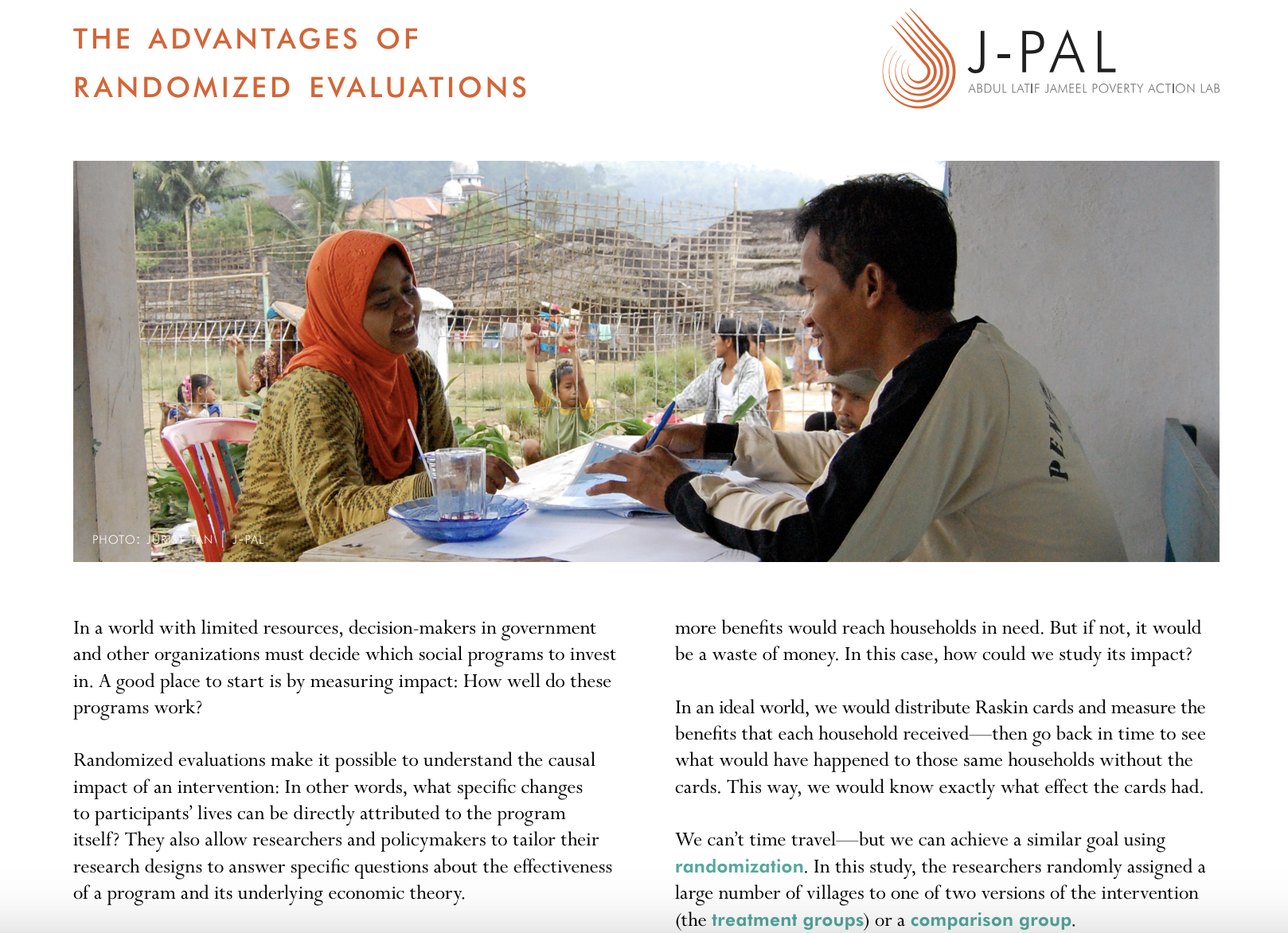
Introduction to randomized evaluations
Summary
This resource gives an overview and non-technical introduction to randomized evaluations. Randomized evaluations can be used to measure impact in policy research: to date, J-PAL affiliated researchers have conducted more than 1,100 randomized evaluations studying policies in ten thematic sectors in more than 90 countries. This resource highlights work from a variety of contexts, including studies on youth unemployment in Chicago, a subsidized rice program in Indonesia, and a conditional cash transfer in Mexico. It includes guidance on when randomized evaluations can be most useful, and also discusses when they might not be the right choice as an evaluation method.
