 28 weeks’ gestation
- Subjects contacted: 12,189 eligible and invited to participate
- Who enrolled: Nurse home visitors (direct service providers)
- Where enrolled: Participant’s home or location of preference
- When enrolled: Enrolled prior to random assignment; on the spot randomization
- Level of risk: Minimal risk
- Enrollment compensation: $25 gift card
Which activities required consent?
Similar to Health Care Hotspotting, the NFP study protocol required consent from participants to be part of the evaluation, as well as consent to use their personal identifiable information to link to administrative data for up to 30 years. These data included Medicaid claims data, hospital discharge records, and vital statistics, as well as a broad range of linked data covering social services, education, mental health, criminal justice, and more. This data allowed the researchers to gather information on the health and well-being of mothers and children. For those assigned to the intervention group, program participation involved a separate consent process.
Who was asked for consent?
All participants, in both intervention and comparison groups, were required to give consent to participate in the study. Potential participants were first-time pregnant people who were less than 28 weeks’ gestation, were income-eligible for Medicaid during their pregnancy, older than 15, and lived in a catchment area served by NFP nurses. Participants consented for themselves and for their children, as stated in the informed consent process.
The NFP program focused on enrolling people in early pregnancy to ensure the home visits included the prenatal period. People were excluded from the study if they were incarcerated or living in a lockdown facility, or under the age of 15. Program services were provided in English, Spanish, and additional translation services were available for participants who spoke other languages. To be eligible for the study, people also needed to have enough language fluency that they would be able to benefit therapeutically from the program.
To identify children born during the study, researchers probabilistically matched mothers to births recorded in vital records, using social security number, birth date, name, and Medicaid ID.
How was program enrollment modified for the study?
NFP had a decentralized method of identifying participants for their program prior to the study. Once patients were identified, nurses would enroll them as part of the first home visit. Under this system, the program served about 600 women per year.
Alongside the randomized evaluation, the NFP program was scaled up to serve an average of 1,200 people per year, compared to 600 people served prior to the study. Extra personnel were hired to conduct outreach to new eligible participants.
Although the randomized evaluation coincided with an expansion of the program and the hiring of new staff, including an outreach team, NFP leadership requested that the nurse home visitors who make home visits be the ones conducting study enrollment. Therefore, nurses provided on-the-spot randomization and informed participants about their study group. NFP and local implementing partners believed their nurses were better equipped to work with the study population rather than external surveyors, as nurses who deliver the program are well-trained in working with low-income, first-time mothers from the local communities. They also believed that shifting the recruitment model to a centralized process with enrollment specialists was infeasible given the scale of the study and the program.
The study’s informed consent process was incorporated into the pre-existing program recruitment and consent process, therefore participants received both program and study information at the same time. This allowed the study team to ensure participants also received clear information about the program.
When did random assignment occur and why?
Random assignment was conducted on-the-spot, after participants consented to the study and completed the baseline survey. The survey software, SurveyCTO, automatically assigned participants to either an intervention or comparison group. Two-thirds of the sample was randomly allocated to receive the treatment intervention and one-third to the comparison group.
As in Health Care Hotspotting, consent prior to randomization guaranteed that there was balance between intervention and comparison groups as both would have received the same information and would be equally likely to consent to be part of the study. On-the-spot randomization also maximized statistical power, as only those who consented to participate were randomized, instead of randomizing and then approaching participants — who may decline to participate. Although withdrawal after randomization could still occur, for those in the intervention group, nurses were able to immediately conduct the first home visit, so this strategy helped improve take-up of the intervention.
Who conducted enrollment?
Nurse home visitors conducted enrollment and randomization with support from a recruitment support specialist (a research associate) at J-PAL North America. This involved investment from the research team to dedicate sufficient staff capacity to train and support nurses during the four years in which enrollment took place. The research associate was responsible for training nurses on study protocols, providing remote and in-person field support, monitoring survey recordings for quality and compliance checks, managing gift cards and study tablets, and maintaining morale and building relationships with nurses.
Nurses invested in learning how to be survey enumerators in addition to delivering high-quality nursing care. The research team trained all nurses on how to recruit, assess eligibility, deliver informed consent, randomize patients into intervention and comparison groups, and deliver the baseline survey using SurveyCTO software on a tablet. All nurses had to complete this training before they could enroll patients. The research team went to South Carolina before the start of the study and conducted a two-day in-person training to practice obtaining informed consent and using tablets to administer the baseline survey. Yearly refresher trainings were offered.
Communicating comparison group assignment was one of the main challenges for nurses during study enrollment. Nurses navigated this challenge by handing patients the tablet and having them press the randomization button, which helped nurses and patients to remember that assignment to a group was automatically generated and out of the control of both parties.
The research team provided additional resources to mitigate the concern that nurses may not adhere to random assignments. The research team conducted quarterly in-person enrollment and field trainings for nurses and nurse supervisors that highlighted benefits for comparison group participants, including that all comparison group participants benefit from meeting with a caring health care professional who can help with Medicaid enrollment if needed and receive a list of other available services.They also reminded nurses that the evaluation helped to expand services to more patients than would otherwise be served.
The research team’s recruitment support specialist operated a phone line that nurses could call for emotional and technical support, coordinated in-person and web-based training for new nurses, sent encouragement to the nurses, and monitored fidelity to the evaluation design through conducting audio checks on the delivery of informed consent and baseline survey. The support specialist also troubleshooted any issues with the tablet in real time and helped to resolve any data input errors...
How were risks and benefits described?
The primary risk of participating in the study was the loss or misuse of protected health information. The benefits included potentially useful information derived from the results of the study, and receipt of the intervention, which could improve the health and wellbeing of participants and their child.
How were participants compensated?
After completing the baseline survey, all study participants were compensated with a $25 Visa gift card for the time it took them to complete the survey.
Acknowledgements: Thanks to Amy Finkelstein, Catherine Darrow, Jesse Gubb, Margaret McConnell, and Noreen Giga for their thoughtful contributions. Amanda Buechele copy-edited this document. Creation of this resource was supported by the National Institute On Aging of the National Institutes of Health under Award Number P30AG064190. The content is solely the responsibility of the authors and does not necessarily represent the official views of the National Institutes of Health.
J-PAL’s Research Resources
J-PAL’s Research Resources provide additional information on topics discussed in this resource, including the Regular intake and consent research resource.
Health Care Hotspotting
- Evaluation summary
- Paper
- Protocol
- Consent forms
Nurse Family Partnership
- Evaluation summary
- Paper
- Protocol
- Consent form can be found in the Protocol, Additional File 3
Camden Coalition of Healthcare Providers. “Camden Core Model.” Camden Coalition of Healthcare Providers. (December 2, 2021). Accessed February 6, 2023. https://camdenhealth.org/care-interventions/camden-core-model/.
Camden Coalition of Healthcare Providers. “The Camden Core Model – Patient Selection and Triage Methodology.” Camden Coalition of Healthcare Providers. Accessed February 6, 2023. https://camdenhealth.org/wp-content/uploads/2019/11/Care-Management_Triage_11022018_v5.pdf.
Finkelstein, Amy, Annetta Zhou, Sarah Taubman, and Joseph Doyle. “Health Care Hotspotting — a Randomized, Controlled Trial.” New England Journal of Medicine 382, no. 2 (2020): 152–62. https://doi.org/10.1056/nejmsa1906848.
Finkelstein, Amy, Annetta Zhou, Sarah Taubman, and Joseph Doyle. “Supplementary Appendix. Healthcare Hotspotting – A Randomized Controlled Trial.” New England Journal of Medicine 382, no. 2 (2020): 152–62. https://www.nejm.org/doi/suppl/10.1056/NEJMsa1906848/suppl_file/nejmsa1906848_appendix.pdf.
Finkelstein, Amy, Annetta Zhou, Sarah Taubman, and Joseph Doyle. “Supplementary Appendix. Healthcare Hotspotting – A Randomized Controlled Trial.” New England Journal of Medicine 382, no. 2 (2020): 152–62. https://www.nejm.org/doi/suppl/10.1056/NEJMsa1906848/suppl_file/nejmsa1906848_protocol.pdf.
Harvard T.H. Chan School of Public Health. “Partners.” South Carolina Nurse-Family Partnership Study Website. Accessed February 6, 2023. https://www.hsph.harvard.edu/sc-nfp-study/partners/.
Harvard T.H. Chan School of Public Health. “Pay for Success.” South Carolina Nurse-Family Partnership Study, July 1, 2022. https://www.hsph.harvard.edu/sc-nfp-study/about-the-study-about-the-study/pay-for-success/.
Harvard T.H. Chan School of Public Health. “People.” South Carolina Nurse-Family Partnership Study Website. Accessed February 6, 2023. https://www.hsph.harvard.edu/sc-nfp-study/people/.
McConnell, Margaret A., Slawa Rokicki, Samuel Ayers, Farah Allouch, Nicolas Perreault, Rebecca A. Gourevitch, Michelle W. Martin, et al. “Effect of an Intensive Nurse Home Visiting Program on Adverse Birth Outcomes in a Medicaid-Eligible Population.” JAMA 328, no. 1 (2022): 27. https://doi.org/10.1001/jama.2022.9703
McConnell, Margaret A., R. Annetta Zhou, Michelle W. Martin, Rebecca A. Gourevitch, Maria Steenland, Mary Ann Bates, Chloe Zera, Michele Hacker, Alyna Chien, and Katherine Baicker. “Protocol for a Randomized Controlled Trial Evaluating the Impact of the Nurse-Family Partnership’s Home Visiting Program in South Carolina on Maternal and Child Health Outcomes.” Trials 21, no. 1 (2020). https://doi.org/10.1186/s13063-020-04916-9.
The Abdul Latif Jameel Poverty Action Lab. “Health Care Hotspotting in the United States.” The Abdul Latif Jameel Poverty Action Lab (J-PAL). Accessed February 6, 2023. https://www.povertyactionlab.org/evaluation/health-care-hotspotting-united-states.
The Abdul Latif Jameel Poverty Action Lab. “The impact of a nurse home visiting program on maternal and early childhood outcomes in the United States.” The Abdul Latif Jameel Poverty Action Lab (J-PAL). Accessed December 22, 2022, from https://www.povertyactionlab.org/evaluation/impact-nurse-home-visiting-program-maternal-and-early-childhood-outcomes-united-states.
South Carolina Healthy Connections. “Fact Sheet: South Carolina Nurse -Family Partnership Pay for Success Project.” Accessed February 6, 2023. https://socialfinance.org/wp-content/uploads/2016/02/021616-SC-NFP-PFS-Fact-Sheet_vFINAL.pdf.
Lessons for assessing power and feasibility from studies of health care delivery
Introduction
This resource highlights key lessons for designing well-powered randomized evaluations, based on evidence from health care delivery studies, funded and implemented by J-PAL North America.1 Determining whether a study is sufficiently powered to detect effects is an important decision to make at the outset of a project, to determine whether a project is worth pursuing. There are dangers to underpowered studies; for example, if the lack of a statistically significant result is interpreted as evidence that a program is ineffective, rather than underpowered. Although designing well-powered studies is critical in all domains, health care delivery presents particular challenges — direct effects on health outcomes are often difficult to measure and for whom an intervention is effective is a particularly important question because differential effects can have a dramatic impact in the type of care received. Health care delivery settings also present opportunities, in that implementing partners have important complementary expertise to address these challenges.
Key takeaways
- Communicate with partners to:
- Choose outcomes that align with the program’s theory of change
- Gather data for power calculations
- Select meaningful minimum detectable effect sizes
- Assess whether a study is worth pursuing
- Anticipate challenges when measuring health outcomes:
- Plan to collect primary data from sufficiently large samples
- Choose health outcomes that can be impacted during the study period and consider prevalence in the sample
- Consider whether results, particularly null results, will be informative
- Think carefully about subgroup analysis and heterogeneous effects:
- Set conservative expectations about subgroup comparisons, which may be underpowered or yield false positives
- Calculate power for the smallest comparison
- Stratify to improve power
- Choose subgroups based on theory
The value of talking with partners early (and often)
Power calculations should be conducted as early as possible. Starting conversations about power calculations with implementing partners early not only provides insight on the feasibility of the study, but also allows a partner to be involved in the research process and offer necessary inputs like data. Estimating power is fundamental to help researchers and implementing partners understand the other's perspective and create a common understanding of the possibilities and constraints of a research project. Implementing partners can be incredibly useful in making key design decisions that affect power.
Decide what outcomes to measure based on theory of change
An intervention may affect many aspects of health and its impact could be measured by many outcomes. Researchers will need to decide what outcomes to prioritize to ensure adequate power and a feasible study. Determining what outcomes to prioritize collaboratively with implementing partners helps ensure that outcomes are theory-driven and decision-relevant while maximizing statistical power.
- In Health Care Hotspotting, an evaluation of the Camden Coalition of Healthcare Providers’ care coordination program for high cost, high need patients, researchers and the Camden Coalition chose hospital readmission rates as their primary outcome. This was not straightforward — the program may have had additional benefits in other domains — but this outcome aligned with the program’s theory of change, was measurable in administrative data, and ensured adequate power despite the limitations imposed by a relatively small sample. Although there was also interest in measuring whether the intervention could reduce hospital spending, this outcome was not chosen as a primary outcome2 because there was not sufficient power and because cost containment was less central to the program’s mission.
Provide data and understand constraints
While it is possible to conduct power calculations without data by making simplifying assumptions, partners can provide invaluable information for initial power calculations. Data from partners, including historical program data and statistics, the maximum available sample size (based on their understanding of the target population), and take-up rates gleaned from existing program implementation, can be used to estimate power within the context of a study. Program data may be preferable to data drawn from national surveys or other sources because it comes from the context in which an evaluation is likely to operate. However, previous participants may differ from study participants, so researchers should always assess sensitivity to assumptions made in power calculations. (See this resource for more information on testing sensitivity to assumptions).
- A randomized evaluation of Geisinger Health’s Fresh Food Farmacy program for patients with diabetes used statistics from current clients as baseline data for power calculations in their pre-analysis plan. This allowed researchers to use baseline values of the outcomes (HbA1c, weight, hospital visits) that were more likely to be reflective of the population participating in the study. It also allowed researchers to investigate how controlling for lagged outcomes would improve power. Without collaboration from implementing partners, these measures would be hard to approximate from other sources.
- In Health Care Hotspotting, the Camden Coalition provided historical electronic medical record (EMR) data, which gave researchers inputs for the control mean and standard deviation necessary to calculate power for hospital readmissions. The actual study population, however, had twice the assumed readmission rate (60% instead of 30%), which led to a slight decrease in power relative to expectations. This experience emphasizes the importance of assessing sensitivity.
Determine reasonable effect sizes
Choosing reasonable effect sizes is a critical component of calculating power and often a challenge. In addition to consulting academic literature, conversations with partners can help determine what level of precision will be decision-relevant. The minimum detectable effect (MDE) required for an evaluation should be determined in that particular context, where the partner’s own cost-benefit assessment or a more standardized benchmark may guide discussions. The risks of interpreting underpowered evaluations often fall on the implementing partner, where lack of power increases the likelihood that an intervention is wrongly seen as ineffective, so choosing a minimum detectable effect should be a joint decision between the research team and partner.