Introduction
In recent years, randomized evaluations, also called randomized controlled trials (RCTs), have gained increasing prominence as a tool for measuring impact in policy research. The 2019 Nobel Memorial Prize in Economics, to J-PAL co-founders Abhijit Banerjee and Esther Duflo, and longtime J-PAL affiliate Michael Kremer, was awarded in recognition of how this research method has transformed the field of social policy and economic development.
Randomized evaluations are a type of impact evaluation method. Study participants are randomly assigned to one or more groups that receive (different types of) an intervention, known as the “treatment group” or groups, and a comparison group that does not receive any intervention. Researchers then measure the outcomes of interest in the treatment and comparison groups. Randomized evaluations make it possible to obtain a rigorous and unbiased estimate of the causal impact of an intervention; in other words, what specific changes to participants’ lives can be attributed to the program. They also allow researchers and policymakers to tailor their research designs to answer specific questions about the effectiveness of a program and its underlying theory of change. If thoughtfully designed and implemented, a randomized evaluation can answer questions such as: How effective was this program? Were there unintended side-effects? Who benefited most? Which components of the program work or do not work? How cost-effective was the program? How does it compare to other programs designed to accomplish similar goals?
J-PAL’s network of researchers works closely with governments, NGOs, donors, and other partners who are interested in using randomized evaluations to find answers to these questions.
Randomized evaluations in practice
Randomized evaluations can help answer policy-relevant questions that have the potential to affect millions of lives. In Indonesia in 2012, for example, a program called Raskin (now called Rastra) provided subsidized rice to low-income households. It reached 17.5 million people in 2012, but researchers estimated that eligible households saw only about one-third of the benefits they were entitled to receive, due to issues related to corruption and inefficiency.
A randomized evaluation by Banerjee et al. (2018)1 tested whether giving households identification cards that included information about their eligibility and program rules would help them access the program. If the Raskin cards worked, more benefits would reach households in need, but if not, the program would be a waste of money. In the ideal scenario, the researchers would be able to distribute Raskin cards and measure the benefits that each household received—then go back in time to see what would have happened to those same households without the cards. This way, they could determine exactly what effect the cards had.
Time travel is not possible—but randomization can achieve a similar goal. In this study, the researchers randomly assigned a large number of villages to one of two versions of the intervention (the treatment groups) or a comparison group. In its simplest sense, random assignment just means that a random device–such as a coin flip, a die roll, or a lottery–determines to which of the three groups a given village is assigned.
The randomized assignment to a treatment or comparison group is the key element of a randomized evaluation. Many of the technical details of how to actually create randomly assigned treatment and comparison groups are described in our Randomization resource.
Credible estimates of impact
An important advantage of randomized evaluations is that they help ensure that systematic differences between groups do not drive differences in outcomes. In other words, we can more confidently attribute the difference in outcomes to the intervention, rather than to other factors.
In our example, suppose instead that program officials had been able to choose which households received cards. Officials who were not following the program’s rules or who oversaw inefficient distribution operations might not want their citizens to receive information about the program, and may, therefore, be less likely to enroll their community. In this case, the communities with the most irregularities in rice distribution would not receive the cards, while communities where Raskin was already working well would get them. As a result, we might overestimate the impact of the intervention.
Random assignment solves this selection problem by ensuring the treatment and comparison groups are comparable. Of course, the two groups may still not be exactly the same. Any two villages assigned to treatment and comparison groups are likely to be different, leading to some chance variation between the different groups. However, as we add more observations (in our example, villages), the groups as a whole tend to become more similar to each other, and more similar to the population from which they are drawn. Statistical methods then allow us to gauge how likely it is that differences in outcomes we observe are due to the program being evaluated. With large enough samples, we can learn the true effect of the intervention with a high degree of confidence.
What kinds of questions can a randomized evaluation answer?
Randomized evaluations are particularly well suited to assessing how a social program works in a real-world setting. An important focus is often on human behavior and participants’ responses to the implementation of the program.
As a hypothetical example, consider a water delivery program in an area with contaminated water sources. How do we know whether this program is succeeding in its goal of reducing morbidity and mortality? This isn’t the same thing as asking a “lab science” type question, such as “Does lead exposure affect kidney function?” or “What toxins are ingested when using unfiltered water for cooking?” Those questions might be better answered in a laboratory or a controlled clinical study. This is also distinct from understanding whether a program is being implemented well, including whether the steps required for the program to work are occurring. Those questions might best be verified through careful process monitoring.