- In Health Care Hotspotting, researchers were powered to detect a 9.6 percentage point effect on the primary outcome of hospital readmissions. While smaller effects were potentially clinically meaningful, the research team and their partners determined that this effect size would be meaningful because it would rule out the much larger 15-45% reductions in readmissions found in previous evaluations of similar programs delivered to a different population (Finkelstein et al. 2020).
- In Fresh Food Farmacy, researchers produced target minimum detectable effects (see Table 2) in collaboration with their implementing partners and demonstrated that the study sample size would allow them to detect effects below these thresholds.
- Implementing partner judgment is critical when deciding to pursue an evaluation that can only measure large effects. Researchers proceeded with an evaluation of legal assistance for preventing evictions, which compared an intensive legal representation program to a limited, much less expensive, and more scalable program, based on an understanding that the full representation program would only be valuable if it could produce larger effects than the limited program. Estimating potentially small effects with precision was not of interest, given the cost of the program and the immense, unmet need for services.
In the section "Calculate minimum detectable effects and consider prevalence of relevant conditions" below, we further cover how special considerations such as prevalence of a condition have to also be considered when determining reasonable effect sizes.
Assess feasibility and make contingency plans
Early conversations about power calculations can help set clear expectations on parameters for study feasibility and define protocols for addressing future implementation challenges. Sometimes early conversations lead to a decision not to pursue a study. Talking points for non-technical conversations about power are included in this resource.
- During a long-term, large-scale evaluation of the Nurse Family Partnership in South Carolina, early power conversations proved to be helpful when making decisions in response to new constraints. Initially the study had hoped to enroll 6,000 women over a period of four years, but recruitment for the study was cut short due to Covid-19. Instead, the study enrolled 5,655 women, 94% of what was originally targeted. Since the initial power calculations anticipated a 95% participation rate and used conservative assumptions, the decision to stop enrollment due to the pandemic was made without concern that it would jeopardize power for the evaluation. Given the long timeline of the study and turnover in personnel, it was important to revisit conversations about power and involve everyone in the decision to halt enrollment.
- A recent study measuring the impact of online social support on nurse burnout was halted due to lack of power to measure outcomes. In the proposed study design, the main outcome was staff turnover. Given a sample of approximately 25,000 nurses, power calculations estimated a minimum detectable effect size of 1.5 percentage points, which represents a 8 to 9 percentage point reduction in staff turnover. When planning for implementation of the study, however, randomization was only possible at the nursing unit level. Given a new sample of approximately 800 nursing units, power calculations estimated a minimum detectable effect size of 7 to 10 percentage points, which represents roughly a 50 percent reduction in turnover. These new power calculations assumed complete take-up, which did not seem feasible to the research team. With the partner’s support, other outcomes of interest were explored, but these had low rates of prevalence in administrative data. Early conversations about study power surfaced constraints early, which prevented embarking on an infeasible research study.
Challenges of measuring health outcomes
Data collection and required sample sizes are two fundamental challenges to studying impacts on health outcomes. Health outcomes, other than mortality, are rarely available in administrative data, or if they are, may be differentially measured for treatment and control groups.3 For example, consider researchers who wish to measure blood pressure but rely on measurements taken at medical appointments. Only those who choose to go to the doctor appear in the data. If the intervention increases appointments attended, the treatment group will be more prevalent in the data in comparison to the control group, further biasing the result. Therefore, measuring health outcomes usually requires researchers to conduct costly primary health data collection to avoid bias resulting from differential coverage in administrative data.4
Required sample sizes also pose challenges to research teams studying health care interventions. Because many factors influence health, health care delivery interventions may be presumed to have small effects. This requires large sample sizes or lengthy follow-up periods. Additionally, health impacts are likely to be downstream of health care delivery or behavioral interventions that may have limited take up, further reducing power.5 As a result of these challenges, many evaluations of health care delivery interventions measure inputs into health — for example, receiving preventive services like a flu shot (Alsan, Garrick, and Graziani 2019) or medication adherence — instead of, or in addition to, measuring downstream health outcomes. These health inputs can often be measured in administrative data and directly attributed to the intervention.
Despite these challenges, researchers may wish to demonstrate actual health impacts of an intervention when feasible. This section introduces key steps in the process of designing a health outcome measurement strategy illustrated by two cases, the Oregon Health Insurance Experiment (OHIE) and a study examining a workplace wellness program. These cases highlight thoughtful approaches and challenges to estimating precise effects and include examples for interpreting null results.
Choose measurable outcomes plausibly affected by the intervention in the time frame allotted
Not all aspects of health will be expected to change as a result of a particular intervention, especially within a study timeframe. For instance, preventing major cardiovascular events like heart attacks may be the goal of an intervention, but it is more feasible to measure blood pressure and cholesterol, both of which are risk factors for heart attacks, than to measure heart attacks, which might not occur for years or at all. What to measure should be determined by a program’s theory of change, an understanding of the study population, and the length of follow up.
- In the OHIE, researchers measured the effects of Medicaid6 on hypertension, high cholesterol, diabetes, and depression. These outcomes were chosen because they were “important contributors to morbidity and mortality, feasible to measure, prevalent in the low-income population of the study, and plausibly modifiable by effective treatment within two years” (Baicker et al, 2013). For insurance to lead to observable improvements in these outcomes, receiving Medicaid would need to increase health care utilization, lead to accurate diagnoses, and generate effective treatment plans that are followed by patients. Any “slippage” in this theory of change, such as not taking prescribed medication, would limit the ability to observe effects. Diabetes, for example, was not as prevalent as expected in the sample, reducing power relative to initial estimates.
- In an evaluation of a workplace wellness program, researchers measured effects of a wellness program on cholesterol, blood glucose, blood pressure, and BMI, because they were all elements of health plausibly improved by the wellness program within the study period.
Make sample size decisions and a plan to collect data.
Measuring health outcomes will likely require researchers to collect data themselves, in person, with the help of medical professionals. In this situation, data collection logistics and costs will limit sample size. The costs of data collection must be balanced with sample sizes needed to draw informative conclusions. In each study, researchers restricted their data collection efforts in terms of geography and sample size. In addition, clinical measurements were relatively simple, involving survey questions, blood tests7, and easily portable instruments. Both of these strategies addressed cost and logistical constraints.
- In the OHIE, clinical measures were only collected in the Portland area, with 20,745 people receiving health screenings, despite the intervention being statewide with a study sample size of over 80,000.
- In workplace wellness, researchers collected clinical data from all 20 treatment sites but only 20 of the available 140 control sites in the study.
Calculate minimum detectable effects and consider prevalence of relevant conditions
In the health care context, researchers should also consider the prevalence of individuals who are at risk for a health outcome and for whom we can expect the intervention to potentially address that outcome. In other words, researchers must understand the number of potential compliers with that aspect of treatment, where a compiler receives that element of the intervention only when assigned to treatment, in comparison to other participants who may always or never receive the intervention.
Interventions like health insurance or workplace wellness programs are broad and multifaceted, but measurable outcomes may only be relevant for small subsets of the population. Consider, for example, blood sugar and diabetes. We should only expect HbA1c (a blood sugar measure) to change as a result of the intervention for those either with diabetes or at risk for diabetes. The theory of change for reducing HbA1c as a result of insurance requires going to a provider, being assessed by a provider, and prescribed either a behavioral modification or medication. If most people do not have high blood sugar and are therefore not told by their provider to reduce it, we should not expect the intervention to affect Hba1c. If this complier group is small relative to the study sample, the intervention will be poorly targeted, and this will reduce power similarly to low take-up or other forms of noncompliance.
Suppose we have a sample of 1,000 people, 10 percent with high HbA1c, and another 10 percent who have near-high levels that would prompt their provider to offer treatment. Initial power calculations with a control mean of 10 percent produce an MDE of about 6 percentage points (for a binary high/low outcome). However, once we correct for 20 percent compliance, observing this overall effect requires a direct effect for compliers of roughly 30 percentage points. This is significantly larger and might make a seemingly well-powered study seem infeasible.
In cases where data from the sample is not yet available, broad based survey or administrative data can be used to measure population level (i.e. control group) outcome means and standard deviations and the prevalence of certain conditions, as well as investigate whether other variables are useful predictors of chosen outcomes and can be included in analysis to improve precision. As always, the feasibility of a study should be assessed using a wide range of possible scenarios.
- In the OHIE, researchers noted that they overestimated the prevalence of certain conditions in their sample. Ex-post, they found that only 5.1% had diabetes and 16.3% had high blood pressure. This limited the effective sample size in which one might expect an effect to be observed, effectively reducing take up and statistical power relative to expectations. One solution when relevant sample sizes are smaller than expected is to restrict analysis to subgroups expected to have larger effects ex ante, such as those with a preexisting condition, for which the intervention may be better targeted.
- In the workplace wellness study, the researchers note that they used data from the National Health and Nutrition Examination Survey, weighted to the demographics of the study population, to estimate control group statistics. These estimates proved quite accurate resulting in the expected power to detect effects.
In both cases, researchers proceeded with analysis while acknowledging the limits of their ability to detect effects.
Ensure a high response rate
Response rates have to be factored into anticipated sample size and given that sample size is a critical input for determining sufficient power, designing data collection methods to prevent attrition is an important strategy to maintain sample size throughout the duration of a study. Concerns about attrition grow when data collection requires in-person contact and medical procedures.8
- To ensure a high response rate in Oregon, researchers devoted significant resources to identifying and collecting data from respondents. This included several forms of initial contact, a tracking team devoted to locating respondents with out-of-date contact information, flexible data collection (interviews were done via several methods and health screenings could be performed in a clinic or at home), intensive follow up dedicated to a random subset of non-respondents, and significant compensation for participation ($30 for the interview, an additional $20 for the dried blood spot collection, and $25 for travel if the interview was at a clinic site). These efforts resulted in an effective response rate of 73%. Dried blood spots (a minimally invasive method of collecting biometric data) and short forms of typical diagnostic questionnaires were used to reduce the burden on respondents. These methods are detailed in the supplement to Baicker et al. 2013.
- In workplace wellness, health surveys and biometric data collection were done on site at workplaces and employees received a $50 gift card for participation. Some employees received an additional $150. Participation rates in the wellness program among current employees were just above 40%. However, participation in data collection was much lower — about 18% — primarily because less than half of participants ever employed during the study period were employed during data collection periods. Participants had to be employed at that point in the study, present on those particular days, and be willing to participate in order to be included in data collection.
Understand your results and what you can and cannot rule out
Both the OHIE and workplace wellness analyses produced null results on health outcomes, but not all null results are created equal.
- The OHIE results differed across outcome measures. There were significant improvements only in the rate of depression; depression was also the most prevalent condition of the four examined. There were no detectable effects on diabetes or blood pressure, but what could be concluded in each of these domains differed. Medicaid’s effect on diabetes was imprecise, with a wide confidence interval that included both no effect and large positive effects, including a very plausible positive effect. The results could not rule out the effect one might expect to find if you estimated how many people had diabetes, saw their doctor as a result of getting insurance, got medication, and how effective that medication is at reducing HbA1c (based on clinical trial results). This is not strong evidence of no effect. In contrast, Medicaid’s null effect on blood pressure could rule out much larger previous estimates from quasi-experimental work because its confidence interval did not include the larger estimates generated from quasi-experimental work (Baicker et al, 2013, The Oregon Experiment: Effects of Medicaid on Clinical Outcomes).
- The effects on health shown in Workplace Wellness were all nearly zero. Given that impact measurements were null across a variety of outcome measures and another randomized evaluation of a large scale workplace wellness program found similar results, it is reasonable to conclude that the workplace wellness program did not affect health significantly in the study time period.
Does health insurance not affect health?
New research has since demonstrated health impacts of insurance, but the small effect sizes emphasize why large sample sizes are needed. The IRS partnered with researchers to randomly send letters to taxpayers who paid a tax penalty for lacking health insurance coverage, encouraging them to enroll. They sent letters to 3.9 million out of 4.5 million potential recipients. The letters were effective at increasing health insurance coverage and in reducing mortality, but the effects on mortality were small: among middle aged adults (45-64 years old) they saw a 0.06 percentage point decline in mortality, one fewer death for about 1,600 letters.
Being powered for subgroup analysis and heterogeneous effects
Policymakers, implementing partners, and researchers may be interested in for whom a program works best, not only in average treatment effects for a study population. However, subgroup analysis is often underpowered and increases the risk of false positive results due to the larger number of hypotheses being tested. Care must be taken to balance the desire for subgroup analysis and the need for sufficient power.
Talk to partners and set expectations about subgroups
Set conservative expectations with partners before analysis begins about what subgroup analyses may be feasible and what can be learned from them. Underpowered comparisons and large numbers of comparisons should be treated with caution as the likelihood of Type I errors (false positives) will be high. These result from multiple hypothesis testing and because underpowered estimation, conditional on finding a statistically significant effect, will overestimate the true effect.
Conduct power calculations for the smallest relevant comparison
If a study is well-powered for an average treatment effect, it may not be powered to detect effects within subgroups. Power calculations should be done using the sample size of the smallest relevant subgroup. Examining heterogeneous treatment effects (i.e. determining whether effects within subgroups are different from each other) require even more sample size to be powered.9
Stratify to improve power
Stratifying on variables that are strong predictors of the outcome can improve power by guaranteeing that these variables are balanced between treatment and control groups. Stratifying by subgroups may improve power for subgroup analyses. However, stratifying on variables that are not highly correlated with the outcome may reduce statistical power by reducing the degrees of freedom in the analysis.10
Ground subgroups in theory
Choose subgroups based on the theory of change of the program being evaluated. These might be groups where you expect to find different (e.g., larger or smaller) effects or where results are particularly important. Prespecifying and limiting the number of subgroups that will be considered can guard against concerns about specification searching (p hacking) and force researchers to consider only the subgroups that are theoretically driven. It is also helpful to pre-specify any adjustments for multiple hypothesis testing.
When examining differential effects among subgroups is of interest, but it is not possible to pre-specify relevant groups, machine learning techniques may allow researchers to flexibly identify data-driven subgroups, but this also requires researchers to impute substantive meaning for the subgroups, which may not always be apparent.11
- In OHIE, researchers prespecified subgroups for which effects on clinical outcomes might have been stronger: older individuals, those with an existing diagnosis of hypertension, high cholesterol, or diabetes, and those with a heart attack or congestive heart failure. Even by doing this, the researchers found no significant improvements in these particular dimensions of physical health over this time period.
- In an Evaluation of Voluntary Counseling and Testing (VCT) in Malawi that explored the effect of a home based HIV testing and counseling intervention on risky sexual behaviors and schooling investments, researchers identified several relevant subgroups for which effects might differ: HIV-positive status, HIV-negative status, HIV-positive status with no prior belief of HIV infection, and HIV-negative status with prior belief of HIV infection. Though the program had no overall effect on risky sexual behaviors or test scores, there were significant effects within groups where test results corrected prior beliefs. Those who had an HIV-positive status but did not have a prior belief of HIV infection engaged in more dangerous sexual behaviors, and those who were surprised by a negative test experienced a significant improvement in achievement test scores (Baird et al 2014).