However, a key component that cannot be tested in a lab or controlled by the implementer is how households react when they receive water deliveries and are asked to use this water for cooking, drinking, and washing: they must consume the filtered and chlorinated water consistently, even though the food tastes differently and the process might require handling heavy water containers; and they must stop consuming untreated water or exposing themselves to toxins in other ways, even though households might be used to frequenting a nearby laundry service or snacking on fruit quickly rinsed under a tap.2 In all these contexts, a program evaluation can help determine whether behavior is successfully changed, and the program’s goals are achieved as a result.
In order to obtain an unbiased estimate of a program’s true impact, it is typically most useful to conduct an impact evaluation by implementing the program in the treatment group as closely as possible to the real-world intervention, in close cooperation between researchers and implementing organizations. However, in order to understand underlying mechanisms, such as the behavioral factors above, researchers might even decide to test the human behavior component directly, without implementing the full program. This could be achieved by setting up a temporary (and perhaps, for the time being, less efficient) distribution system to deliver water to households in the treatment group, solely for the purposes of the evaluation.
An important related benefit of randomized evaluations is that the intervention as well as the data collection can be tailored to answer specific questions. For example, it may be important to understand the individual impact of different components of a program and the channels through which they work.
In the Raskin example, researchers tested two variations on the ID card design, including creating a treatment group to test whether providing information about the official subsidized price of rice had an additional impact on the quantity of rice eligible households received and the price they paid. They found that households that received price information did not pay less—but they did receive higher quantities of subsidized rice. This suggests that the price information increased households’ bargaining power with local officials, some of whom were overcharging for rice before the cards were introduced. In part based on these results, the government decided to scale up social assistance identification cards to 15.5 million households across the country, reaching over 65 million people in 2013.
Randomized evaluations can also be used to understand the long-term effects of an intervention.3 Consider the Raskin example: the short-run effect of receiving more rice could in theory improve the nutrition of household members, which could potentially decrease their school absences or increase their working hours. Over time, these secondary short-run effects could accumulate into increased years of schooling or higher wages. Longer time horizons pose challenges while measuring long-term effects––for example, it is likely that external factors outside of the study will affect study participants, or researchers may have difficulty in locating participants. However, with periodic monitoring and measurement of intermediary outcomes, the long-run treatment effects can be credibly estimated due to the randomized aspects of the Raskin program. For more information, see Bougen et al.’s (2018) Using RCTs to Estimate Long-Run Impacts in Development Economics
Administrative data can be particularly useful for measuring long-run effects. As an example, J-PAL affiliated researchers used administrative tax data collected by the United States federal government to measure the long-run effects of the Moving to Opportunity program (MTO). The MTO program provided to treatment group households housing vouchers which could only be redeemed in neighborhoods with lower poverty rates. In the short-run, the program results were mixed: researchers showed that treatment group adults who moved had no increases in employment or income but did report feeling happier and safer in their new neighborhoods (Katz et al., 2001). However, in using administrative data to examine outcomes twenty years after the original intervention, researchers found that children in the treatment group households who moved experienced improvements in a range of outcomes in their adulthood relative to children in comparison group families. In particular, as adults they had higher incomes, were more likely to attend college, and less likely to live in neighborhoods with higher poverty rates (Chetty et al., 2016).
In another evaluation, J-PAL affiliated researchers periodically surveyed recipients of the Targeting the Ultra Poor (TUP) program in India to identify the long-run effects of the program and some potential channels through which they operated.4 A key feature of the TUP program is that treatment households receive a productive asset, such as livestock. The short-run effects of the program––increases in household consumption, wealth, and income––grew each year for the first seven years after the program was delivered, and then held steady in years 8-10. Researchers determined the long-run effects developed in part from treatment households using the income generated by their new livestock to create new businesses (Banerjee et al., 2020). More examples of studies evaluating the long-term effects of a program can be found on J-PAL’s Evaluations page.
When is a randomized evaluation the right tool?
Making use of policy windows
The value of rigorously evaluating a program or policy is particularly high when conducted at the right point in time to have an influence on policy.
Take the example of Mexico, a country that has experienced steady economic growth and is today an upper-middle income country, albeit with still high levels of inequality. In the process of increasing economic development, many countries begin implementing national social protection programs, and Mexico is no exception. The Mexican program PROGRESA (later called Oportunidades, then PROSPERA), a conditional cash transfer program launched in 1997, is an example of a well-timed randomized evaluation that had lasting policy impact. The policy gave mothers cash grants for their family, as long as they ensured their children attended school regularly and received scheduled vaccinations. PROGRESA was remarkable partly because its external randomized evaluation helped the program survive a change in political leadership in the presidential elections in 2000. Anticipating the possibility that incumbent social programs would be dismantled, then president Ernesto Zedillo had initiated the evaluation in order to depoliticize the decision about PROGRESA and demonstrate the policy’s effectiveness in improving child health and education outcomes (Behrman, 2007).
PROGRESA was first introduced as a pilot program in rural areas of seven states. Out of 506 communities sampled by the Mexican government for the pilot, 320 were randomly assigned to treatment and 186 to the comparison group. Children in the treatment group were enrolled in school longer and were healthier (measured by illness incidence and growth) than children in the comparison group (Gertler and Boyce, 2003). Based on the findings, the new government expanded the program significantly to urban areas, and the program was soon replicated in other countries, such as Nicaragua, Ecuador, and Honduras. Following Mexico’s lead, these countries conducted pilot studies to test the impact of PROGRESA-like programs on their populations before scaling up. By 2014, 52 countries had implemented PROGRESA-like programs (Lamanna, 2014).
By contrast, a randomized evaluation may not be the right tool when external factors are likely to interfere with the program during the randomized evaluation, or the program is significantly altered under evaluation conditions.
Unlike laboratory experiments, randomized studies for policy evaluation are not isolated from general environmental, political, and economical factors. External factors may arise which lessen our confidence in the generalizability or transferability of our research findings. For example, studying the impact of microfinance or workforce development programs in the midst of a major recession could distort the findings significantly. A changing policy environment may also make a randomized evaluation difficult, for example because government representatives are unwilling to commit to leaving the program unchanged, or because a non-governmental organization is ramping up a similar program as the one being studied and making it available to the comparison group, while the evaluation is ongoing. All these factors need to be considered in the planning phase of a randomized evaluation.
Informing scale-up and design
A randomized evaluation can be particularly valuable when a lot of resources are being invested in a new program, but we do not yet have evidence about its impacts.
In this context, a randomized evaluation can inform decisions about scaling up the program. For example, consider the summer jobs program One Summer Chicago Plus (OSC+), which partners with local community organizations to place youth in nonprofit and government jobs (such as camp counselors, community garden workers, or office assistants). Youth employment programs such as OSC+ had been used in the United States for decades to address issues such as high youth unemployment and crime rates, yet there was little evidence on their effects. (Gelber et al., 2014).
A randomized evaluation of the 2012 cohort of the OSC+ program showed that it led to a 43 percent reduction in arrests for violent crimes among youth who received the summer employment offer (Heller, 2014). The evidence that a program is effective can help make the case to allocate resources towards expanding the program: In 2015, the Inner City Youth Empowerment, LLC donated the funds necessary for Chicago to expand OSC+ to double the number of youth, citing the evidence from the evaluation as a compelling factor motivating their investment.5
Researchers can also use tailored research designs to learn about the effects of ongoing programs, and use the results to gain a deeper understanding of program effects or finetune the program design. For example, the expansion of OSC+ allowed the city of Chicago and researchers to build on the original evaluation and study whether the program’s impacts varied for different subgroups, such as for male students compared to female students, or Hispanic students compared to non-Hispanic students.
Often, researchers can also use the roll-out phase to learn more about the key mechanisms driving the program’s impact through research designs such as a randomized staggered design, (where treatment is periodically extended to a randomly selected proportion of the sample), or, if initial take-up is low, a randomized encouragement design (where people are randomly chosen to receive encouragement or support to take up a program). Evaluating a program during its roll-out phase can be an opportunity to improve its design by experimentally varying aspects of the program. If different models of the program–or, the program as a whole–prove to not be as effective as expected, then the program providers can decide to invest resources in other, more successful models. See our How to Randomize lecture for more information on different methods of randomization that take advantage of the natural process of ramping up or rolling out a program.
It is important to note that all randomized evaluations require a sufficiently large enough sample size to detect any impact. When the sample is too small, natural variation in the outcome of interest may not allow us to conclusively measure the impact of the intervention: we are not able to tell if the difference between treatment and comparison group is a result of the program or pure chance. Conducting a randomized evaluation in this situation would unnecessarily burden study participants and use up resources that could be used more productively elsewhere. See J-PAL North America’s resource on the risks of underpowered evaluations for more information.