- An evaluation that used discounts and counseling strategies to incentivize the use of long term contraceptives in Cameroon used causal forests to identify subgroups that were more likely to be persuaded by price discounts. Causal forests are a machine learning technique to identify an optimal strategy for splitting a sample into groups. Using this approach, the researchers found that clients strongly affected by discount prices are younger, more likely to be students and have higher levels of education. Given this subgroup analysis, the researchers found that discounts increased the use of contraceptives by 50%, with larger effects for adolescents (Athey et al, 2021). Researchers pre-specified this approach without having to identify the actual subgroups in advance.
Acknowledgments: Thanks to Amy Finkelstein, Berk Özler, Jacob Goldin, James Greiner, Joseph J Doyle, Katherine Baicker, Maggie McConnell, Marcella Alsan, Rebecca Dizon-Ross, Zirui Song and all the researchers included in this resource for their thoughtful contributions. Thanks to Jesse Gubb and Laura Ruiz-Gaona for their insightful edits and guidance, as well as to Amanda Buechele who copy-edited this document. Creation of this resource was supported by the National Institute On Aging of the National Institutes of Health under Award Number P30AG064190. The content is solely the responsibility of the authors and does not necessarily represent the official views of the National Institutes of Health.
1 For more information on statistical power and how to perform power calculations, see the Power Calculations resource; for additional technical background and sample code, see the Quick Guide to Power Calculations; for practical tips on conducting power calculations at the start of a project and additional intuition behind the components of a well-powered study, see Six Rules of Thumb for Determining Sample Size and Statistical Power.
2 The practice of designating a primary outcome is common in health care research and is described in the checklist for publishing in medical journals.
3 The challenge of differential coverage in administrative data is discussed at length in the resource on using administrative data for randomized evaluations.
4 The exception would be events, like births or deaths, that are guaranteed to appear in administrative data unaffected by post-treatment selection bias. In an evaluation of the Nurse Family Partnership in South Carolina researchers were able to measure adverse birth outcomes like preterm birth and low birth weight from vital statistics records.
5 Program take up has an outsize effect on power. A study with 50% take up requires four times the sample size to be equally powered as one with 100% take up, because sample size is inversely proportional to the square of take up. See Power Calculations 101: Dealing with Incomplete Take-up (McKenzie 2011) for a more complete illustration of the effect of the first stage of an intervention on power.
6 Health insurance that the government provides – either for free or at a very low cost – to qualifying low-income individuals.
7 In the OHIE, 5 blood spots were collected and then dried for analysis.
8 More information about how to increase response rates in mail surveys can be found in the resource on increasing response rates of mail surveys and mailings.
9 More on the algebraic explanation can be found in the following post “You need 16 times the sample size to estimate an interaction than to estimate a main effect”.
10 One would typically include stratum fixed effects in analysis of stratified data. The potential loss of power would be of greater concern in small samples where the number of parameters is large relative to the sample size.
11More on using machine learning techniques for pre-specifying subgroups in the following blog post: “What’s new in the analysis of heterogeneous treatment effects?“
- What is the risk of an underpowered randomized evaluation?
- Power Calculations
- Quick guide to power calculations
- Six Rules of Thumb for Determining Sample Size and Statistical power
- Increasing response rates of mail surveys and mailings
- Real-Word Challenges to Randomization and their Solutions
- Pre-Analysis Plans
Evaluation Summaries
- Healthcare Hotspotting in the United States
- Randomized Evaluation of the Nurse Family Partnership in South Carolina
- Reducing Nurse Burnout through Online Social Support
- Workplace Wellness Programs to Improve Employee Health Behaviors in the United States
- The Oregon Health Insurance Experiment in the United States
- Voluntary Counseling and Testing (VCT) to Reduce Risky Sexual Behaviors and Increase Schooling Investments in Malawi
- Prescribing Food as Medicine among Individuals Experiencing Diabetes and Food Insecurity in the United States
- Matching Provider Race to Increase Take-up of Preventive Health Services among Black Men in the United States
Research Papers
- Athey, Susan, Katy Bergstrom, Vitor Hadad, Julian Jamison, Berk Özler, Luca Parisotto, and Julius Dohbit Sama. “Shared Decision-Making Can Improved Counseling Increase Willingness to Pay for Modern Contraceptives?” World Bank Document, September 2021.
- Baicker, Katherine, Sarah L. Taubman, Heidi L. Allen, Mira Bernstein, Jonathan H. Gruber, Joseph P. Newhouse, Eric C. Schneider, Bill J. Wright, Alan M. Zaslavsky, and Amy N. Finkelstein. “The Oregon Experiment — Effects of Medicaid on Clinical Outcomes.” New England Journal of Medicine 368, no. 18 (2013): 1713–22.
- Baicker, Katherine et al. “Supplementary Appendix —The Oregon Experiment: Effects of Medicaid on Clinical Outcomes.” New England Journal of Medicine 368, no. 18 (2013).
- Baird, Sarah, Erick Gong, Craig McIntosh, and Berk Özler. “The heterogeneous effects of HIV testing”. Journal of Health Economics (2014).
- Brookes, ST et al. “Subgroup Analyses in Randomised Controlled Trials: Quantifying the Risks of False-Positives and False-Negatives.” Health technology assessment (Winchester, England). U.S. National Library of Medicine.
- Finkelstein, Amy, Annetta Zhou, Sarah Taubman, and Joseph Doyle. “Health Care Hotspotting — a Randomized, Controlled Trial.” New England Journal of Medicine 382, no. 2 (2020): 152–62.
- Goldin, Jacob, Ithai Lurie, and Janet McCubbin. “Health Insurance and Mortality: Experimental Evidence from Taxpayer Outreach.” NBER, December 9, 2019.
- Song, Zirui and Katherine Baicker. “Effect of a Workplace Wellness Program on Employee Health and Economic Outcomes: A Randomized Clinical Trial”. National Library of Medicine, JAMA, April, 2019.
- Song, Zirui and Katherine Baicker. “Health And Economic Outcomes Up To Three Years After A Workplace Wellness Program: A Randomized Controlled Trial”. National Library of Medicine, JAMA, June, 2021.
- Greiner, James, Cassandra Wolos Pattanayak and Jonathan Hennessy. “How Effective Are Limited Legal Assistance Programs? A Randomized Experiment in a Massachusetts Housing Court”. SSRN, March, 2012.
Others
- “Health Expenditures by State of Residence, 1991-2020” Centers for Medicaid and Medicare Services. Accessed February 24, 2023.
- Camden Coalition
- Nurse Family Partnership
- Gelman, Andrew. “Statistical Modeling, Causal Inference, and Social Science.” Statistical Modeling Causal Inference and Social Science, March 15, 2018.
- Bulger, John, and Joseph Doyle. “Fresh Food Farmacy: A Randomized Controlled Trial - Full Text View.” ClinicalTrials.gov, August 12, 2022.
- Kondylis, Florence, and John Loeser. “Back-of-the-Envelope Power Calcs.” World Bank Blogs, Jan 29, 2020. Accessed March 2, 2023.
- Özler, Berk. “What's New in the Analysis of Heterogeneous Treatment Effects?” World Bank Blogs, May 16, 2022.
For more information on statistical power and how to perform power calculations, see the Power Calculations resource; for additional technical background and sample code, see the Quick Guide to Power Calculations; for practical tips on conducting power calculations at the start of a project and additional intuition behind the components of a well-powered study, see Six Rules of Thumb for Determining Sample Size and Statistical Power.
Acquiring and using administrative data in US health care research
Summary
This resource provides guidance on acquiring and working with administrative data for researchers working in US health care contexts, with a particular focus on how the Health Insurance Portability and Accountability Act (HIPAA) structures data access. It illustrates the concepts with examples drawn from J-PAL North America’s experience doing research in this area. This resource assumes some knowledge of administrative data, IRBs, and the Common Rule. Readers seeking a comprehensive overview of how to obtain and use nonpublic administrative data for randomized evaluations across multiple contexts should consult the resource on using administrative data for randomized evaluations.
Disclaimer: This document is intended for informational purposes only. Any information related to the law contained herein is intended to convey a general understanding and not to provide specific legal advice. Use of this information does not create an attorney-client relationship between you and MIT. Any information provided in this document should not be used as a substitute for competent legal advice from a licensed professional attorney applied to your circumstances.
Introduction
There are a number of advantages to using administrative data for research, including cost, reduced participant burden and logistical burden for researchers, near-universal coverage and long term availability, accuracy, and potentially reduced bias. For health research in particular, administrative data contains precise records of health care utilization, procedures, and their associated cost, which would be difficult or impossible to learn from surveying participants directly.1
Despite these advantages, a key challenge for research in health care contexts in the United States is acquiring administrative data when researchers are outside the institution that generated the data. Protected Health Information (PHI) governed by the Health Insurance Portability and Accountability Act (HIPAA) makes health care data especially sensitive and challenging for researchers to acquire, which may cause lengthy negotiation processes and confusion about the appropriate level of protection for transferring and using the data.
This resource covers:
- The relationships between HIPAA, human subjects research regulations, and researchers
- Levels of data defined by HIPAA
- Important considerations for making a health care data request
- Compliance with IRBs and DUAs
"An example of the value of administrative data over survey data can be seen in the Oregon Health Insurance Experiment’s study of the impact of covering uninsured low-income adults with Medicaid on emergency room use. This randomized evaluation found no statistically significant impact on emergency room use when measured in survey data, but a statistically significant 40 percent increase in emergency room use in administrative data (Taubman, Allen, Wright, Baicker, & Finkelstein 2014). Part of this difference was due to greater accuracy in the administrative data than the survey reports; limiting to the same time periods and the same set of individuals, estimated effects were larger in the administrative data and more precise” (Finkelstein & Taubman, 2015).
What is HIPAA and how does it affect researchers?
In the United States, the Privacy Rule of the Health Insurance Portability and Accountability Act (HIPAA) regulates the sharing of health-related data generated or held by health care entities such as hospitals and insurance providers. HIPAA imposes strict data protection requirements with strict penalties and liability for non-compliance to the health care entities that it regulates (known as covered entities). These regulations allow, but do not require, sharing data for research. These regulations augment the more general research protections codified in the Common Rule—the US federal policy for the protection of human subjects that outlines the criteria and mechanisms for IRB review—sometimes in overlapping and confusing ways.
Researchers (in most cases) are not covered entities, however researchers must understand HIPAA requirements in order to interact with data providers who are bound by them. Many of the obligations to protect data under HIPAA will be passed to the researcher and their institution through data use agreements (DUAs) executed with covered entities.2 HIPAA imposes restrictions on what and how covered entities may disclose for research. HIPAA introduces distinct categories of data for health care research that researchers should understand, as they dictate much of the compliance environment a particular research project will fall under. In addition, researchers must understand how HIPAA requirements interact with requirements from the Common Rule. Note that HIPAA only applies in the United States and only to health care data; other topic areas and countries may have their own compliance standards.3
What kind of data does HIPAA cover?
The HIPAA Privacy Rule is a US federal law that covers disclosure of Protected Health Information (PHI) generated, received, or maintained by covered entities and their business associates. Covered entities are health care providers, health plans, and health care clearinghouses (data processors). Business associates include organizations that perform functions on behalf of a covered entity that may involve protected health information. In the research context, researchers may interact with business associates who work as data processors, data warehouses, or external analytics or data science teams. Data from covered entities used in research may include “claims” data from billing or insurance records, hospital discharge data, data from electronic medical records like diagnoses and doctors’ notes, or vital statistics like dates of birth.
Protected health information (PHI) refers to identifiable health data maintained or received by a covered entity or its business associates. More specifically, the HIPAA Privacy Rule defines 18 identifiers and 3 levels of data — research identifiable data, also known as research identifiable files (RIF), limited data sets (LDS) and de-identified data — each with different requirements depending on their inclusion of these identifiers, detailed in Table 1 below. Data without any of the 18 identifiers is considered de-identified, does not contain PHI, and is therefore not restricted by HIPAA. The inclusion of any identifiers means that the data is subject to at least some restrictions. Notably, between fully identified and de-identified data, HIPAA defines a middle category — limited data — that contains some potentially-identifiable information and is subject to some but not all restrictions. As a result, the extent to which a data request must comply with HIPAA depends on the level of protected health information contained in the data to be disclosed.
Not all health data is protected health information (PHI) governed by HIPAA. Health information gathered by researchers themselves during an evaluation is not PHI, though it would be personally identifiable information subject to human subject protections under the Common Rule.4 Not all secondary data providers are necessarily covered entities either. Student health records from a university-affiliated medical center, for example, are governed by the education privacy law FERPA rather than HIPAA, because FERPA offers stronger privacy protections. Researchers should confirm whether their implementing partner or data provider is a covered entity, as some organizations will incorrectly assume they are subject to HIPAA, invoking unnecessary review and regulation. For guidance ondetermining whether an organization is subject to the HIPAA Privacy Rule, the US Department of Health & Human Services (HHS) defines a covered entity and the Centers for Medicare & Medicaid Services (CMS) provides a flowchart tool.
Levels of data
Research identifiable data
Research identifiable data contain sufficient identifying information such that the data may be directly matched to a specific individual. Under HIPAA this means any identifiers (such as name, address, or record numbers) not including the dates and locations allowed in limited data sets (LDS). A list of variables that make a dataset identifiable under the HIPAA Privacy Rule can be found in Table 1. Identifiable data may only be shared for research purposes with individual authorization from each patient or a waiver of authorization approved by an institutional review board (IRB) or privacy board (a process similar to informed consent or waivers of consent as required by the Common Rule). This level of data is typically not necessary to conduct analysis for impact evaluations if a data provider or third party is able to perform data linkages on the researcher’s behalf. Researchers may need to acquire identified data to perform data linkages or because the data provider is not able or willing to remove identifiers prior to sharing the data.
Researchers receiving research identifiable files must take pains to protect data with strong data security measures and by removing and separately storing identifiers from analysis data after they are no longer needed (a J-PAL minimum must do). Researchers should expect extensive negotiations and review of their data request and to receive IRB approval and execute a DUA prior to receiving data. Protecting participant data confidentiality is a vital component of conducting ethical research.
Limited data sets
The HIPAA Privacy Rule defines limited data sets as those that contain geographic identifiers smaller than a state (but less exact than street address) or dates related to an individual (including dates of medical service and birthdates for those younger than 90) but do not otherwise contain HIPAA identifiers. For research purposes, this geographic and time information (such as hospital admission and discharge dates) can be particularly useful for analysis. This makes limited data a particularly useful class of administrative health data that balances ease of access with ease of use.
Limited data sets may be shared by a covered entity with a data use agreement (DUA).5 HIPAA does not require individual authorization or a waiver of authorization. Researchers should always seek IRB approval for research involving human subjects or their data, but it is possible that research using only a limited data set may be determined exempt or not human subjects research by an IRB if there is no other involvement of human subjects. Using a limited data set for randomized evaluations typically means that another party besides the researcher, such as the data provider or an intermediary, must link data to evaluation subjects.