Relatedly, a decision parameter should be whether the evidence from the randomized evaluation will actually be able to change how policies are made or how the program is administered. If this is not the case, or if the expected learning does not justify the investment, it may not be reasonable to conduct a randomized evaluation. For example, if the cost of data collection is so high that it uses up most of the program budget, or if the time it takes to evaluate means that the program itself will likely end before results are seen, it may not be useful to conduct an evaluation (unless the results can also inform future programs). This point is closely related to the discussion on research ethics below: a study should only be undertaken if the benefits of the research actually outweigh the costs.
Learn more about the PROGRESA, Raskin, and OSC+ programs below:
The Impact of PROGRESA on Health in Mexico
Real-world challenges to randomization
While randomized evaluations can be excellent tools for learning about the impact of a policy or program, they are not appropriate for all situations. In the following examples, clever research design can often be used to mitigate challenges to randomization, but in some cases, a randomized evaluation may simply not be the right choice. For more information, see also J-PAL North America’s guide to Real-World Challenges to Randomization or our How to Randomize lecture (slides below).
Policies that affect whole groups or even countries
Randomization becomes more difficult when the group that is affected by a program is potentially large. At the extreme end of the spectrum are policies that are implemented centrally at the national or even supra-national level, such as a monetary policy regulating the money supply. Here, randomization is not possible because the sample has a size of one–the country with the central bank that is instituting the policy – and no counterfactual is available where a different policy is introduced. Similarly, we would not be able to use randomization to learn about the impact of a specific historical or natural event, such as a government change or an earthquake (and constructing a credible counterfactual for such policies or events would be quite difficult, see also below on other evaluation methods).
At a smaller scale, an intervention may still affect many people at once, or there may be spillover effects from the intervention on third parties. Spillover effects mean that the treatment does not only have a direct impact on those who receive it, but also indirect impacts on other people. For example, in a close-knit community, cash grants or loans to some households often eventually reach other community members as well, in the form of gifts or loans from the original household. These effects can occur in many areas of social policy, from the environment (e.g., lower asthma rates for all households when some heat with cleaner fuels) to the labor market (e.g., more intense competition for jobs when some people have better training) to food subsidies for the poor (e.g., households taking in other family members).
Spillovers can make it difficult to conduct an impact evaluation, because the treatment may also indirectly affect the comparison group. In many cases, a randomized evaluation with a good research design can address this problem, and the solution typically involves increasing the level of randomization: for example, we randomize the intervention not at the household level, but at the community level (see below). However, sometimes controlling these issues would make a randomized study too difficult or too costly. Note that this challenge is shared by all forms of impact evaluation; if the comparison group is “contaminated” by the intervention group, we cannot obtain an unbiased measure of impact.
Randomization is not possible
Some things can simply not be randomly assigned. For example, we cannot carry out a randomized evaluation of the impact of gender on education outcomes: it is not possible to use randomization to learn how an otherwise “identical” person would have fared in school if they were a different gender. Many argue in fact that even the hypothetical construct of such a counterfactual is meaningless: what does it mean for a person to be “the same” except that their gender is different? (Angrist & Pischke, 2009). Again, this challenge is not specific to using randomization for estimating impact, in fact, Angrist and Pischke make this point for any kind of causal estimate.
It should be noted that this does not prevent us from studying discrimination using randomized evaluations: J-PAL affiliates have for example conducted studies that create hypothetical resumes where just the name of the person is changed to hint at their gender or ethnic background, and then counted employer response rates and interview invitation.6 7 Moreover, randomized evaluations can of course be used to study the impact of policies that aim to empower women. J-PAL’s gender sector covers these topics.
Ethical concerns
Ethical principles of research dictate that a research study with human participants should be designed to maximize the benefit from research and minimize the risks for the participants. Researchers should also carefully consider any risks from the research to non-participants, for example, staff or community members. A detailed resource on the ethical conduct of randomized evaluations can be found in Ethical conduct of randomized evaluations.
In some cases, ethical concerns about providing the intervention to a treatment group or withholding it from a comparison group prevent a randomized evaluation. For example, there is broad agreement that a randomized evaluation should not be used to test the effects of an entitlement that all members of the target population have the right to receive. In this case, it would be unethical to create a comparison group that is prevented from receiving the program, even if additional insights could be gained from conducting a randomized evaluation. By the same token, researchers cannot test interventions that are highly likely to cause harm.
Design modification may help to address these concerns. It is possible to conduct a randomized evaluation without restricting access to the intervention; for example, as described above, we could randomly select people to receive encouragement to enroll in a program - such as reminder emails or phone calls - without denying any interested participants access. In other cases, it can be useful to compare two different versions of an intervention, such as an existing version and a version with a new component added.
J-PAL affiliated researchers and staff weigh ethical factors carefully when deciding about conducting a study, and every evaluation funded or carried out by J-PAL is reviewed by a formal Institutional Review Board (IRB) whose mission is to protect the rights and welfare of study participants.
Other methods for impact evaluation
All types of quantitative impact evaluation estimate program effectiveness by comparing outcomes of those (individuals, communities, schools, etc.) who participated in the program against those who did not participate. The main difference between the various methods is how the intervention and comparison groups are chosen. For example, they might be the same group of participants, but at different points in time (pre-post comparison), or individuals who are just above or below some eligibility cutoff for a given program (regression discontinuity design).
A key challenge with non-randomized studies is to rule out systematic differences between participants and non-participants–the selection problem described earlier. Each non-experimental method makes a set of assumptions that help establish comparability of treatment and comparison groups. When these assumptions hold, the result of the evaluation is unbiased. It is important to verify that the assumptions are satisfied in each individual case.
A table taken from our case studies (embedded below) lists common methods of evaluation and the assumptions needed for them. Randomization is an effective tool to rule out selection bias, but non-randomized studies can be invaluable in contexts where randomized evaluations are not feasible (see above).
FAQs
More information about J-PAL’s approach can be found on the About Us page of our website, while our protocols for ensuring ethical research are discussed in greater detail in our resource on ethical conduct of randomized evaluations. In addition J-PAL North America’s guide to Common questions and concerns about randomized evaluations discusses frequently asked questions about randomized impact evaluations, also described below.
What are J-PAL’s protocols for ensuring ethical research?
J-PAL works to reduce poverty by ensuring that policy is informed by scientific evidence, and we hope that the research conducted at J-PAL ultimately serves the communities who participate in it. Ethical conduct of this research is therefore core to J-PAL’s mission, and it is of utmost importance that every study is done ethically from start to finish. Practical guidance on ethical considerations when designing and implementing a research project is discussed in greater detail in our Ethics resource.
J-PAL applies this guidance to ensure ethical conduct of studies we implement and fund through a number of proactive measures enacted across all of our offices. This begins with, but is not limited to, the requirements specified in our Research Protocols, which must be followed by every project funded or implemented by J-PAL. Projects carried out by J-PAL offices are regularly audited for adherence to these protocols. Additional measures include ethics training for research staff, in-depth informed consent training and confidentiality agreements for surveyors, strict data security requirements to protect participants’ private data at every stage of the project lifecycle, and collaboration with local institutions to set up formal ethics review boards or IRBs. More details on these measures can be found under the section "Ethics and research at J-PAL" in our Ethics resource.
Is it ethical to assign people to a control group, potentially denying them access to a valuable intervention?
As discussed above in the section "Real world challenges to randomization," there are cases when it is not appropriate to do an RCT. If there is rigorous evidence that an intervention is effective and sufficient resources are available to serve everyone, it would be unethical to deny some people access to the program. However, in many cases we do not know whether an intervention is effective (it is possible that it could be doing harm), or if there are enough resources to serve everyone. When these conditions exist, a randomized evaluation is not only ethical, but capable of generating evidence to inform the scale-up of effective interventions, or shift resources away from ineffective interventions.
When a program is first being rolled out, or is oversubscribed, financial and logistical constraints may prevent an organization from serving everyone. In such a case, randomization may be a fairer way of choosing who will have access to the program than other selection methods (e.g. first-come, first-served). Conducting a randomized evaluation may change the selection process, but not the number of participants served.
It is also possible to conduct a randomized evaluation without denying access to the intervention. For example, we could randomly select people to receive encouragement to enroll without denying any interested participants access to the intervention. In other cases, it may be useful to compare two different versions of an intervention, such as an existing version and a version with a new component added.
Is it possible to conduct randomized evaluations at low-cost without having to wait years for the results?