- In Health Care Hotspotting, J-PAL affiliated researchers partnered with the Camden Coalition of Healthcare Providers in New Jersey to evaluate the impact of a care transition program that provided assistance to high-cost, high-need patients with frequent hospitalizations and complex social needs, known as “super-utilizers.” Researchers executed multiple DUAs for limited data sets. As discussed above, working with hospital discharge data required matching to study participants on site in Camden, NJ before taking limited data back to MIT for analysis. For other datasets, including Medicaid data used in secondary analysis, the Camden Coalition submitted a finder file to the data provider, who matched study participants to their outcome data before delivering a dataset stripped of identifiers to the researchers. More options for data transfers where researchers cannot receive identifiers are discussed in the resource on using administrative data for randomized evaluations.
De-identified or publicly available data
De-identified data does not contain any of the 18 HIPAA identifiers and is therefore considered not to contain sufficient identifiers to link to specific individuals with certainty. HIPAA permits health care providers to share de-identified data for research purposes without further obligations (U.S. Department of Health & Human Services 2018). De-identified data is not PHI.
Using de-identified data means that it is possible to obtain data through a much less onerous legal and contractual process, with less risk. However, this is balanced against the need for the data provider to conduct any linkages and to pre-process any information that cannot be included, such as turning dates of service into relative dates or exact locations into distances if such information is needed for analysis.
- For the study “Clinical decision support for high-cost imaging: A randomized clinical trial,” researchers received only de-identified data from the implementing partner and data provider Aurora Health Care. This approach simplified legal compliance but necessitated additional work on the side of the data provider (and trust on the side of the researchers) to make the data usable for research, such as converting dates of service to days measured relative to the introduction of the intervention. This case is documented in significant detail in a chapter of J-PAL’s IDEA handbook
HIPAA Identifier |
De-identified |
Limited Data Set |
Research Identifiable |
1. Names or initials |
X | ||
2. Geographic subdivisions smaller than a state |
First 3 digits of zip code (provided that the geographic unit formed by combining all ZIP codes with the same 3 initial digits contains >20,000 people) |
City, state, zip code (or equivalent geocodes) |
X |
3. Dates directly related to an individual (such as birth dates, hospital admissions and discharges) |
Year |
X | X |
4. Telephone numbers |
X | ||
5. Fax numbers |
X | ||
6. Email addresses |
X | ||
7. Social Security numbers |
X | ||
8. Medical record numbers |
X | ||
9. Health plan beneficiary numbers |
X | ||
10. Account numbers |
X | ||
11. Certificate/license numbers |
X | ||
12. Vehicle identifiers and serial numbers, including license plate numbers |
X | ||
13. Device identifiers and serial numbers |
X | ||
14. Web Universal Resource Locators (URLs) |
X | ||
15. Internet protocol (IP) addresses |
X | ||
16. Biometric identifiers, including fingerprints and voiceprints |
X | ||
17. Full-face photographs or comparable images |
X | ||
18. Any other unique identifying number, characteristic, or code |
X |
Considerations for making a data request
Picking a level of data
Researchers should understand what level of data is required to conduct their research. Using the least identifiable data possible will lower compliance costs and may increase the speed of negotiations and their likelihood of success. However, data without sufficient information can limit your research questions and additional data preparation may be required when working with de-identified data. Researchers should consider what is necessary to conduct their research and request what they need. Requesting limited data is often a good compromise as it balances these considerations.
The level of data is not always under researcher control. Data providers that frequently work with researchers may be able to provide essentially the same data in several formats. However data providers may also limit what is possible with each data type.
- For research using Medicare data, identifiable data is often necessary as using a unique beneficiary identifier is needed to track individuals across datasets and not all limited datasets include this information. Medicare also limits the dates allowed in limited datasets relative to the HIPAA definition. Researchers interested in working with CMS data should consult ResDAC’s documentation defining what is included in each file type.6
- For Health Care Hotspotting, researchers requested uniform billing data from the New Jersey Department of Health for all hospital discharges in the state related to an 800 patient trial in Camden, NJ. The health department preferred to send all data on discharges in the state, with identifiers. Researchers balanced the opportunity to conduct the match to study participants themselves with MIT’s desire not to receive identified data by having the implementing partner, the Camden Coalition of Healthcare Providers, receive the data, matching the data onsight in Camden, and only transferring the matched limited data set back to MIT. This process nonetheless required researchers to implement strong data security requirements and required a lengthy data request process.
Minimum necessary requirement
In addition to considering data in terms of the level of identification, HIPAA requires that data providers release only the minimum information “reasonably necessary to accomplish the purpose [of the research].” This restriction applies to data that contains PHI unless researchers have individual authorization from research subjects. Covered entities are required to develop their own protocols for determining what constitutes the minimum necessary, so the restrictiveness of this requirement varies significantly. Covered entities may also rely on IRB approval of a data request as evidence of meeting the minimum necessary standard. Researchers should consider the scope of a data request both in terms of the number of individuals and the number of variables requested. Minimizing the size of a request in either dimension can help smooth the data request process. Researchers should be prepared to justify the inclusion of particular variables as necessary to the research. To limit the number of individuals requested, it may be possible to request a random sample, particularly in cases where treatment is not assigned at the individual level or when requesting data for observational research.
Additional data provider requirements
Data providers may apply additional restrictions or conditions beyond what the law requires for the researcher to use their administrative data, especially for data considered “sensitive” for any reason. Practically speaking, researchers must follow whatever additional requirements data providers request. Data holders are typically under no obligation to share data.
Researchers may be asked to demonstrate how their research will benefit the program from which they are requesting data. In some cases this may be to prioritize responding to requests for data. In others this may be legally required. Emphasizing the value of the research can be helpful in building trust and interest in any data request process.
Researchers may be asked to submit their project for additional IRB review beyond what their own institution requests.
- In Health Care Hotspotting, the New Jersey Department of Health requires all data requests to be approved by the Rowan University IRB, which reviews projects on their behalf and which will not cede to an existing IRB.
- In an evaluation of the End Stage Renal Disease Treatment Choice model, a payment reform model to encourage greater use of home dialysis, CMS required a waiver of individual authorization issued by MIT’s IRB in order to process the request to access Medicare data via the Virtual Research Data Center (VRDC). Because MIT had initially determined the project exempt, researchers had to go back to the IRB and request a full review in order to be granted the waiver. CMS’s own Privacy Board also reviews requests for identifiable data.
Data use agreements frequently extend required protections for PHI to data elements that are not PHI, such as information about doctors or hospitals rather than patients. Researchers may also be asked to implement onerous data security requirements, whereas the Privacy Rule requires that researchers only "use appropriate safeguards," not the full list of administrative, technical, and physical safeguards the HIPAA Security Rule envisions for covered entities with complex businesses. In the experience of research staff at J-PAL North America, this has included setting up automated audit trails, intrusion detection, and vulnerability scans on research servers, which required close collaboration with IT staff and went significantly beyond standard operating procedures.
Researchers are typically not in a strong negotiating position for reducing additional requirements. However it can be helpful to be knowledgeable about what HIPAA requires and what safeguards the research team and institution provide in order to build trust. Building trust and highlighting the value of the research to the data provider may increase the provider’s motivation to work with you. (Further consideration of each of these points is included in the resource on using administrative data). For example, particularly in cases where data providers do not frequently share data, providers may be unaware that outside researchers are not covered entities or unaware of the different requirements related to levels of identified data.7 Data providers who initially request security frameworks appropriate for handling health care data at enterprise scale may be satisfied with more limited measures already in place in a research computing environment, especially if researchers can speak knowledgeably about them and remain sensitive to the data provider’s concerns.8
How to receive data
How data will be received or accessed by researchers has implications for data security as well as cost. It may be possible to send data directly to a personal research server from a data provider via secure file transfer, or have the data provider send data to a data intermediary that maintains suitable infrastructure for research access. The National Bureau of Economic Research (NBER), for example, houses CMS data in use by multiple researchers. It may also be possible to access data remotely on a system maintained by the data provider instead of taking physical possession of the data.
- As an alternative to receiving actual copies of data, in an evaluation of the End Stage Renal Disease Treatment Choice model researchers chose to access Medicare data remotely, using CMS’s VRDC. This access method provides significant advantages in terms of speed of access (decreased lag time between when an event occurs and when it is reflected in the data), cost, and ease of use (responsibility for data security infrastructure remains with the data provider).
Compliance
Researchers should expect to need to receive IRB approval and execute a DUA prior to receiving data. Even de-identified data may be considered sensitive by the data provider and require a legal agreement. Researchers have their own obligation to seek IRB approval under the Common Rule and data providers may request evidence that someone external to the research team has signed off on a project and its use of data even if not required under HIPAA. In most instances, an IRB will also function as an institution’s HIPAA Privacy Board.
IRB Approval and HIPAA Authorization
Researchers seeking access to identifiable data can do so with either individual authorization or a waiver of authorization. In either case, an IRB serves to document and approve the authorization form or the waiver.
An authorization to release protected health information is a signed record of an individual’s permission to allow a HIPAA Covered Entity to use or disclose their Protected Health Information (PHI). The authorization must describe the information requested and the purpose, and must be written in plain language that is readily understood by the individual. This is similar to the concept of informed consent, and is often embedded within an informed-consent document. However, an authorization has a distinct set of criteria and may be a separate written agreement obtained outside of the informed-consent process. Researchers should consult their IRB for specific guidelines or templates. If possible it is also recommended to confirm with a data provider that a planned authorization will be sufficient to access data.
The waiver standard is likewise quite similar to the waiver of individual consent under the Common Rule. Research must be no more than minimal risk, could not be accomplished without the protected health information being requested, and could not practically be accomplished without the waiver. In other words, it would not be possible to contact subjects to seek their consent, which may be the case in randomized evaluations where researchers are not involved in the delivery of the intervention, where the program does not interact with all research subjects — such as an informational intervention or encouragement design that does not contact the control group — or in observational research.
For researchers seeking a limited data set, HIPAA only requires a DUA. However, researchers (who are not covered entities and therefore bound by the Common Rule not the HIPAA Privacy Rule) must still seek IRB approval for human subjects research. In the case that research involves only limited data and not other contact with human subjects, it may be determined exempt under category 4 (ii) by an IRB because limited data is not “readily identifiable,” despite the inclusion of PHI in the form of dates and locations. Research involving only de-identified data is not considered human subjects research. Note that in the context of running randomized evaluations, there are likely elements of the project beyond the data set that would require human subjects compliance. When in doubt about what constitutes human subjects research, researchers should consult their institution’s IRB. IRB approval is a minimum must do for all J-PAL projects.
Data Use Agreement (DUA)
A data use agreement outlines the allowable uses of the data to be transferred and the requirements the receiving institution must adhere to for protecting the data. A DUA is a legal contract executed by the institutions sharing and receiving data. DUAs should be signed by a legal representative of the institution, not the researcher.
HIPAA lists specific requirements for what a data use agreement must include. In particular, recipients must agree to the following:
- "Not to use or disclose the information other than as permitted by the data use agreement or as otherwise required by law;
- Use appropriate safeguards to prevent the use or disclosure of the information other than as provided for in the data use agreement;
- Report to the covered entity any use or disclosure of the information not provided for by the data use agreement of which the recipient becomes aware;
- Ensure that any agents, including a subcontractor, to whom the recipient provides the limited data set agrees to the same restrictions and conditions that apply to the recipient with respect to the limited data set; and
- Not to identify the information or contact the individual.”
Data providers may impose additional restrictions or safeguards. However, the DUA should also make clear the freedom of the researcher to publish the results of their work without interference from the data provider, except for reasonable review of publications to ensure no accidental disclosures.
Rather than construct a DUA from scratch, researchers should make use of an existing DUA template already in use by one of the parties and approved by their legal office. Universities will have standardized forms and many data providers, particularly if they share data frequently, will as well. For example, CMS’s DUA templates are linked on the ResDAC website. Despite a large degree of standardization, researchers should be aware that DUA negotiations can often take significant time (months, if not more) and plan for these delays.
For guidance on formulating a data request and executing a data use agreement, please see the resource on using administrative data for randomized evaluations.
Relationship between IRB and DUA
Simultaneously seeking IRB approval and executing a data use agreement often generates a circular problem where the IRB wants to approve a research protocol that includes a data use agreement and the data provider wants to execute a data use agreement with researchers that have already received IRB approval. IRBs want to review data use agreements, as they contain details about what information will be acquired about study participants and how that information will be handled. Data providers, in contrast, often request proof that IRB approval has been received, before beginning negotiations.
It may be possible to get provisional approval from one party to move forward with approval from the other party and then iterating between the two, as noted in the resource on using administrative data for randomized evaluations. In practice, this has typically meant first seeking IRB approval, providing as much detail as possible on the data request process, using that approval to execute the DUA, and then submitting the DUA (and any additional details, as necessary) as an amendment to the IRB protocol.
If an IRB does in fact require documentation from a data provider, it may be possible to submit the data request application materials, which will often detail the purpose of the data for the research, the specific variables requested for the study, specify how data will be linked and potentially de-identified, and explain the data security plan, which are the necessary elements that an IRB will consider when making a determination about a research project. If the IRB requests approval from a data provider that does not yet exist, it may be possible to produce a letter of intent or support from the data provider, indicating general support for providing data to the research project, with the specifics of the data transfer subject to further negotiation and the execution of the DUA. (An example of such a letter is included as an attachment).
Acknowledgments: We are grateful to Catherine Darrow, Amy Finkelstein, Laura Ruiz, Lisa Turley Smith, and Fatima Vakil for their insight and advice. This resource is heavily indebted to the creators of J-PAL’s original and more expansive guide to using administrative data for randomized evaluations: Laura Feeney, Jason Bauman, Julia Chabrier, Geeti Mehra, and Michelle Woodford. Amanda Buechele copyedited this document. Creation of this resource was supported by the National Institute On Aging of the National Institutes of Health under Award Number P30AG064190. The content is solely the responsibility of the authors and does not necessarily represent the official views of the National Institutes of Health
1 The benefits of administrative data, as well as the challenges, limitations, and risks, are covered in great detail in the resource on using administrative data for randomized evaluations.
2 Researchers would be a covered entity if employed by a health care provider, or in some cases if doing research using health data from their own institution, such as researchers at an university doing research with data from an affiliated medical center. This resource addresses the more typical situation for social scientists conducting randomized evaluations where the data provider is a separate institution from the researchers.
3 Education data in the United States is covered by the Family Educational Rights and Privacy Act (FERPA). Health care data in other countries will be covered by other rules. The General Data Protection Regulation (GDPR) in the European Union provides broad protections across a range of topic areas, and other countries have adopted similarly broad privacy laws. Researchers should consult experts in their particular context before proceeding with a data request.
4 Consult the resource on IRB proposals for more information or talk to your IRB, which may have institution-specific HIPAA guidance.