Collecting original survey data is often the most expensive part of an evaluation, but it is not unique to randomized evaluations. Likewise, it is increasingly possible to conduct evaluations at relatively low-cost by conducting surveys remotely or measuring outcomes using existing administrative data, instead of collecting survey data.
The length of time required to measure the impact of an intervention largely depends on the outcomes of interest. For example, long-term outcomes for an educational intervention (e.g. earnings and employment) require a lengthier study than shorter-term outcomes, such as test scores, which can be obtained from administrative records.
Finally, the time and expense of conducting a randomized evaluation should be balanced against the value of the evidence produced and the long-term costs of continuing to implement an intervention without understanding its effectiveness.
Can a randomized evaluation tell us not just whether an intervention worked, but also how and why?
When designed and implemented correctly, randomized evaluations can not only tell us whether an intervention was effective, but also answer a number of other policy-relevant questions. For example, a randomized evaluation can test different versions of an intervention to help determine which components are necessary for it to be effective, provide information on intermediate outcomes in order to test an intervention’s theory of change, and compare the effect of an intervention on different subgroups.
However, as with any single study, a randomized evaluation is just one piece in a larger puzzle. By combining the results of one or more randomized evaluations with economic theory, descriptive evidence, and local knowledge, we can gain a richer understanding of an intervention’s impact.
Are the results of randomized evaluations generalizable to other contexts?
The problem of generalizability is common to any impact evaluation that tests a specific intervention in a specific context. Properly designed and implemented randomized evaluations have the distinct advantage over other impact evaluation methods of ensuring that the estimate of an intervention’s impact in its original context is unbiased.
Further, it is possible to design randomized evaluations to address generalizability. Randomized evaluations may test an intervention across different contexts, or test the replication of an evidence-based intervention in a new context. Combining a theory of change that describes the conditions necessary for an intervention to be successful with local knowledge of the conditions in each new context can also inform the replicability of an intervention and the development of more generalized policy lessons.
Last updated April 2023.
These resources are a collaborative effort. If you notice a bug or have a suggestion for additional content, please fill out this form.
We thank Rohit Naimpally for helpful comments and Evan Williams for copy-editing this resource. All errors are our own.
Banerjee, Abhijit, Rema Hanna, Jordan Kyle, Benjamin A. Olken, and Sudarno Sumarto, 2018. “Tangible Information and Citizen Empowerment: Identification Cards and Food Subsidy Programs in Indonesia.” Journal of Political Economy 126 (2): 451-491.
Similar considerations arise with many programs that attempt to supplement, substitute, or fortify a household’s normal food and drink, from iron and iodine fortification to water chlorination: habits and tastes are powerful and difficult to change.
See Bougen et al.’s (2018) Using RCTs to Estimate Long-Run Impacts in Development Economics for a discussion of which types of randomized evaluations are best suited for measuring long-run effects.
The TUP program, pioneered by BRAC in Bangladesh, is a multifaceted approach to reducing poverty through which households are offered a productive asset (typically livestock), weekly food allowances, and training on how to increase the productivity of the asset. See Banerjee et al. (2020) for more information.
Behaghel, Luc, Bruno Crepon, and Thomas Le Barbanchon. 2014. “Unintended Effects of Anonymous Resumes”. IZA Discussion Paper Series no. 8517.
Bertrand, Marianne, and Sendhil Mullainathan. 2004. “Are Emily and Greg More Employable than Lakisha and Jamal? A Field Experiment on Labor Market Discrimination”
Additional Resources
J-PAL’s Table of Estimation Methods
J-PAL North America’s Real World Challenges to Randomization
References
Angrist, Joshua and Pischke, Jorn-Steffen. 2009. Mostly Harmless Econometrics: An Empiricist's Companion. 1 ed., Princeton University Press
Banerjee, Abhijit, Rema Hanna, Jordan Kyle, Benjamin A. Olken, and Sudarno Sumarto. 2018. “Tangible Information and Citizen Empowerment: Identification Cards and Food Subsidy Programs in Indonesia.” Journal of Political Economy 126 (2): 451-491. https://doi.org/10.1086/696226
Banerjee, Abhijit, Esther Duflo, and Garima Sharima. 2020. “Long-term Effects of the Targeting the Ultra Poor Program.” NBER Working Paper 28074. DOI 10.3386/w28074
Bates, Mary Ann and Glennerster, Rachel, 2017. “The Generalizability Puzzle”. Stanford Social Innovation Review, https://ssir.org/articles/entry/the_generalizability_puzzle
Behagel, Luc, Bruno Crépon, and Thomas Le Barbanchon. 2014. "Unintended Effects of Anonymous Resumes" IZA Discussion Paper Series No. 8517
Behrman, James. 2007. "Policy-Oriented Research Impact Assessment (PORIA) Case Study on the International Food Policy Research Institute (IFPRI) and the Mexican PROGRESA Anti-Poverty and Human Resource Investment Conditional Cash Transfer Program" International Food Policy Research Institute.
Bertrand, Marianne and Sendhil Mullainathan. 2004. "Are Emily And Greg More Employable Than Lakisha And Jamal? A Field Experiment On Labor Market Discrimination." American Economic Review 94(4): 991-1013. https://doi.or/10.1257/0002828042002561
Chetty, Raj, Nathaniel Hendren, and Lawrence F. Katz. 2016. "The Effects of Exposure to Better Neighborhoods on Children: New Evidence from the Moving to Opportunity Experiment." American Economic Review, 106 (4): 855-902. DOI: 10.1257/aer.20150572
Cunha, Jesse M., Giacomo De Giorgi, and Seema Jayachandran. 2019. “The Price Effects of Cash Versus In-Kind Transfers”. The Review of Economic Studies, 86(1):240–281. https://doi.org/10.1093/restud/rdy018
Gelber, Alexander, Adam Isen and Judd B. Kessler. 2016. "The Effects of Youth Employment - Evidence from New York City Summer Youth Employment Program Lotteries." Quarterly Journal of Economics 131 (1): 423-460. https://doi.org/10.1093/qje/qjv034
Duflo, Esther, Pascaline Dupas, and Michael Kremer. 2011. “Peer Effects, Teacher Incentives, and the Impact of Tracking: Evidence from a Randomized Evaluation in Kenya.” American Economic Review 101 (5): 1739-74.
Duflo, Esther, Pascaline Dupas, and Michael Kremer. 2014. “School Governance, Teacher Incentives, and Pupil-teacher Ratios: Experimental Evidence from Kenyan Primary Schools.” Journal of Public Economics 123 (15): 92-110.
Finkelstein, Amy, Yunan Ji, and Neale Mahony. 2018. “Mandatory Medicare Bundled Payment Program for Lower Extremity Joint Replacement and Discharge to Institutional Postacute Care: Interim Analysis of the First Year of a 5-Year Randomized Trial” JAMA, 320(9): 892-900 doi:10.1001/jama.2018.12346
Gertler, Paul J and Simone Boyce. 2003. "An Experiment in Incentive-Based Welfare: The Impact of PROGRESA on Health in Mexico," Royal Economic Society Annual Conference 2003 85, Royal Economic Society. RePEc:ecj:ac2003:85
Heller, Sara. 2014. "Summer Jobs Reduce Violence among Disadvantaged Youth" Science Magazie 346(5): 1219-1223. http://dx.doi.org/10.1126/science.1257809
J-PAL. 2019. “Case Study: Extra Teacher Program: How to Randomize.” Abdul Latif Jameel Poverty Action Lab. 2019. Cambridge, MA.
J-PAL. “Case Study: Cognitive Behavioral Therapy in Chicago Schools: Theory of Change and Measuring Outcomes.” Abdul Latif Jameel Poverty Action Lab. 2019. Cambridge, MA.
J-PAL. “Case Study: Get Out the Vote: Why Randomize?” Abdul Latif Jameel Poverty Action Lab. 2019. Cambridge, MA.
J-PAL. “Deworming in Kenya: Threats and Analysis.” Abdul Latif Jameel Poverty Action Lab. 2019. Cambridge, MA
J-PAL. The Generalizability Puzzle. Delivered in J-PAL Global & North America’s 2019 Evaluating Social Programs course.
Katz, Lawrence, F., Jeffrey R. Kling, and Jeffrey B. Liebman. 2001. “Moving to Opportunity in Boston: Early Results of a Randomized Mobility Experiment.” The Quarterly Journal of Economics 116(2)607–654. https://doi.org/10.1162/00335530151144113
Lamanna, Francesca. 2014. "A Model from Mexico for the World." The World Bank.
Sacarny, Adam, David Yokum, Amy Finkelstein, and Shantanu Agrawal. 2016. “Medicare Letters to Curb Overprescribing of Controlled Substances Had No Detectable Effect on Providers”. Health Affairs, 35(3). https://doi.org/10.1377/hlthaff.2015.102