5 More information on DUAs is below and in the resource on using administrative data for randomized evaluations.
6 The Research Data Assistance Center (ResDAC) at the University of Minnesota is the official broker for research data requests to CMS. ResDAC provides a wealth of information on CMS data available for research, provides technical assistance to researchers, and conducts the first round of review for data requests before they are passed to CMS.
7 Confusion over roles and responsibilities under HIPAA may also extend a researcher’s own institution.
8 Of course, researchers are also ethically obligated to avoid taking advantage of provider ignorance.
J-PAL Research Resources
- For a more exhaustive resource on admin data please see J-PAL’s resource Using administrative data for randomized evaluations
- For additional resources on IRB approval, consult the resources on IRBs and working with IRBs in health care contexts
HIPAA guidance
- The US Department of Health & Human Services provides a detailed guide to the requirements associated with research identifiable health data and how the HIPAA Privacy Rule applies to research and a guide to understanding HIPAA for all types of users.
- 45 CFR 164.502 – Uses and disclosures of protected health information (original text).
- NIH guidance on complying with HIPAA, including de-identified health information, Authorizations, and Authorization waivers.
- 45 CFR 164.514 – Describes the HIPAA standard for de-identification of protected health information (original text).
- 45 CFR 160.103 – Defines Individually identifiable health information.
- HIPAA defines a Minimum Necessary Requirement: Covered entities and business associates must make reasonable efforts to limit disclosures of protected health information to the minimum necessary to accomplish the intended purpose of the use, disclosure, or request. Though a limited data set is permitted to contain, for example, an individual's exact birthdate, the birthdate should only be included if it serves a specific research purpose.
- HHS defines covered entities and business associates and CMS provides a helpful flowchart.
- IRBs often provide their own HIPAA documentation, for example MIT’s IRB COUHES has this guidance document.
Data sources
- The ResDAC website provides a wealth of information for researchers considering using CMS data. Of note, CMS and ResDAC define limited data sets and research identifiable files slightly differently by excluding most dates from limited data sets. ResDAC is the official broker for research data requests to CMS and conducts the first round of review on data requests and also provides technical assistance to researchers.
- J-PAL Administrative Data Catalog houses access procedures and related information for datasets used in randomized evaluations, largely in the United States.
DUAs
- J-PAL’s IDEA Handbook includes a chapter on Model Data Use Agreements (O’Hara 2022) which includes a template DUA with sample text as an appendix. .
Eval summaries
IDEA
Feeney, Laura, and Amy Finkelstein. 2022. “Aurora Health Care: Using Electronic Medical Records for a Randomized Evaluation of Clinical Decision Support.” In: Cole, Dhaliwal, Sautmann, and Vilhuber (eds), Handbook on Using Administrative Data for Research and Evidence-based Policy, Version v1.1. Accessed at https://admindatahandbook.mit.edu/book/latest/ahc.html#fn260 on 2023-06-01.
Papers
- Doyle J, Abraham S, Feeney L, Reimer S, Finkelstein A (2019) Clinical decision support for high-cost imaging: A randomized clinical trial. PLoS ONE 14(3): e0213373. https://doi.org/10.1371/journal.pone.0213373
- Ji Y, Einav L, Mahoney N, Finkelstein A. Financial Incentives to Facilities and Clinicians Treating Patients With End-stage Kidney Disease and Use of Home Dialysis: A Randomized Clinical Trial. JAMA Health Forum. 2022;3(10):e223503. doi:10.1001/jamahealthforum.2022.3503 https://jamanetwork.com/journals/jama-health-forum/fullarticle/2797080
- Finkelstein, Amy, and Sarah Taubman. 2015. “Randomize Evaluations to Improve Health Care Delivery.” Science 347 (6223): 720–22. https://doi.org/10.1126/science.aaa2362.
- Finkelstein, Amy, Zhou, Annetta, Taubman, Sarah, and Doyle, Joseph. 2020. Health Care Hotspotting — A Randomized, Controlled Trial. New England Journal of Medicine 382(2). https://doi.org/10.1056/NEJMsa1906848
- Taubman, S. L., H. L. Allen, B. J. Wright, K. Baicker, and A. N. Finkelstein. 2014. “Medicaid Increases Emergency-Department Use: Evidence from Oregon’s Health Insurance Experiment.” Science 343 (6168): 263–68. doi:10.1126/science.1246183.
Navigating hospital Institutional Review Boards (IRBs) when conducting US health care delivery research
Summary
This resource provides guidance to avoid challenges when partnering with a hospital to implement a randomized evaluation and working with the hospital's institutional review board (IRB), which may be more familiar with medical research than social science evaluations. Conducting randomized evaluations in these settings may involve logistical considerations that researchers should be aware of before embarking on their experiment. Topics include how to write a protocol to avoid confusion, objections, and delay by taking pains to thoroughly explain a study design and demonstrate thoughtful approaches to randomization, risks and benefits, and how research impacts important hospital constituencies like patients, providers, and vulnerable groups. The resource also addresses how to structure IRB review when work involves multiple institutions or when research intersects with quality improvement (QI) in order to reduce concerns about which institution has responsibility for a study and to minimize administrative burden over the length of a study. For a more general introduction to human subjects research regulations and IRBs, including responding to common concerns of IRBs not addressed here, please first consult the resource on institutional review board proposals.
The challenge
Randomized evaluations, also known as randomized controlled trials (RCTs), are increasingly being used to study important questions in health care delivery, although the methodology is still not as commonly used in the social sciences as it is in studies of drugs and other medical interventions (Finkelstein 2020; Finkelstein and Taubman 2015). Economists and other social scientists may seek to partner with hospitals to conduct randomized evaluations of health care delivery interventions. However, compared to conducting research at their home institutions, it may seem as if some hospital IRBs may have a more lengthy process for reviewing social science RCTs. While all IRBs follow the guidelines set forth in the Common Rule, differences in norms and expectations between disciplines may complicate reviews and require clear communication strategies.
Lack of familiarity with social science RCTs
Hospital IRBs, accustomed to reviewing randomized evaluations in the context of clinical trials, may review social science RCTs as if they were comparatively higher risk clinical trials, while researchers may feel that social science RCTs are generally less risky. These IRBs may be more accustomed to reviewing clinical trials, research involving drugs or devices, or more intensive behavioral interventions. Thus, a protocol that mentions randomization may prompt safety concerns and lead to review processes required for trials that demand more scrutiny and risk mitigation.
Randomization
Randomization invites scrutiny on decisions about who receives an intervention, as well as scrutiny of the intervention itself. This can cause a disconnect for the researcher when trying to justify the research, or trigger assumptions that reviewers may have regarding ethics. Is the researcher denying someone something they should be able to receive? Unlike a drug or device that requires testing, it may not be clear to a hospital IRB why an intervention, for instance, to increase social support or to provide feedback on prescribing behavior to physicians cannot be applied to everyone.
Researchers being external to the reviewing IRB organization
Although not a challenge inherent to the field of health care delivery, partnering with a hospital to conduct research may involve researchers interacting with a new IRB. This presents challenges when IRBs are unaware of, or unable to independently assess the expertise of researchers, may not understand the division of labor between investigators at the hospital and elsewhere, or may not be clear on where hospital responsibility for a project starts and ends. This may also pose logistical challenges if it limits who has access to IRB software systems.
Despite these challenges, researchers affiliated with J-PAL North America have successfully partnered with hospitals to conduct randomized evaluations.
- In Clinical Decision Support for High-cost Imaging: A Randomized Clinical Trial, J-PAL affiliated researchers partnered with Aurora Health Care, a large health care provider in Wisconsin and Illinois to conduct a large-scale randomized evaluation of a clinical decision support (CDS) system on the ordering of high-cost diagnostic imaging by healthcare providers. Providers were randomized to receive CDS in the form of an alert that would appear within the order entry system when they ordered scans that met certain criteria. Aurora Health Care’s IRB reviewed the study and approved a waiver of informed consent for patients, the details of which are well documented in the published paper.
- In Health Care Hotspotting, J-PAL affiliated researchers partnered with the Camden Coalition of Healthcare Providers in New Jersey and several Camden area hospitals (each with their own IRB) to evaluate the impact of a care transition program that provided assistance to high-cost, high-need patients with frequent hospitalizations and complex social needs, known as “super-utilizers.” The study recruited and randomized patients while in the hospital, although the bulk of the intervention was delivered outside of the hospital after discharge. Each recruiting hospital IRB reviewed the study.
- In Prescribing Food as Medicine among Individuals Experiencing Diabetes and Food Insecurity, J-PAL affiliated researchers partnered with the Geisinger Health System in Pennsylvania to evaluate the impact of the Fresh Food Farmacy (FFF) “food-as-medicine” program on clinical outcomes and health care utilization for patients with diabetes and food insecurity. A reliance agreement was established between MIT and the Geisinger Clinic, with the Geisinger Clinic’s IRB serving as the reviewing IRB.
- In Encouraging Abstinence Behavior in a Drug Epidemic: Does Age Matter? J-PAL affiliated researchers partnered with DynamiCare Health and Advocate Aurora Health to evaluate whether an app-based abstinence incentive program through a mobile application is effective for older adults with opioid use disorders. Advocate Aurora Health reviewed the study, and a reliance agreement was not needed, as it was determined that the co-PIs at the University of California, Santa Cruz and the University of Chicago were not involved in human subjects research.
The following considerations are important to ensure positive relationships, faster reviews, and increased likelihood of approval when working with hospital IRBs:
Considerations for the institutional arrangement
Partner with a PI based at the IRB's institution
RCTs generally involve partnerships between investigators and implementing partners. Hospitals differ from typical implementing partners in that they are research institutions with their own IRBs. Consequently, hospital partnerships often require partnership with a researcher based at that hospital. Therefore, if you want to work with a hospital to conduct your research, plan to partner with a PI based at that hospital. Beyond the substantive knowledge and expertise that a hospital researcher brings, there may be practical benefits for the IRB process. This includes both institutional knowledge and the mundane task of clicking submit on an internal IRB website. Procedures for approving external researchers vary from hospital to hospital and having an internal champion in this process can be invaluable.
Building a relationship with a local PI as your co-investigator can be beneficial for a number of reasons: utilizing content expertise during the research design, addressing the alignment of the research goals with the mission, interest, and needs of the hospital, and also in navigating IRB logistics. A local PI may already have knowledge of nuances to consider when designing a protocol and submitting for IRB review.
- In the evaluation of clinical decision support, J-PAL and MIT-based researchers partnered with Aurora-based PI, Dr. Sarah Reimer, who played a key role in getting the study approved. Aurora requires all research activities to obtain Research Administrative Preauthorization (RAP) before submitting the proposal to the IRB for approval. Dr. Reimer ensured the research design made it through the formal steps of both reviews. She also helped with the approval process allowing the external J-PAL investigators to participate in the research, which included a memo sent to Aurora’s IRB explaining their qualifications, experience, and role on the project.
- Challenges in collaboration are always a possibility. In Health Care Hotspotting, researchers initially partnered with Dr. Jeffrey Brenner, the founder of the Camden Coalition and a clinician at the local hospitals. When Dr. Brenner left the Coalition and the research project, the research team needed to find a hospital-based investigator in order to continue managing administrative tasks like submitting IRB amendments.
Decide which IRB should review
Multi-site research studies (including those with one implementation site but researchers spread across institutions, if all researchers are engaged in human subjects research) can generally opt to have a single IRB review the study with the other IRBs relying on the main IRB. In fact, this is required for NIH-funded multi-site trials. Implementation outside of the NIH-required context is more varied.
All else equal, it may be easier to submit and manage an IRB protocol at one’s home university, with partnering hospitals ceding review. However, not all institutions will agree to cede review. This seems to be the case particularly when most activities will take place at another institution (e.g., you are the lead PI based at a university but recruitment is taking place at a hospital and the hospital IRB does not want to cede review). Sometimes hospitals may require their own independent IRB review, which results in the same protocol being reviewed at two (or more) institutions. Understanding each investigator’s role and level of involvement in the research, along with the research activities happening at each site is crucial when thinking about a plan to submit to the IRB. Consult the IRBs and talk to your fellow investigators about how to proceed with submitting to the IRB and the options for single IRB review. For long-term studies, consider how study team turnover and the possibility of researchers changing institutions will affect these decisions.
- In Health Care Hotspotting, all four recruiting hospitals required their own IRB review. MIT, where most researchers were based, ceded review to Cooper University Hospital, the primary hospital system where most recruitment occurred. Cooper has since developed standard operating procedures for working with external researchers and determining who reviews. Other institutions may have similar resources. Maintaining separate approvals proved labor intensive for research and implementing partner staff.
- Reliance agreements can be easier with fewer institutions. In Prescribing Food as Medicine among Individuals Experiencing Diabetes and Food Insecurity, a reliance agreement was established between MIT (where the J-PAL affiliated researcher was based) and the Geisinger Health System. MIT ceded to Geisinger, since recruitment and the intervention took place at Geisinger affiliated clinics.
We recommend single IRB review whenever possible, because a single IRB will drastically reduce the effort required for long term compliance, such as submitting amendments and continuing reviews. While setting up single IRB review initially may not be straightforward, doing so will pay dividends over the length of the project.
If a researcher at a university wants to work with a co-investigator at a hospital and have only one IRB oversee the research, a reliance agreement or institutional authorization agreement (IAA) will be required by the IRBs. Researchers select one IRB as the ‘reviewing IRB’, which provides all IRB oversight responsibilities. The other institutions sign the agreement stating that they agree to act as ‘relying institutions’ and their role is to provide any conflicts of interest, the qualifications of their study team members, and local context if applicable but otherwise cede review.
SMART IRB is an online platform aimed at simplifying reliance agreements, funded through the NIH National Center for Advancing Translational Sciences. NIH now requires a single reviewing IRB for NIH-funded multisite research. Many institutions use SMART IRB, however some may use their own paper based systems or use a combination of both (e.g., SMART IRB for medical studies that require it, paper forms for social science experiments). An example of a reliance agreement form can be found here. The process of getting forms signed and sent back and forth between institutions can take time. If you are planning to collaborate with a researcher at another institution, plan ahead by looking into whether they have SMART IRB and talk to other researchers about their experiences with obtaining a reliance agreement via traditional forms.
Anticipate roadblocks to reliance agreements to save yourself time in the future and avoid potential compliance issues. Explain your roles and responsibilities in the protocol, check to see if your institution(s) use SMART IRB, discuss with your co-investigator(s) which institution should be the relying institution, and talk to your IRB if you are unsure whether a reliance agreement will be needed.
Understand who is “engaged” in research
It is fairly common for research teams to be spread across multiple universities; however, not all universities may be considered engaged in human subjects research. Considering which institutions must approve the research can cut down on the number of approvals needed, even in cases of single IRB review.
An institution is engaged in research if an investigator at that institution is substantively involved in human subjects research. Broadly, “engagement” involves activities that merit professional recognition (e.g., study design) or varying levels of interaction with human subjects, including direct interaction with subjects (e.g., consent, administering questionnaires) or interaction with identifiable private information (e.g., analysis of data provided by another institution). If you have co-investigators who are not involved in research activities with subjects and do not need access to the data, even if they are collaborators for authorship and publication purposes, reliance may not be necessary (See Engagement of Institutions in Human Subjects Research, Section B 11). To learn more about criteria for engagement, please review guidance from the Department of Health & Human Services. When in doubt, an IRB may issue a determination that an investigator is “not engaged” in human subjects research.
- In Treatment Choice and Outcomes for End Stage Renal Disease, the study team was based at MIT, Harvard, and Stanford. Data access was restricted such that only the MIT-based team could access individual data. The study design, analysis, and paper writing were collaborative efforts, but the IRBs determined that only MIT was engaged in human subjects research.
- One J-PAL affiliated researcher experienced a weeks-long exchange when trying to set up a reliance agreement between their academic institution and their co-PI’s hospital. As co-PIs they designed the research protocol together. The plan was for recruitment and enrollment of patients into the study to take place at the hospital where the co-PI was situated and for our affiliate to lead the data analyses. The affiliate initiated the reliance agreement, but because the study was taking place at the hospital and our affiliate would not actually be involved in any of the direct research activities (e.g., recruitment, enrollment), their IRB questioned whether their level of involvement even qualified as human subjects research and subsequently did not see the need for a reliance agreement. Our affiliate provided their IRB with justification of their level of involvement in the study design and planned data analyses. An academic affiliation was also set up for the J-PAL affiliate at the co-PI’s hospital for data access purposes. Because the study received federal funding, the investigators emphasized the need for compliance with the NIH’s single IRB policy. Ultimately, the reliance agreement was approved.
Determine whether your study is Quality Improvement (QI)
Quality improvement (QI) is focused on evaluating and identifying ways to improve processes or procedures internally at an organization. Because the focus is more local, the goals of QI differ from research, which is defined as “a systematic investigation… to develop or contribute to generalizable knowledge” (45 CFR 46.102(2)). Instead, QI has purposes that are typically clinical or administrative. QI projects are not human subjects research and are not subject to IRB review. However, hospitals typically have an oversight process for QI work, including making a determination that a project is QI and thus not human subjects research. If you are doing QI, it is crucial to follow the local site’s procedures, so make sure to discuss your work with the IRB or, if separate, the office responsible for QI determinations.
Quality improvement efforts are common in health care. In some cases, researchers may be able to distinguish between the QI effort and the work to evaluate it. In such work, the QI would receive a determination of “not human subjects research” and would not be overseen by the IRB, whereas a non-QI evaluation would be considered research and would receive oversight from the IRB. If the evaluation only involved retrospective data analysis, it might be eligible for simplified IRB review processes, such as exemption or expedited review.
Quality improvement projects are often published as academic articles. The intent to publish does not mean your QI project fits the regulatory definition of research (45 CFR 46.102(d)) and should not, on its own, hinder you from pursuing a QI project. If your QI project also has a research purpose, then regulations for the protection of human subjects in research may apply. In this case, you should discuss with the IRB and/or the office responsible for QI determinations. The OHRP has an FAQ page on Quality Improvement Activities that can help you get a better understanding of the differences between QI and research.
Understanding QI matters because hospital-based partners may feel that a project does not require IRB review. While a project may be intended to evaluate and identify ways to improve processes or procedures, there may be research elements embedded in the design. If so, then your project must be reviewed by an IRB, per federal regulations. If you are unsure if your project can be classified as QI, human subjects research, or both, talk to your IRB and/or the office that makes QI determinations.
Additionally, there may be funder requirements to be mindful of when it comes to IRB review. For example, J-PAL’s Research Protocols require PIs to obtain IRB approval, exemption, or a non-human subjects research determination.
- The Rapid Randomized Controlled Trial Lab at NYU Langone, run by Dr. Leora Horwitz, does exclusively QI, because the lab prioritizes the speed of projects. By limiting the projects to just QI and certain types of evaluations, the lab is able to complete projects within a timespan of weeks. Researchers have investigated non-clinical improvements to the health system, such as improving post-discharge telephone follow-up.
- Lessons from Langone: QI projects may not need IRB review, but that doesn’t mean ethical considerations should not be taken seriously. Before getting started, the researchers discussed the approach with their IRB, who then determined that their projects qualified as QI. Two hallmarks of QI work include: no collection of personal identifiers, since this is generally unnecessary in evaluation of an effect, and the prioritization of oversubscribed interventions to avoid denying patients access to a potentially beneficial program (Horowitz, et al 2019).
Quality improvement should not be undertaken to avoid ethical review of research. As the researcher, it is your obligation to be honest and thorough in designing your protocol and assessing the roles human subjects will play and how individuals may be affected.
Do not outsource your ethics! Talk to your IRB about your options for review if you think your protocol involves both QI and research activities. It is always possible to submit a protocol, which may lead to a determination that a project is not human subjects research, or exempt from review.
Consider splitting up your protocol
A research study may involve several components, some of which may qualify for exemption or expedited review while others will not. For example, researchers may be interested in accessing historical data (retrospective chart review, defined below) to inform the design of a prospective evaluation. In this case, the research using historical data could be submitted to the IRB as one protocol, likely qualifying for an exemption, while the prospective evaluation could later be submitted as a separate protocol. Removing some elements of the project from the requirements of ongoing IRB review may be administratively beneficial and doing so allows some of the research to get approved and commence before all details of an evaluation are finalized. Similar strategies may be used when projects involve elements of QI. An IRB may also request that projects be split into separate components.
Considerations for writing the protocol
The following sections contain guidance for how to write the research protocol to avoid confusion and maintain a positive relationship with an IRB, but these points also relate to important design considerations for how to design an ethical and feasible randomized evaluation that fits a particular context. For more guidance on these points from a research design perspective consult the resource on randomization and the related download on real-world challenges to randomization and their solutions.
Use clear language and default to overexplaining
IRB members reviewing your protocol are likely not experts in your field. Explain your project so that someone with a different background can understand what you are proposing. If you have co-investigators who have previously submitted to hospital IRBs, consult with them on developing the language for your protocol. You should also consult implementing partners in order to sufficiently describe the details of your intervention. Because IRBs only know as much as you tell them, it is important to be diligent when explaining your research. By being thorough and anticipating questions in the protocol, you are leaving less ground for confusion and questions that may arise and elongate the process of review and approval. Questions from the IRB aren’t necessarily bad or indicative that you won’t receive approval, however providing ample explanation is a way to proactively answer questions that may come up during review, therefore potentially saving time when waiting to hear back about the determination.
Be mindful of jargon and specific terminology
The IRB may imbue certain words with particular meaning.
- “Preparatory work,” for example, may be interpreted as a pilot or feasibility study and the IRB may instruct you to modify your application and specify that your study is a pilot in the protocol and informed consent. If you are not actually conducting a pilot, this can lead to confusion and delay.
- Similarly, mentioning “algorithms” may suggest an investigation of new algorithms to determine a patient’s treatment, prompting an IRB to review your work like it was designed to test a medical device.
- In the medical world, IRBs often refer to research with historical data as retrospective chart review. Chart review is research requiring only administrative data — materials, such as medical records, collected for non research purposes — and no contact with subjects. Chart reviews can be retrospective or prospective, although many IRBs will grant exemptions only to retrospective chart reviews. Prospective research raises the question of whether subjects should be consented, and prospective data collection may require more identifying information to extract data. Proposals therefore require additional scrutiny.
- If working only with data where the “identity of the human subjects cannot readily be ascertained” (including limited data sets subject to a DUA) these studies can be determined exempt under category 4(ii) (45 CFR 46.104). If chart review involves data that is identified, this still qualifies for expedited review (category #5) if minimal risk. In this case researchers are trading off a longer IRB process for greater freedom with how to code identifiers.
IRBs may provide glossaries or lists of definitions that are helpful to consult when writing a protocol. NIA also maintains a glossary of clinical research terms.
Carefully consider and explain the randomization approach.
Typical justifications for randomization are oversubscription (i.e., resource constraints or scarcity), where demand exceeds supply, or equipoise. Randomization given oversubscription ensures a fair allocation of a limited resource. Equipoise is defined as a “state of genuine uncertainty on the relative value of two approaches being compared.” In this case, randomization for the sake of generating new knowledge is ethically sound.
Careful explanation of the motivations behind randomization and the approach are crucial in making sure that both the IRB and participants understand they are not being deprived of care that they would normally be entitled to.
- In Health Care Hotspotting, eligible patients were consented and randomized into an intervention group that was enrolled in the Camden Core Model and a control group that received the usual standard of care (a printed discharge plan). Great care was taken to explain to patients that no one would be denied treatment that they were otherwise entitled to as a result of participating or not participating in the study. Recruiters emphasized that participation in the study would not affect their care at the hospital or from their regular doctors. However, the only way to access the Camden program was through the study and randomization. More detail on this intake process is in the resource designing intake and consent processes in health care contexts.
Different design decisions may reduce concerns about withholding potentially valuable treatments. Strategies such as phasing in the intervention, so all units are eventually treated but in a random order; randomizing encouragement to take up the intervention rather than randomizing the intervention itself; and randomizing among the newly eligible when expanding eligibility can all be considered during study design. For more details on these strategies, refer to this resource on randomization.
Explain risks and benefits
Behavioral interventions may not pose the risk of physical harm that drug or medical interventions might, however social scientists still must consider the risks participants face. Using data (whether administrative or collected by researchers) involves the risk of loss of privacy and confidentiality, including the possibility of reidentification if anonymized data is linked back to individuals. The consequences of these risks depend on the data content, but health data is generally considered sensitive. Risks beyond data-related ones do exist and must be considered. Are there physical, psychological, or financial risks that participants may be subjected to?
Do not minimize the appearance of risks. Studies are judged on whether risks are commensurate with the expected benefits. They need not be risk free. Being thoughtful in the consideration of risks is more effective than asserting their absence.
Don’t outsource your ethics; as the researcher, you have an obligation to consider risks and whether they are appropriate for your study. Researchers should ensure that they consider a study to be ethical before they attempt to make the case to someone else like an IRB. Many but not all behavioral studies will be minimal risk. Minimal risk does not mean no risk, but rather a level of risk comparable to what one would face in everyday life (45 CFR 46. 102(i)). Such risks, for example, may include taking medication that has the risk of side effects. Minimal risk studies may be eligible for expedited review, as detailed in this guidance set forth by the Office for Human Research Protections (OHRP). If you do not plan to use a medical drug or device intervention in your research, clearly distinguishing your study from medical research may be helpful in ensuring that your research is reviewed commensurate with risks. If you have a benign behavioral intervention* that is no more than minimal risk to participants, use those terms and justify your reasoning (*please see section 8. in Attachment B - Recommendations on Benign Behavioral Intervention for a more detailed definition of what constitutes a benign behavioral intervention). This will put your study in a shared framework of understanding. Check your IRB’s website for a definitions page and contact your IRB if you have any clarifying questions.
- In Encouraging Abstinence Behavior in a Drug Epidemic: Does Age Matter?, the intervention is administered using an app for a mobile device. Through the app, participants will receive varying incentive amounts for drug negative saliva tests. Participants must have a smartphone with a data plan in order to run the application. There is a potential risk of participants incurring extra charges on their phone plan if they exceed their data limit while uploading videos to the app. To address this risk, researchers made sure that the option in the app settings to “Upload only over Wi-Fi” was known to participants so that they can upload their videos without using mobile data and risking extra charges.
There is no requirement that your study must pose no more than minimal risk. Studies that are greater than minimal risk will face full institutional review board review. You must also explain the measures you plan to take to ensure that protections are put into place for the safety and best interests of the participants.
All studies must report adverse or unanticipated events or risks. An example of an adverse event reporting form can be found here (under “Protocol Event Reporting Form”). However, for studies that are greater than minimal risk, your IRB may ask you to propose additional safety monitoring measures. It is possible that hospital IRBs with a history of reviewing proposals for clinical trials are more accustomed to the need for safety monitoring plans and may expect them even from social science trials that do not appear risky.
- In Health Care Hotspotting, where the only risk was a breach of data confidentiality, weekly project meetings involved a review of data management procedures with staff as well as discussion of any adverse events. The hospital IRB submission prompted researchers to detail these plans.
Additionally, depending on the level of risk and funder requirements, you may encounter the need for a Data and Safety Monitoring Board (DSMB) to review your study. A DSMB is a committee of independent members responsible for reviewing study materials and data to ensure the safety of human subjects and validity and integrity of data. National Institutes of Health (NIH) policy requires DSMB review and possible oversight for multi-site clinical trials and Phase III clinical trials funded through the NIH and its Institutes and Centers. The policy states that monitoring must be commensurate with the risks, size, and complexity of the trial. For example, a DSMB may review your study if it involves a vulnerable population or if it has a particularly large sample size. The DSMB will make recommendations to ensure the safety of your subjects or possibly set forth a frequency for reporting on safety monitoring (e.g., submit a safety report every six months). Be sure to check your funder’s requirements and talk to your IRB about safety monitoring options if you think your study may be greater than minimal risk.
Address the inclusion of vulnerable populations
The Common Rule (45 CFR 46 Subparts B, C, and D) requires additional scrutiny of research on vulnerable populations, specifically pregnant people, prisoners, and children. This stems from concern around additional risks to the person and fetus (in the case of pregnancy) and the ability of the individual to voluntarily consent to research (in the case of those with diminished autonomy or comprehension).
The inclusion of vulnerable populations should not deter you from conducting research, whether they are the population of interest, a subgroup, or included incidentally. Although it may seem easier to exclude vulnerable individuals, relevant statuses such as pregnancy may be unknown to the researcher and unnecessary exclusions may limit the generalizability of findings. In addition to generalizability of findings, diversifying your research populations is important to avoid overburdening the same groups of participants. If you are considering the inclusion of vulnerable populations in your research, brainstorm safeguards that can be implemented to ensure vulnerable populations can safely participate in the research if there are additional risks. In some cases (including if funders require), you may need a Data Safety and Monitoring Board (DSMB) to review your research and provide safety recommendations.
- One J-PAL affiliated researcher proposed a study with pregnant women as the population of interest. Although the researcher was working with a vulnerable population, the study itself was a benign behavioral intervention involving text messages and reminders of upcoming appointments. Thorough explanation of the research activities and how the vulnerable population faced no more than minimal risk led to the study being approved without a lengthy review process.
-
In the evaluation of clinical decision support, researchers argued that it was important to understand the impact of CDS on advanced imaging ordering by providers for all patients, including vulnerable populations. Although not explicitly targeted for the study, vulnerable populations should not be excluded from having this benefit. Furthermore, the researchers noted that identifying vulnerable populations for the purposes of exclusion would result in an unnecessary loss of their privacy.
Consider the role of patients even if they are not the research subjects
In a hospital setting, patient considerations will be paramount, even if they are not the direct subject of the intervention, unit of randomization, or unit of analysis. It therefore behooves researchers to address them directly, even if they are not research subjects.
- In the evaluation of clinical decision support (CDS), healthcare providers were randomly assigned to receive the CDS. The provider was the unit of randomization and analysis, and the outcome of interest was the appropriateness of images ordered. The IRB considered potential risks to patients in terms of data use and patient welfare. The researchers proposed and the IRB approved a waiver of informed consent based on the following justification: the CDS could be overridden, ensuring providers maintained professional discretion in which images to order, which contributed to a determination of minimal risk to the patients. Further, obtaining consent from patients would not be practicable, and may have increased risk by requiring the collection of personally identifiable information that was not otherwise required for the study. You can learn more about activities requiring consent and waivers in this research resource.
These considerations boil down to communication of details and proper explanation in the protocol.
Conclusion
While social scientists may be initially hesitant, collaborating with hospitals to conduct randomized evaluations is a great way to increase the number of RCTs conducted in health care delivery research. Understanding levels of risk, writing a thorough protocol, strategizing for effective collaboration, and considering the role of quality improvement (QI) are important when planning for a submission to a hospital IRB.
Acknowledgments: This resource was a collaborative effort that would not have been possible without the help of everyone involved. A huge amount of thanks is owed to several folks. Thank you to the contributors, Laura Feeney, Jesse Gubb, and Sarah Margolis; thank you to Adam Sacarny for taking the time to share your experiences and expertise; and thank you to everyone who reviewed this resource: Catherine Darrow, Laura Feeney, Amy Finkelstein, Jesse Gubb, Sarah Margolis, and Adam Sacarny.
Creation of this resource was supported by the National Institute On Aging of the National Institutes of Health under Award Number P30AG064190. The content is solely the responsibility of the authors and does not necessarily represent the official views of the National Institutes of Health.
- Research Resource on Institutional Review Boards (IRBs)
- Research Resource on Ethical conduct of randomized evaluations
- Research Resource on Define intake and consent process
- The Common Rule - 45 CFR 46
- The Belmont Report
- OHRP guidance on considerations for regulatory issues with cluster randomized controlled trials
- SACHRP Recommendations: Attachment B - Recommendations on Benign Behavioral Intervention
- OHRP guidance on engagement of institutions in human subjects research
- OHRP FAQs on quality improvement activities
Abramowicz, Michel, and Ariane Szafarz. 2020. “Ethics of RCT: Should Economists Care about Equipoise?.” In Florent Bédécarrats, Isabelle Guérin, and François Roubaud (eds), Randomized Control Trials in the Field of Development: A Critical Perspective. https://doi.org/10.1093/oso/9780198865360.003.0012
Finkelstein, Amy. 2020. “A Strategy for Improving U.S. Health Care Delivery — Conducting More Randomized, Controlled Trials.” The New England Journal of Medicine 382 (16): 1485–88. https://doi.org/10.1056/nejmp1915762.
Finkelstein, Amy, and Sarah Taubman. 2015. “Randomize Evaluations to Improve Health Care Delivery.” Science 347 (6223): 720–22. https://doi.org/10.1126/science.aaa2362.
Horwitz, Leora I., Masha Kuznetsova, and Simon Jones. 2019. “Creating a Learning Health System through Rapid-Cycle, Randomized Testing.” The New England Journal of Medicine 381 (12): 1175–79. https://doi.org/10.1056/nejmsb1900856.
Checklist for social scientists publishing in medical journals: before, during, and after running an RCT
Summary
This resource is intended for researchers in the social sciences who are considering publishing their randomized evaluation in a medical journal. Publishing in a medical journal allows researchers to share their study with researchers and practitioners in different disciplines and expand the reach of their findings to those outside of their immediate professional network. This resource highlights journal guidelines and requirements that may be unfamiliar to those used to publishing in the social sciences. Most medical journals are members of the International Committee of Medical Journal Editors (ICMJE) and follow its recommendations and requirements for publishing.1 Some of the requirements for publishing necessitate action before a study has even started. While most of the content in this checklist is relevant across all medical journals, certain elements may differ from journal to journal. Researchers are encouraged to consult requirements listed in this resource in conjunction with the requirements of their specific journal(s) of interest, as well as requirements from funders and universities that could influence the publication process.
Key steps for publishing a randomized evaluation in a medical journal:
- Before participant enrollment begins, register a trial in an ICMJE compliant registry such as clinicaltrials.gov. Failure to do so may prevent publication in most medical journals. Note that the American Economic Association (AEA) registry is not an ICMJE compliant registry.
- Upload a statistical analysis plan (SAP) to the trial registry prior to the start of the study. Although not strictly a requirement for most medical journals, publically archiving a SAP in advance will allow greater flexibility in presenting and interpreting causal results. In particular, ensure primary vs. secondary outcomes and analysis methods are prespecified in the SAP.
- Draft a manuscript according to journal requirements. Medical journals require papers to contain a limited number of figures and tables and adhere to specific formats and short word counts. Consult the Consolidated Standards of Reporting Trials (CONSORT) guidelines used by most journals as well as individual journal requirements before drafting your manuscript.
- Be aware of embargo and preprint policies. Some journals may de-prioritize study publication if study results are public or shared through a working paper or preprint. However, other journals publicly state they will not penalize such papers. Journals also impose restrictions about what can be shared after acceptance and prior to publication. Make sure to check the journal’s specific guidelines and adhere to these rules.
1. Before starting an RCT
Register the trial before enrollment begins
The ICMJE defines a clinical trial as, “any research study that prospectively assigns human participants or groups of humans to one or more health-related interventions to evaluate the effects on health outcomes.” If a randomized evaluation meets the definition of a clinical trial, researchers must register it in an ICMJE compliant registry before the first participant is enrolled. If researchers fail to meet this condition, they risk having their paper rejected by medical journals. Note that registration on the AEA’s RCT Registry (supported by J-PAL) does not meet this requirement.
Not every randomized evaluation that researchers submit to a medical journal will meet the ICMJE definition of a clinical trial and require registration. However, if it is unclear whether a study meets the definition of a clinical trial, follow the registration guidelines for clinical trials as a cautionary measure. This way if a journal editor feels that a study is a clinical trial, researchers will have met the registration requirement.
Some scenarios where registration prior to enrollment may be unnecessary (although different journals may reach different conclusions) include the following:
- Secondary analysis of a clinical trial does not need to be registered separately. Instead, researchers should reference the trial registration of the primary trial.
- Example: In Health Care Hotspotting, researchers conducted a randomized evaluation of a care transition program’s impact on hospital readmissions for those with complex needs. In a related secondary study, the same research team is analyzing the effect of the program on outpatient utilization. Researchers referenced the original study’s registration instead of separately registering the secondary analysis.
- Analysis of a clinical trial implemented by someone else without researcher involvement is also considered a secondary analysis and does not need to be registered separately. Researchers may reference the existing clinical trial record, if one exists. As a best practice, researchers may also register a secondary analysis separately.
- Example: In The Spillover Effects of a Nationwide Medicare Bundled Payment Reform, independent researchers conducted a secondary analysis of a randomized evaluation implemented by the Centers for Medicare and Medicaid Services (CMS). This trial did not have an existing registration, so the researchers created one for their analysis. The trial was registered prior to analysis but after enrollment had begun.
- Evaluations of health care providers where the study population is made up solely of health care providers and the outcomes of interest include only effects on providers (rather than patients or community members), do not need to be registered. In this case, researchers may still consider registering the trial to avoid any barriers to publication if a journal editor disagrees with their assessment. Regardless, if the trial evaluates the effects of the provider intervention on patient outcomes then the trial should be registered.
- Example: In a clinical trial evaluating an educational intervention's impact on physical therapists’ prescribing behavior, registration is not required because outcomes are measured at the provider level (see case study example three in Hinman 2015).
More information on trial registration, including J-PAL requirements, can be found in this resource on trial registration. Information on labeling a journal submission that is not a clinical trial is discussed below.
Develop a trial protocol
A trial protocol outlines the objectives, methodology, study procedures, statistical analysis plan (SAP), and ethical considerations of a study. This is the same protocol that researchers must submit to an institutional review board (IRB) prior to beginning human subjects research. Medical journals require the trial protocol to be submitted at the time of manuscript submission. Because IRBs may vary in what information they request, researchers should use the SPIRIT reporting guidelines when preparing protocols to ensure completeness. An example is the trial protocol (with an integrated SAP) for Health Care Hotspotting.
Researchers should plan to submit the original approved protocol along with all amendments and their rationale. Amendments should always be reviewed and approved by an IRB prior to taking effect. Significant changes to the original protocol should be described in any future publications. The trial protocol is typically included with a publication as an online supplement.
Develop a statistical analysis plan (SAP)
A statistical analysis plan (also known as a pre-analysis plan) provides detailed information on how data will be coded and analyzed. A SAP can be integrated into a trial protocol or may exist as a separate document. Most medical journals require a SAP to be submitted along with the manuscript.
Unlike trial registration, journals differ on when a SAP must be completed. However, for some journals, completing a SAP prior to the launch of the intervention, or at least prior to receiving data and beginning analysis, can increase the credibility of findings and/or allow researchers greater freedom in using causal language when interpreting results. Defining analysis plans in advance also reduces concerns about specification searching (p-hacking), particularly when there is researcher discretion about what outcomes to consider and when and how they are measured. Most medical journals will require researchers to indicate which analyses are prespecified and which are post hoc. As with the trial protocol, the final SAP should be submitted to the journal, with any changes to original plans tracked and justified.
J-PAL does not universally require SAPs, but many individual J-PAL initiatives and funders require that an analysis plan be completed prior to the launch of an evaluation. For example, all J-PAL North America funded projects must upload a SAP to the AEA RCT Registry. See this resource on pre-analysis plans for more detailed information and recommended practices.
Guidelines for the Content of Statistical Analysis Plans in Clinical Trials provides a checklist that researchers can follow to help structure their SAPs. A detailed explanation of each section can be found in Appendix 2 of the article. Some important topics to cover in a SAP include:
Outcomes
Ensure primary and secondary outcomes are prespecified. Defining a single primary outcome aids in interpretation of the overall result of the research, particularly if multiple outcomes yield different results. The primary outcome should be one that the study is well powered to detect, whereas important outcomes that researchers may be less confident observing effects for can be listed as secondary outcomes. For example, in medical research a short term clinical endpoint may be selected as a primary outcome, with mortality selected as a secondary outcome. Include details on how and when outcomes will be measured, their units, and any calculations or transformations made to derive outcomes.
- Example: Prespecifying a primary outcome may be unfamiliar to social scientists. In Mandatory Medicare Bundled Payment Program for Lower Extremity Joint Replacement and Discharge to Institutional Postacute Care, researchers chose to measure discharges to postacute care as the primary outcome of a bundled payment model on lower extremity joint replacements. Prior observational studies suggested that discharges to postacute care would be the main outcome to change as a result of the bundled payment model, and power calculations confirmed that a reasonable effect size could be detected. Researchers analyzed secondary outcomes to measure additional impacts of the bundled payment model, including spending, the number of days in postacute care, changes to quality of care, and patient composition.
Planned analyses
Include statistical models, any plans for covariate adjustment, and additional analysis beyond the intention to treat effect, such as local average treatment effect (LATE), and approaches to noncompliance or partial take-up.
Missing Data
Outline how missing data will be dealt with and reported. Attrition due to imperfect matches in administrative data and survey non-responses are both situations where researchers will need to plan to address missing data. Medical journals often have strong opinions about how to handle missing data and may request sensitivity analysis or multiple imputation even when missingness is low. Defining an approach in advance will reduce concerns about specification searching. The plan may include flexibility depending on the severity of missing data. See the Journal of the American Medical Association's (JAMA) guidelines on missing data and the New England Journal of Medicine's (NEJM) recommendations for handling missing data.
- Example: In Health Care Hotspotting, even though match rates to administrative data were greater than 95 percent, researchers were asked to include an analysis using multiple imputation as a sensitivity check.
Multiplicity
Testing multiple hypotheses increases the probability of false positive results. Therefore, researchers should have a plan to address multiple testing when designing an evaluation. Absent a prespecified approach, journals may treat results for secondary outcomes as only exploratory. The multiple hypothesis testing section of this resource on data analysis has suggestions on statistical corrections when examining multiple outcomes.
- Example: In Health Care Hotspotting, because researchers did not address multiplicity in their SAP, they were asked to remove p-values from all but the primary outcome and include the following caveat about interpreting secondary outcomes: “There was no prespecified plan to adjust for multiple comparisons; therefore, we report P values only for the primary outcome and report 95 percent confidence intervals without P values for all secondary outcomes. The confidence intervals have not been adjusted for multiple comparisons, and inferences drawn from them may not be reproducible.”
2. While implementing an RCT
Medical journals typically require significant detail on how participants progress through an evaluation. During an evaluation’s implementation stage, researchers should keep track of participants’ study involvement, the mechanics of randomization, and fidelity to the study protocol.
Track participants throughout the evaluation
Researchers should track the flow of participants as they move through each stage of the trial and should report this in their paper. The Consolidated Standards of Reporting Trials’ (CONSORT) Statement, a minimum set of recommendations for reporting RCTs, recommends using this diagram (see Figure 2) to display participant flow. All trials published in medical journals include this CONSORT flow diagram, and one should always be included as a figure in your paper (typically the first exhibit). See an example of a CONSORT flow diagram below.
At a minimum, researchers should track the number of individuals randomly assigned, the number who actually receive the intervention, and the number analyzed. Information on individuals assessed for eligibility prior to randomization can be included if available. Any additional information on exclusions or attrition (also known as loss to follow-up) should be included as well.
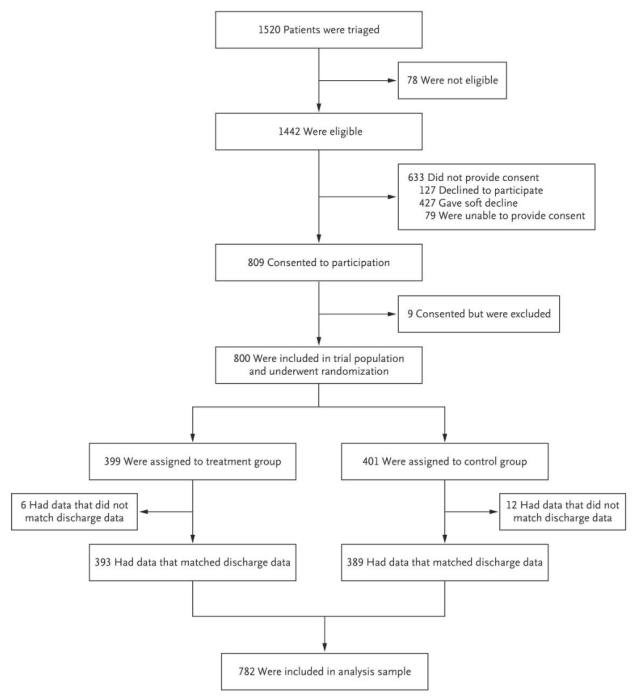
Source: Finkelstein et al. 2020
Track the mechanics of randomization
Researchers should keep track of the randomization process and the mechanics of implementation, as these details must be reported in detail in a manuscript. This typically includes the type of randomization procedure — was randomization stratified, done on the spot or from a list — as well as who generated random assignments, how the information was concealed or protected from tampering, and how information was revealed to participants. Items 8-10 of the CONSORT checklist cover reporting on randomization processes. See Financial Support to Medicaid-Eligible Mothers Increases Caregiving for Preterm Infants for an example of reporting these details in a manuscript.
Monitor implementation fidelity
The CONSORT statement notes that “the simple way to deal with any protocol deviations is to ignore them.” This is consistent with the intention to treat approach to randomized evaluation. However, providing detail is helpful to contextualize and interpret results. See the resource on implementation monitoring and real-time monitoring and response plans for guidance. Researchers approach monitoring implementation and reporting on it in different ways.
- In Health Care Hotspotting, researchers quantitatively tracked program metrics in the treatment group and reported them in a table.
- In Effects of a Workplace Wellness Program on Employee Health, Health Beliefs, and Medical Use, researchers reported adherence by presenting completion rates for screenings and other elements of the intervention, as well as control group awareness of the intervention.
3. After completing an RCT
Clinical trial reporting in medical journals follows a specific style characterized by consistent structure, short word counts, and a small number of tables and figures. The Consolidated Standards of Reporting Trials’ (CONSORT) Statement provides a set of standardized recommendations, in the form of a checklist and flow diagram, for reporting randomized evaluations in medical journals. Researchers should consult the CONSORT Statement, as well as particular journal requirements, in order to ensure a manuscript is properly formatted and structured. This section highlights elements of analysis and manuscript preparation that may be less familiar to social scientists.
Adhere to medical journal analysis norms
CONSORT guidelines state that researchers should not report statistical tests of balance in participant characteristics, and many medical journals follow this recommendation. This practice differs from a common norm in economics to report balance tests in summary statistics tables. Researchers can find more information on the logic behind this rule in the CONSORT Explanation and Elaboration resource.
Results should be reported for all primary and secondary outcomes, regardless of their statistical significance. Detailed results should be reported for each outcome, typically mean levels for each group, treatment effect, confidence interval, and p-value. Confidence intervals are preferred to standard errors. For binary outcomes, both absolute and relative effects should be reported. Researchers should make clear which analyses are prespecified, particularly in the case of subgroup analyses.
Below is an example of a results table with multiple outcomes from Health Care Hotspotting. Each row highlights one outcome, with mean levels for each group, an unadjusted group difference (from a regression without controls), an adjusted group difference (from a regression with controls), and corresponding confidence intervals. In this case, all analyses were prespecified and p-values were omitted.
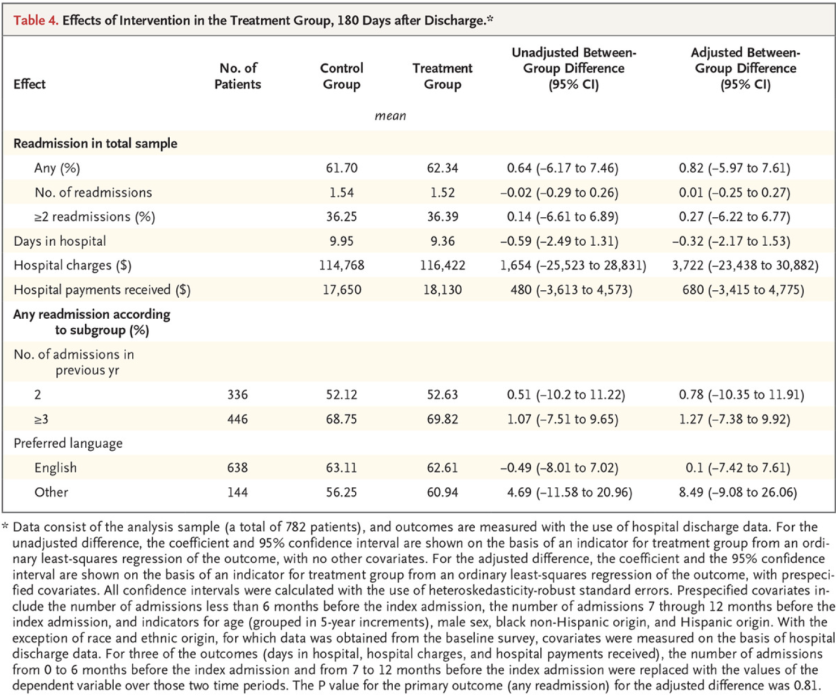
Source: Finkelstein et al. 2020
Construct tables and figures
Journals typically cap the total number of exhibits (tables and figures) at five. Figure 1 should typically be the participant flow diagram. A summary statistics table, typically Table 1, should always describe baseline demographic and clinical characteristics for each group. The remaining figures and tables display the results of the evaluation.
For studies with multiple treatment arms or subgroup analyses, it may be helpful to use an exhibit with multiple panels or a hybrid exhibit that combines a table and a figure to provide more detail. Figure 2 in Effectiveness of Behaviorally Informed Letters on Health Insurance Marketplace Enrollment gives an example of a hybrid exhibit. Note, however, that some journals may not view this as a single exhibit. Additional exhibits may be included in a supplemental appendix.
Prepare your manuscript with the correct structure
Before preparing a manuscript for a medical journal, you should consult the specific word and exhibit limits of that journal as well as its requirements on how the manuscript should be structured. Compared to social science publications, reports of clinical trials in medical journals are consistently structured and short (e.g., 3000 words) with binding limits on the number of words and exhibits. Medical journals are generally much more prescriptive about what each section in the paper must accomplish. Medical journals do allow for supplemental appendices where researchers can include details that did not fit in the main body of the paper. Most journals follow CONSORT reporting requirements. Consult the CONSORT checklist as you write and make sure to include all sections. Some journals require submission of the checklist to ensure that all elements have been addressed.
Some key requirements from the CONSORT checklist have been highlighted below:
Title
Include ‘randomized’ in the manuscript title so that databases properly index the study and readers can easily identify the study as a randomized trial.
Abstract
The abstract should be framed as a mini paper, with clear headings, and include a sufficient summary of the work, including results and interpretation. The abstract is not a preview that raises questions to be answered in the main text. Effort should be focused here because this is the first section that will be reviewed and often the only section that many readers will read. A full list of what to include can be found within Item 1b. of the CONSORT Statement. Trial registration information (registry name, trial ID number) should be listed at the end of the abstract.
Methods
Despite the short length of medical journal articles, the methods section must include a high level of detail. Required details include mechanisms of random assignment, power calculations, intake and consent procedures, where IRB approval was granted, statistical analysis methods, and software packages used.
Results
Participant flow and baseline characteristics should be described first, followed by the effects of the intervention. Results are presented in text with similar detail to the tables discussed above (i.e., control and treatment group means, effect sizes, confidence intervals). The results section presents results without interpretation, which is reserved for the discussion section.
Discussion
The discussion section offers the opportunity to discuss limitations and generalizability, and provide additional interpretation. This section is where researchers can discuss and weigh the sum total of the evidence if results across outcomes are mixed, or draw comparisons to results in other scholarship. This section also offers an opportunity to summarize and conclude, though information provided in other parts of the paper should not be repeated in detail. If a journal allows a separate conclusion section it is typically a single summary paragraph.
Researchers should ensure their paper is properly pitched for a medical or public health audience rather than an economics audience. Consulting the stated aims and scope of a journal can help with framing study results and emphasizing its relevance to the field. Highlighting the clinical rationale and patient care implications of a study can be helpful as medical journal readership is often interested in these factors. If possible, researchers should have someone who has frequently published in medical journals review their manuscript before submission to make sure the manuscript is properly framed for the intended audience.
Submit your manuscript for review and publication
There are some additional considerations to keep in mind at the time of submission:
Cover letter
Journals may require submission of a cover letter along with a manuscript. There are conflicting opinions on whether or not the cover letter is important. One view is that it needs to say nothing more than “Thank you for considering the attached paper.” Another view is that the cover letter is an opportunity to pitch the paper and explain why the findings are important and relevant to the readers of this journal. It can also be used to provide context for information included elsewhere in the submission, such as whether there are preprints of the paper or potential conflicts of interest.
Study type label
When submitting a paper to a journal, researchers typically select what type of study is being submitted. If the study is labeled as a clinical trial, journals will expect the study to have been registered prior to enrollment. If this was not the case, this may lead the paper to be automatically rejected. If the study is a secondary analysis of a clinical trial, make sure to specify this. Journals may list secondary analyses as a subcategory of clinical trials or may list them as a separate type. A detailed definition of clinical trials and considerations for trial registration are discussed in Section 1 of this resource.
Preprints and working papers
Journals may be reluctant to publish studies or results that are already available online. Distributing a manuscript as a working paper or uploading it to a preprint server may lead journals to de-prioritize publication of a paper. Researchers should confirm the policies at their target journal(s) prior to releasing a working paper. The BMJ, The Lancet, NEJM, and JAMA do not penalize studies that are already available as preprints as long as researchers provide information about the preprint during the submission process. Other journals may accept manuscripts only if they have been significantly revised after being released as a working paper.
Embargoes
Most journals impose embargoes where authors cannot disclose paper acceptance or share results until the work has been published. Most journals allow researchers to present at conferences, but may impose restrictions, such as prohibiting researchers from sharing complete manuscripts, and may request that researchers inform the journal of planned presentations. Embargoes will typically include a post-acceptance window where the media has access to upcoming publications, in order to conduct interviews and prepare articles that can be released once the manuscript is published.
Researchers should consult individual journals to ensure they are adhering to their specific embargo rules. Researchers working with implementing partners should ensure that everyone understands and can abide by the embargo policy, and both parties should work together to develop communication plans to take advantage of media access periods. This resource on communicating with a partner about results has additional details on embargo policies and communication plans. While embargoes may seem restrictive, medical journals tend to publish frequently, so the publishing timeline and embargo period can often move along quickly.
4. Examples of published work from J-PAL researchers
A selection of J-PAL affiliated-studies that have been published in medical journals has been highlighted below.
- Health Care Hotspotting — A Randomized, Controlled Trial (NEJM)
- Mandatory Medicare Bundled Payment Program for Lower Extremity Joint Replacement and Discharge to Institutional Postacute Care (JAMA)
- Financial Support to Medicaid-Eligible Mothers Increases Caregiving for Preterm Infants (Maternal and Child Health Journal)
- Effect of a Workplace Wellness Program on Employee Health and Economic Outcomes: A Randomized Clinical Trial (JAMA)
- Effects of a Workplace Wellness Program on Employee Health, Health Beliefs, and Medical Use: A Randomized Clinical Trial (JAMA Internal Medicine)
Acknowledgments: Thanks to Marcella Alsan, Catherine Darrow, Joseph Doyle, Laura Feeney, Amy Finkelstein, Ray Kluender, David Molitor, Hannah Reuter, and Adam Sacarny for their thoughtful contributions. Amanda Buechele copy-edited this document. Creation of this resource was supported by the National Institute On Aging of the National Institutes of Health under Award Number P30AG064190. The content is solely the responsibility of the authors and does not necessarily represent the official views of the National Institutes of Health.
1 The full list of journals that follow ICMJE recommendations can be found on ICMJE.org.
- The CONSORT statement provides information on the CONSORT checklist and flow diagram, as well as the CONSORT Explanation and Elaboration Document, which contains the rationale for items included in the checklist.
- The ICMJE website includes information about:
- JAMA has extensive instructions for authors, covering the requirements for reporting different types of research like clinical trials, including the handling of particular topics like missing data.
- J-PAL’s Research Resources provide additional information on several topics discussed in this resource, including:
- SPIRIT Checklist for Trial Protocols
Finkelstein, Amy, Annetta Zhou, Sarah Taubman, and Joseph Doyle. “Health Care Hotspotting — A Randomized, Controlled Trial.” New England Journal of Medicine 382, no. 2 (January 9, 2020): 152–62. https://doi.org/10.1056/NEJMsa1906848.
Finkelstein, Amy, Annetta Zhou, Sarah Taubman, Joseph Doyle, and Jeffrey Brenner. "Health Care Hotspotting: A Randomized Controlled Trial." AEA RCT Registry. (2014) https://doi.org/10.1257/rct.329.
Finkelstein, Amy and Jeffrey Brenner. “Health Care Hotspotting: A Randomized Controlled Trial.” Clinicaltrials.gov. (March 18, 2014). https://clinicaltrials.gov/ct2/show/NCT02090426.
Finkelstein, Amy, Yunan Ji, Neale Mahoney, and Jonathan Skinner. “Mandatory Medicare Bundled Payment Program for Lower Extremity Joint Replacement and Discharge to Institutional Postacute Care: Interim Analysis of the First Year of a 5-Year Randomized Trial.” JAMA 320, no. 9 (September 4, 2018): 892–900. https://doi.org/10.1001/jama.2018.12346.
Finkelstein, Amy, Yunan Ji, Neale Mahoney, and Jonathan Skinner. “The Impact of Medicare Bundled Payments.” Clinicaltrials.gov. (January 23, 2018) https://clinicaltrials.gov/ct2/show/NCT03407885
Gamble, Carrol, Ashma Krishan, Deborah Stocken, Steff Lewis, Edmund Juszczak, Caroline Doré, Paula R. Williamson, et al. “Guidelines for the Content of Statistical Analysis Plans in Clinical Trials.” JAMA 318, no. 23 (December 19, 2017): 2337–43. https://doi.org/10.1001/jama.2017.18556.
Hinman, Rana S., Rachelle Buchbinder, Rebecca L. Craik, Steven Z. George, Chris G. Maher, and Daniel L. Riddle. “Is This a Clinical Trial? And Should It Be Registered?” Physical Therapy 95, no. 6 (June 1, 2015): 810–14. https://doi.org/10.2522/ptj.2015.95.6.810.
J-PAL. “Health Care Hotspotting in the United States.” https://www.povertyactionlab.org/evaluation/health-care-hotspotting-united-states
J-PAL. “The Spillover Effects of a Nationwide Medicare Bundled Payment Reform.” https://www.povertyactionlab.org/evaluation/spillover-effects-nationwide-medicare-bundled-payment-reform
Reif, Julian, David Chan, Damon Jones, Laura Payne, and David Molitor. “Effects of a Workplace Wellness Program on Employee Health, Health Beliefs, and Medical Use: A Randomized Clinical Trial.” JAMA Internal Medicine 180, no. 7 (July 1, 2020): 952–60. https://doi.org/10.1001/jamainternmed.2020.1321.
Song, Zirui, and Katherine Baicker. “Effect of a Workplace Wellness Program on Employee Health and Economic Outcomes: A Randomized Clinical Trial.” JAMA 321, no. 15 (April 16, 2019): 1491–1501. https://doi.org/10.1001/jama.2019.3307.
Ware, James H., David Harrington, David J. Hunter, and Ralph B. D’Agostino. “Missing Data.” New England Journal of Medicine 367, no. 14 (October 4, 2012): 1353–54. https://doi.org/10.1056/NEJMsm1210043.
Yokum, David, Daniel J. Hopkins, Andrew Feher, Elana Safran, and Joshua Peck. “Effectiveness of Behaviorally Informed Letters on Health Insurance Marketplace Enrollment: A Randomized Clinical Trial.” JAMA Health Forum 3, no. 3 (March 4, 2022): e220034. https://doi.org/10.1001/jamahealthforum.2022.0034.